Host-microbiota interactions in the infant gut revealed by daily faecal sample time series
Abstract
Aim: This study aims to explore the interplay between host immune factors and gut microbiota in human infants in vivo using time-series daily stool samples and identify biomarkers of host-microbe interactions.
Methods: 216 faecal samples collected from infants aged 5-6 or 11-12 months were analysed for gut microbiota composition, total bacterial load, and biomarkers of immune function.
Results: We identified indications of microbial stimulation of eosinophil cationic protein (ECP), IgA, calprotectin (Cal), intestinal alkaline phosphatase (IAP), and Bactericidal/permeability-increasing protein (BPI) at 6 and 12 months, as well as stimulation of lipocalin 2 (LCN2), lactoferrin (LTF), and alpha-defensin-5 only at 6 months. The associations between biomarker concentrations and bacterial population growth were primarily positive at 6 months and mostly negative at 12 months, suggesting increasing host regulation of the microbiota with age. The exceptions were IAP, which was predictive of declining bacterial populations at both time points, and Cal, whose associations changed from negative at 6 months to positive at 12 months.
Conclusion: There is an age-associated development in the correlation pattern between bacterial population growth and the biomarker concentrations, suggesting that host-microbe interactions change during early development. Albumin appeared as a potential marker of gut permeability, while LCN2 seemed to correlate with gut transit time. Mucin degradation appeared to decrease with age. Mucin2 and IAP emerged as potentially important regulators of the bacterial populations in the infant gut. The study demonstrates the utility of biomarker and bacteria profiling from daily stool samples for analysing in vivo associations between the immune system and the gut microbiota and provides evidence of host regulation of the microbiota in infants.
Keywords
INTRODUCTION
The human body is a bustling metropolis of microorganisms collectively known as the microbiota. The microbiota includes fungi, bacteria, archaea, and bacteriophages, with bacteria forming the most abundant taxonomic group. In the human gut, the main bacterial species are members of the phyla Firmicutes, Bacteroidetes, Proteobacteria, Actinobacteria, and Verrucomicrobia[1].
The microbiota of an individual begins to form at birth, with significant colonisation happening at the moment of birth. During vaginal birth, the infant receives microbes from the mother, which lays the groundwork for microbiota development[2]. After the initial seeding, the composition of the gut microbiome is influenced by everything the infant is exposed to, mainly what they consume, including solid foods[3,4].
The gut microbiome is involved in many vital facets of life, such as digestion and regulation of metabolism and the immune system[5]. Dysregulation of the microbiota is associated with a wide range of diseases, both during infancy and later in life, including metabolic diseases, allergic and chronic inflammatory diseases[5,6].
Infants are born with an immature immune system[7], making them vulnerable to infectious diseases within the first months of life. The infant gut microbiota can prevent pathogen colonisation and help train the immune system. Importantly, the infant gut microbiota impacts the later health of the host by affecting the development of the host’s physiology. Aberrations in the infant gut microbiota development are linked to the later onset of immune disorders such as atopic dermatitis (eczema), inflammatory bowel disease (IBD), and asthma, as well as obesity[5,8]. Because factors influencing the infant’s gut microbiome also impact the infant’s future health, ensuring a healthy infant microbiome, regardless of external factors such as birth method and feeding style, can be a cost-effective and promising approach to preventing later health issues.
To regulate the infant microbiome for health purposes, understanding the determinants of microbiota composition is essential. Most of the interindividual variation in gut microbiota composition in infants is still unexplained - studies have shown that approximately 20%-30% of the variation can be explained by external factors, such as birth mode, diet, and antibiotic exposure[9,10]. It is assumed that host-specific factors are important in regulating the gut microbiota, but these are poorly understood. The host influences the microbiome through dietary exposures and genetic factors. Quantitative trait loci (QTLs) that affect the microbiome are immune- or metabolism-related. Notably, the ABO (A, B antigens) and LCT locus (lactase) explain a small percentage of microbiota variance consistently across multiple GWAS[11]. How the gut microbiota interacts with the infant’s immature immune system and how the immune system, in turn, affects the abundance and composition of the microbiota have not been extensively studied.
Most human microbiota studies rely on cross-sectional data on the relative abundances of microbes. However, microbial populations undergo fluctuations in size, which may induce noise into cross-sectional data sets. Furthermore, compositional data suffer from the problem that the relative abundances of the different microbes are not independent, and thus, a change in one microbe will cause artefactual changes in other microbes[12,13]. True population growth or decline cannot be measured from relative abundance data. Analysing absolute abundances can overcome the problem of compositionality[12,13].
Studies aimed at elucidating the link between immune markers and microbiota are often limited to a few markers or microbes and are performed in mice or in vitro or linked to a specific disease. As a result, we lack data on host-microbe interactions in healthy infants. This exploratory study aims to provide insight into the host factors influencing the microbiota and vice versa. We analysed stool samples from 6- and 12-month-old infants for 30 days. Stool biomarkers are reliable and non-invasive indicators of intestinal and, in some cases, general health[14]. We combined absolute abundances of bacteria based on metagenomic sequencing and qPCR with immune-related biomarkers: intestinal alkaline phosphatase (IAP) and bactericidal/permeability-increasing protein (BPI) as markers of host reaction to bacterial lipopolysaccharide that could inhibit bacterial growth[15,16], human alpha defensin 5 (HD-5) as a marker of Paneth cell response[17], eosinophil cationic protein (ECP) as a marker of eosinophil response[18], lipocalin 2 (LCN2), lactoferrin (LTF), and calprotectin (Cal) as markers of inflammation and neutrophil response[19,20], immunoglobulin A (IgA) as a general regulator of microbiota homeostasis in the gut[15,21], mucin 2 (Muc2) as an indicator of mucus production[22], and albumin as a potential indicator of gut epithelial integrity[23]. In addition, we measured faecal pH, total bacterial load using qPCR, and assessed the Bristol score. The daily samples enabled correlative analysis of the daily changes of both biomarkers and microbiota, enabling the identification of potential microbe-induced expression of the biomarkers and biomarker-induced regulation of the microbiota.
METHODS
Samples collection
Faecal samples of infants were collected as part of the Helmi Plus study in 2017-2018 as part of the HELMi cohort[24]. HELMi cohort consists of 1,055 healthy term infants born in 2016-2018, mainly in the capital region of Finland, and their parents. The intestinal microbiota development of the infants is characterised based on nine strategically selected faecal samples and connected to extensive online questionnaire-collected metadata at weekly to monthly intervals focusing on the diet, other exposures, and family’s lifestyle, as well as the health and growth of the child. A subset of the HELMi families participated in HelmiPlus, where the caretakers collected daily samples for 20-30 days when the infants were 5-6 months old (during the first introduction of solid foods) and/or 11-12 months old (when the infants were mostly consuming solid foods). Samples were stored in the home freezer at -20 °C until transported frozen to the lab and stored at
Median (interquartile range) biomarker concentrations categorised by birth mode and age
5-6 months | 11-12 months | Total | ||||
Vaginal | C-section | Vaginal | C-section | Vaginal | C-section | |
Number of samples | 54 | 60 | 48 | 54 | 102 | 114 |
IAP mg/g | 25.97 (6.20-58.85) | 24.18 (16.32-32.73) | 14.09 (8.28-23.19) | 8.85 (3.14-16.07) | 14.4 (3.9-32.7) | 20.6 (10.1-29.7) |
BPI ng/g | 0.00 (0.00-0.00) | 0.00 (0.00-0.00) | 25.48595 (1.67-42.25) | 0.00 (0.00-10.45) | 0.00 (0.00-27.3) | 0.00 (0.00-7.0) |
HD5 pg/g | 0.00 (0.00-142.21) | 37.50 (0.00-230.32) | 0.00 (0.00-34.46) | 102.21 (52.49-160.04) | 0.00 (0.00-73.2) | 96.0 (0.00-184) |
ECP pg/g | 183.97 (0.00-1,037.84) | 198.38 (0.00-558.94) | 155.03 (0.00-331.03) | 662.31 (488.187-772.91) | 183 (0.00-684) | 458 (173-672) |
IgA μg/g | 238.47 (54.26-609.15) | 328.80 (227.96-452.58) | 253.88 (123.26-1,031.91) | 307.57 (208.23-497.60) | 243 (80.6-905) | 309 (208-491) |
LTF μg/g | 6.91 (2.53-12.25) | 10.42 (6.49-16.08) | 0.91 (0.34-2.69) | 3.96 (1.90-6.68) | 2.75 (0.89-9.11) | 6.75 (3.75-12.40) |
Alb ng/g | 2.32 (0.33-20.63) | 5.575 (2.74-25.19) | 58.07 (7.31-198.96) | 9.51 (6.39-17.34) | 12.3 (1.91-72.1) | 8.89 (4.50-22.6) |
LCN2 ng/g | 1.16 (0-5.47) | 1.77 (0.00-17.51) | 0.01 (0.00-22.70) | 0.00 (0.00-1.24) | 0.83 (0.00-8.95) | 0.00 (0.00-6.55) |
Cal ng/g | 354.20 (88.01-1,602.02) | 1,857.89 (1,199.08-2,707.68) | 167.22 (0.00-465.38) | 669.41 (266.36-1,958.44) | 231 (21.0-842) | 1,341 (601-2,542) |
Tot.prot. mg/g | 3.76 (0.31-13.79) | 6.95 (0.00-17.16) | 5.34 (0.87-8.37) | 15.35 (11.40-18.67) | 5.17 (0.46-11.8) | 13.1 (6.16-17.6) |
Muc2 ng/g | 0.71 (0.30-2.42) | 45.04 (22.53-75.84) | 1.96 (0.20-7.41) | 3.87 (1.75-7.99) | 0.98 (0.24-5.81) | 14.9 (4.00-51.5) |
Lysozyme ng/g | 0 (0-2.05) | 0.00 (0.00-0.00) | 0.00 (0.00-0.00) | 0.9780727 (0-2.07) | 0 (0-1.13) | 0 (0-1.74) |
Zonulin ng/g | 0.0 (0.0-0.34) | 0.7 (0.0-1.30) | 1.5 (0.8-1.80) | 0 (0-0) | 0.7 (0-1.5) | 0.0 (0-0.7) |
Bristol | 6 (6-6.00) | 6 (6-6.00) | 5 (5-5.00) | 5 (5-5.75) | 6.00 (5.00-6.00) | 6.00 (5.00-6.00) |
pH | 6.065 (5.70-6.90) | 7.240 (6.98-7.61) | 7.220 (7.07-7.42) | 6.600 (6.36-6.93) | 6.95 (5.91-7.27) | 6.97 (6.57-7.29) |
Total bacteria (log 10) genomes/g | 25.19 (23.98, 29.93) | 28.02 (26.43, 29.93) | 27.82 (24.75, 28.89) | 25.24 (24.47, 26.80) | 26.50 (24.40-29.41) | 26.63 (25.45-28.40) |
DNA extraction and qPCR
Bacterial DNA was extracted from faecal samples using a modified version of repeated bead beating[25]. Briefly, the faecal DNA was extracted from 250 to 340 mg of faecal material that was suspended in 0.5 mL of sterile ice-cold phosphate-buffered saline (PBS), and 250 μL of the faecal suspension was combined with
Quantification of total bacteria was carried out by qPCR using a BioRad iCycler iQ thermal cycler system (BioRad, Hercules, CA) with HOT FIREPol® EvaGreen® qPCR Mix Plus (Solis BioDyne, Tartu, Estonia) as explained[12], 331F (TCCTACGGGAGGCAGCAGT)/797R (GGACTACCAGGGTATCTAATCCTGTT) primers targeting the 16S rRNA gene and 0.5 ng of faecal DNA. Briefly, the thermal cycling conditions started with a DNA-denaturation step at 95 °C for 15 min, followed by 40 cycles of (1) denaturation at 95 °C for 15 s; (2) annealing at a primer-specific temperature for 20 s; (3) extension at 72 °C for 30 s; and (4) an incubation step to detect the fluorescent data. A melting curve analysis was carried out to ensure the specificity of the amplification products. The 10-log-fold standard curves ranging from 102 to 107 copies were produced using the full-length amplicons of the 16S rRNA gene of Bifidobacterium longum to convert the threshold cycle (Ct) values into the average estimates of genomes present in 1 g of faeces (copy numbers/g of wet faeces) in each assay[26].
Metagenomic sequencing
Sequencing libraries were prepared using the Illumina Nextera DNA Flex kit, according to the manufacturer’s instructions. Shotgun metagenomic sequencing of 2 × 150 bp was performed with an Illumina NovaSeq system using S4 flow cells with a lane divider (Illumina, San Diego, CA, United States) at the sequencing laboratory of the Institute for Molecular Medicine Finland (FIMM), University of Helsinki.
Taxonomic annotation
Raw reads were filtered using fastp[27], with parameters Q < 20, min read length > 50, reads merged with a minimum of 15 bp overlap, reads with Ns discarded, and 3 bp trimmed from the front and back of the reads. To remove host DNA, filtered reads were mapped using Minimap2 (Li, 2021) and SAMtools[28] against the human genome (GRCh38.p14, NCBI RefSeq assembly: GCF_000001405.40). Taxonomic annotation was performed by mapping the filtered reads using Mininimap2 against the HumGutDB[29]. Relative abundances were summarized at different taxonomic levels in R and translated into absolute abundances by multiplying with the total number of bacterial genomes.
Bristol score
Bristol score was determined visually from faecal samples upon retrieval from -20 °C storage.
Faecal water extraction from faecal samples
Approximately 100 mg aliquots of the frozen faecal samples were taken and suspended into 0.5 mM solution of PMSF protease inhibitor (Thermo Scientific, 36978) in 1× PBS (VingLab) in a 1:10 ratio. The median pH was 6.97 (interquartile range 6.47-7.28). Samples were kept on ice. Samples were centrifuged twice for 15 min, at +4 °C at 13,000 rpm. Sample supernatants were distributed to 96-well plates for storage at -80 °C and further analysis.
Biomarker quantification
All absorbances were read with Hidex Sense microplate reader.
Total protein levels
Total protein concentrations were measured using a DC Protein Assay Reagents Package (5000116, Bio-Rad) according to the manufacturer’s protocol, with bovine serum albumin lyophilized (Biowest, P6154) as standard. A linear standard curve was used for the calculation of the results.
Albumin - Alb
Albumin was quantified with sandwich ELISA using Human Albumin Matched Antibody Pair Kit (Abcam, ab246841) according to the manufacturer’s general protocol for matching antibody pair kits. A four-parameter logistic curve was used for the calculation of the results.
IAP
IAP activity was quantified with QUANTI-Blue solution (rep-qbs, InvivoGen) according to the manufacturer’s instructions. A standard test was performed to identify the correct standard concentrations. Secreted embryonic alkaline phosphatase (SEAP) protein (rec-hseap, Invivogen) was used as the standard. A linear standard curve was used for the calculation of the results.
BPI
BPI was quantified by sandwich ELISA using BPI, Human, ELISA kit (HK314, Hycult Biotech) according to the manufacturer’s instructions, except samples were diluted 1:2 in dilution buffer. A four-parameter logistic curve was used for the calculation of the results.
HD5
Alpha defensin five was quantified with sandwich ELISA using HD5 (Paneth Cell Specific) ELISA Kit (E-EL-H1798, Elabscience) according to the manufacturer’s instructions. Samples were diluted 1:2 in reference standard and sample diluent. A four-parameter logistic curve was used for the calculation of the results.
ECP
ECP was quantified with sandwich ELISA using Human RNASE3/ECP (Ribonuclease A3/Eosinophil Cationic Protein) ELISA Kit (E-EL-H1379, Elabscience) according to the manufacturer’s instructions. Samples were diluted 1:2 in reference standard and sample diluent. A four-parameter logistic curve was used for the calculation of the results.
Muc2
Muc2 was quantified by sandwich ELISA using Human Muc2 ELISA Kit (E-EL-H0632, Elabscience) according to the manufacturer’s protocol. Samples were diluted 1:4 in reference standard and sample diluent. A four-parameter logistic curve was used for the calculation of the results.
IgA
IgA was quantified by sandwich ELISA using Human IgA Matched Antibody Pair Kit (Abcam, ab219536) according to the manufacturer’s general protocol for matched antibody pair kits. A linear standard curve was used for the calculation of the results.
LTF
LTF was quantified with sandwich ELISA using Human Lactotransferrin / LTF ELISA Pair Set (SEK11096, SinoBiological) according to the manufacturer’s instructions. A linear standard curve was used for the calculation of the results.
LCN2
LCN2 was quantified with sandwich ELISA using Human Lipocalin-2/NGAL Matched ELISA Antibody Pair Set (SEK10222, SinoBiological) according to the manufacturer’s instructions, using 100 μL of STOP solution per sample. A linear standard curve was used for the calculation of the results.
Cal
Cal was quantified by sandwich ELISA using Human Cal (S100A8 + S100A9) Antibody Pair - BSA and Azide free (Abcam, ab309558) and Recombinant Human Cal (S100A8 + S100A9) protein (Abcam, ab130945) for the standards. The detector antibody was biotinylated using Biotinylation Kit / Biotin Conjugation Kit (Fast, Type A) - Lightning-Link® (Abcam, ab201795) according to the manufacturer’s instructions. The ELISA was performed according to the general matched antibody pair kit protocol in Human IgA Matched Antibody Pair Kit (Abcam, ab219536). A linear standard curve was used for the calculation of the results.
Lysozyme
Lysozyme was quantified by sandwich ELISA using Human Human LZM (Lysozyme) ELISA Kit (Elabscience, E-EL-H1869). A four-parameter logistic curve was used for the calculation of the results.
Zonulin
Zonulin was quantified by sandwich ELISA using Human Zonulin ELISA Kit (elabscience, E-EL-H5560) according to the manufacturer’s instructions. A four-parameter logistic curve was used for the calculation of the results.
Statistical analysis
All analyses were performed in R 4.3.1 (2023-06-16) within Rstudio Version 2023.06.2+561 for macOS.
We used R the packages mare[30], reshape2[31], nlme[32], and gplots[33]. Both microbial absolute abundances and biomarker levels were analysed after log transformation to obtain normal distributions. We calculated the daily changes in both by subtracting the log-transformed abundance on day t from the log-transformed abundance on day t + 1. Bacteria were analysed at the family and genus levels, including only taxa that were present at > 0.1% in at least 50% of at least one of the time series (48 genera and 23 families). We used linear mixed models (function lme) to identify associations between microbial taxa and biomarkers, with the time series ID, combining information on subject ID and age, as a random factor. The model residuals were random and normally distributed without temporal patterns. The models were adjusted for total protein concentration/change and total bacteria change in the sample.
When modelling the associations between biomarker concentrations and bacterial changes, the biomarker concentrations were normalised by scaling and centring by time series to remove average-level differences between individuals. Due to the exploratory nature of the study, we chose to define statistical significance as P < 0.05 without multiple testing adjustment, as it was considered important to find all true associations (reduce the number of false negatives) even if some false positives may arise. To reduce the number of false positives, we did not analyse the data at the species level, as we expect related species to have similar biomarker associations.
RESULTS
We investigated associations between faecal microbiota and immune-related biomarkers in daily time series of 6 infants sampled at the age of 5-6 and/or 11-12 months. The infants were selected from a larger cohort for this exploratory study based on diverse microbiota compositions, including Bifidobacterium-dominated, Bacteroides-dominated, Enterobacteriaceae-dominated, and Clostridia-dominated communities to maximize the generality of the results despite the small sample size. All infants were breastfed at 5-6 months and ¾ infants at 11-12 months.
Biomarker concentrations
The average immune-related biomarker concentrations fluctuated during each time series, but typically did not show strong directional change. They did not differ by birth mode or infant age, apart from Cal, which was higher in the caesarean-born infants (P = 0.02, linear mixed model, Table 1), and Bristol score, which was higher at 6 months (P < 0.001).
Associations between faecal biomarkers
As a first exploratory step, we assessed the raw correlations between faecal biomarkers normalised within each time series without adjusting for repeated sampling. At both 6 and 12 months, total bacteria load was positively associated with pH and total protein [Figure 1A and B]. The concentrations of most measured biomarkers - IAP, LTF, Cal, Muc2, HD5, lysozyme, and ECP - were positively correlated with each other and with total protein and total bacterial load [Figure 1A]. BPI was positively correlated with albumin and negatively correlated with lysozyme and Muc2 [Figure 1A]. At 12 months, total protein was still significantly correlated with IAP, LTF, HD5, IgA, and ECP and with Cal, but not with Cal [Figure 1B]. Cal correlated only with zonulin and vice versa [Figure 1B]. Total bacteria were not significantly associated with any biomarker at 12 months [Figure 1B].
Figure 1. Intercorrelations between faecal biomarker concentrations, lower left triangle, and daily changes (delta), upper right triangle, (A) at 6 months and (B) at 12 months. P-values are indicated as asterisks: P < 0.001***; P < 0.1**; P < 0.5*.
At both time points, LCN2 concentrations were inversely correlated with the protein and bacteria content of the stool and with pH, but only significantly at 6 months [Figure 1A and B]. Muc2 was negatively associated with pH at 6 months and with total bacteria at both time points [Figure 1A and B].
To obtain a more detailed understanding of the interrelated dynamics between the biomarkers, we analysed the associations between their daily changes [Figure 1A and B upper right triangle]. Increases in total bacterial load were associated with increasing pH and total protein content at both time points. Changes in HD5 were positively correlated with changes in ECP, BPI, and zonulin. So too were changes in albumin and IgA, and LTF, IAP, and Cal [Figure 1A and B].
Changes in LCN2 were negatively correlated with changes in IAP, total protein, and pH at 6 months. At 6 months, muc2 decreased when total bacteria or pH increased [Figure 1A]. These associations were still present but not significant at 12 months [Figure 1B].
Because bifidobacteria have been previously associated negatively with faecal pH in infants, we tested these associations specifically, and discovered a significant negative association at 6 months (P ≤ 0.001), but a positive one at 12 months (P ≤ 0.001).
Associations between bacterial population growth and faecal biomarker changes
General association patterns
We attempted to identify bacterial stimulation of immune biomarkers by predicting the daily change in biomarker concentrations with the daily changes in microbial absolute abundances. We identified both negative and positive associations between bacterial population growth and biomarker changes, potentially indicative of bacterial stimulation or inhibition of biomarker expression [Figure 2]. Overall, Collinsella emerged as the most consistent and the strongest potential inhibitor of many immune-related biomarkers, especially ECP. Bifidobacterium and Akkermansia stood out due to their weak and exclusively negative associations with the biomarkers [Figure 2]. Both had a negative association with HD5 and Cal at 6 months (although not significant for Bifidobacterium), and Bifidobacterium with albumin at 12 months.
Figure 2. Gut microbes predicting faecal biomarker changes after adjustment for total protein and total bacteria change. Associations between daily faecal biomarker changes and daily changes in gut microbe abundances at genus levels at (A) 6 months and (B) 12 months of age. The colour represents the strength of association from a linear mixed model adjusting for total protein and total bacterial abundance changes. P-values are indicated as asterisks: P < 0.001***; P < 0.1**; P < 0.5*. The microbial class is presented as the sidebar colour.
Indicators of gut homeostatic regulation
Levels of muc2 at 6 months increased with an increase in Haemophilus, Veillonella, Lachnospira, and Dorea, and decreased with increasing levels of Sellimonas and Collinsella [Figure 2A]. At 12 months, muc2 decreased with an increase in many bacterial genera, including members of Clostridia, but was positively associated with Lactobacillus and Streptococcus [Figure 2B]. Albumin levels declined with increasing Collinsella (both time points) and Bifidobacterium (12 months) and increased in association to Haemophilus and members of Negativicutes [Figure 2A and B]. IgA had mostly negative associations at 6 months, including with Anaerotruncus and Dialister, but mostly positive ones at 12 months, with Phascolarctobacterium statistically significant [Figure 2A and B].
IAP was positively correlated with Bacteroides at 6 months [Figure 2A], and Actinomyces, Streptococcus, and genera of the Enterobacteriaceae family at 12 months [Figure 2B]. At 12 months, IAP had negative associations with members of Clostridia, including Roseburia and Lachnospira [Figure 2B]. BPI was positively associated with members of Enterobacteriaceae at both time points and negatively with Lactobacillus at 6 months and members of Clostridia at 12 months [Figure 2].
Indicators of inflammation
Cal had only negative (Prevotella and Akkermansia) associations with bacteria at 6 months [Figure 2A], but mostly positive ones at 12 months, mainly with Prevotella and Pseudoruminococcus [Figure 2B]. LTF had negative associations with members of Clostridia at both time points [Figure 2A and B], and with members of Enterobacteriaceae at 6 months [Figure 2A].
Apart from a strong negative association between ECP and Collinsella, ECP appeared mostly stimulated by bacteria at 6 months, especially by Streptococcus and Clostridioides [Figure 2A], while it had mostly negative associations with increasing bacterial populations at 12 months, including Blautia, Lachnospira, and Haemophilus [Figure 2B].
Strong associations were observed for LCN2. At 6 months, increasing abundances of Streptococcaceae and Veillonellaceae were associated with increasing LCN2 levels, while members of the class Clostridia and Roseburia correlated negatively with LCN2 [Figure 2A]. However, these associations were not observable at 12 months [Figure 2B]. At 12 months, LCN2 increase was associated with declining populations of Bacilli and Enterobacteriaceae [Figure 2B].
Levels of HD5 were negatively associated with several bacterial taxa, including Dialister at both time points, Bifidobacteriaceae and Ruminococcaceae at 6 months [Figure 2A], and Prevotellaceae at 12 months [Figure 2B]. HD5 was positively associated with Megasphaera at 6 months [Figure 2A], and with Actinomyces at 12 months [Figure 2B].
Lysozyme levels were strongly negatively associated with Clostrida members at 6 months [Figure 2A]. Yet, at 12 months, lysozyme levels were mostly positively correlated with other Clostridia members, Megasphaera, Pseudoruminococcus, Parabacteroides, and Dorea but negatively with Collinsella and Bifidobacterium [Figure 2B].
Zonulin was not associated with any bacteria genera at 6 months, but at 12 months, was positively correlated with several, including Megasphaera, Eggerthella, and several Clostridia members [Figure 2A and B].
Associations between biomarker concentration and bacterial population growth
We hypothesized that associations between biomarker concentration on day t and the change in bacterial population size from day t to t + 1 (population growth) would represent bacterial responses to the biomarkers [Figure 3].
Figure 3. Biomarker levels predicting gut microbe changes. Associations between faecal biomarker concentrations and daily changes in gut microbe abundances at the family and genus levels at (A) 6 months and (B) 12 months of age. The colour represents the strength of association from a linear mixed model adjusting for total protein concentration. P-values are indicated as asterisks: P < 0.001***; P < 0.1**; P < 0.5*. The microbial class is presented as the sidebar colour.
Indicators of gut homeostasis
The association between muc2 and bacterial growth changed from mostly positive at 6 months [Figure 3A] to mostly negative at 12 months [Figure 3B]. The only exception at 12 months was Bifidobacterium, which appeared to be stimulated by muc2 [Figure 3B]. At 6 months, higher albumin levels were predictive of increasing Haemophilus and Lacticaseibacillus [Figure 3A], but no associations were observed at 12 months. IgA at 6 months was predictive of increasing Veillonella and Citrobacter [Figure 3A], while at 12 months, Lacticaseibacillus declined in association with high IgA levels [Figure 3B].
High IAP levels were generally associated with bacterial declines, especially among Clostridia. BPI was negatively associated with the growth of Bilophila, Lachnospira, and members of Negativicutes at 12 months [Figure 3B], but not at 6 months.
Indicators of inflammation
Cal was mostly negatively associated at 6 months, notably with Akkermansia, Klebsiella, and Prevotella, and was only positively correlated with Erysipelatoclostridium [Figure 3A]. Cal was positively correlated with Lactobacillus and Pseudoruminococcus at 12 months [Figure 3B]. LTF and ECP concentrations had no strong associations with microbial changes, apart from a notable strong negative association between ECP and Akkermansia at 12 months. High levels of LCN2 were associated with increasing abundances of many Clostridia genera at 6 months [Figure 3A], but these were mostly non-significant at 12 months. High levels of HD5 were negatively associated with Akkermansia (significant only at 6 months) and Haemophilus (12 months), and positively with Lactobacillus, Enterococcus, and Enterocloster (12 months) [Figure 3A and B]. High lysozyme levels predicted a decline in Anaerostipes at 6 months. At 12 months, it correlated with increasing levels of Pseudoruminococcus and decreasing levels of Prevotella and Senegalimassilia. A high zonulin level was associated with increasing abundance of Blautia at 6 and 12 months[Figure 3A and B]. At 6 months, it was also associated positively with Bilophila and Flavonifractor [Figure 3A], and at 12 months with Clostridioides [Figure 3B].
DISCUSSION
Utilising densely sampled absolute abundance time series of infant gut microbiota and immune-related biomarkers, we were able to identify potential host-microbe interactions occurring in vivo in healthy infants. Although microbial programming of the immune system is considered important during early life, there is limited research on this topic in human infants. Most of the previous work on the subject has been done in vitro or in mice. Exploring approaches to investigate host-microbe interactions in humans in vivo is important, as in vitro studies often do not represent the whole human gut ecology[34] and mouse models suffer from questionable relevance and difficulties in interpretation and translation[35,36].
At 6 months, most immune biomarkers were intercorrelated and correlated to total protein, total bacterial content and pH, except BPI, albumin, and LCN2, which were associated with each other and inversely correlated with bacterial load and pH. The intercorrelated markers included LTF, IgA, and lysozyme, which, especially at 6 months, may be derived from breastmilk[37]. However, due to the strong associations with the non-breastmilk-associated biomarkers, such as ECP, DH5, and Cal[35], it is likely that the LTF and IgA in our samples are mostly infant-derived.
In infants, low pH has been associated with a high abundance of bifidobacteria[38,39], which we confirmed at 6 months when bifidobacteria are typically the dominant group and the most important taxon responsible for human milk oligosaccharide fermentation, but not at 12 months when the fermentation of other, non-HMO substrates will be more prevalent.
We identified indications of microbe-induced stimulation of muc2, ECP, IgA, albumin, Cal, HD5, IAP, and BPI at both time points, as well as stimulation of LCN2 and LTF only at 6 months. The associations between biomarker concentrations and bacterial population growth were mostly positive at 6 months and largely negative at 12 months, suggestive of increasing host regulation of the microbiota with age. The results indicate an effect of immune signalling in the gut shifting from tolerance toward more defensive, as the immune system does when maturing[7,40]. The exception was Cal, whose effects changed from negative at 6 months to positive at 12 months. Thus, the major host-derived regulators of microbial growth appeared to be Cal at 6 months, and IAP, BPI, and mucin at 12 months. We will briefly discuss the results per biomarker below.
Markers of gut homeostatic regulation
Muc2 is the most abundant mucin in the gut and, thus, an important mediator of host-microbe interactions[41]. We hypothesised that faecal muc2 may indicate the balance between mucus production and degradation and, thus, the condition of the gut mucus layer. Our results suggest that mucin degradation is more substantial at 6 months than at 12 months, as muc2 decreased with increasing bacterial load only at 6 months, and it appeared to stimulate microbial growth at 6 months but to reduce it at 12 months. Before infants consume substantial amounts of solid foods, breastmilk and mucin are the primary carbon sources for gut bacteria[41]. In vivo, the mucin, which forms the intestinal epithelial mucous membrane, would serve as a scaffold and source of nourishment for bacteria when the infant is not yet weaned[41]. Negative associations between muc2 and members of Clostridia were observed at both time points, suggesting that these organisms either reduce its secretion or participate in its degradation. The former is more likely since these populations did not appear to benefit from increasing muc2 levels.
At 12 months, muc2 emerged as a potential inhibitor of microbial growth. Only Bifidobacterium appeared to benefit from muc2 at 12 months. Mucin contains a similar oligosaccharide structure as breastmilk, and therefore, some of the breastmilk-adapted Bifidobacterium spp. can also degrade mucin[42]. Thus, Bifidobacterium spp. likely benefit from increasing muc2 levels, especially at 12 months, when the amount of breastmilk the infant receives is decreasing. We did not observe a significant association between muc2 and the mucin-degrading Akkermansia, possibly because Akkermansia grows in the mucus layer and its abundance may not depend on luminal mucin.
Albumin is the most abundant protein in blood, and thus, its presence in faecal samples has been suggested to be an indication of increased gut permeability[23]. Albumin increased in association with Gram-negative organisms, including Haemophilus, but lowered with Collinsella and Bifidobacterium. These results are in line with previous findings from preterm infants, where increased gut permeability measured by the lactulose-mannitol test was associated with opportunistic pathogens, such as Staphylococcus and enterobacteria, while permeability was low in infants dominated by bifidobacteria[43]. Albumin was correlated with IAP and BPI, suggesting a shared regulatory pattern or the possibility that increased permeability stimulates the secretion of antibacterial compounds. However, zonulin, a protein known to increase tight junction permeability and often used as a marker of gut permeability[44], did not correlate with albumin. In most infants, zonulin levels were below the detection level. Our results suggest that albumin may be a more sensitive marker of gut permeability in healthy infants than zonulin.
Secretory IgA is the most abundant antibody in the intestine and is considered the first line of defence against pathogens in the gut[21,45,46], but is also secreted into breastmilk. IgA is thought to help support favourable microbiota[21,46]. We found that changes in IgA correlated with changes in IAP, lysozyme, and albumin. Like albumin, IgA was positively associated with Haemophilus at 6 months and at 12 months by Phascolarctobacterium. Putative inhibition or degradation of IgA by specific bacterial populations was observed at 6 months but not at 12 months.
IAP is produced by intestinal epithelial cells in response to bacterial lipopolysaccharide (LPS) produced by Gram-negative bacteria, and it serves to neutralise LPS and thus limit LPS-induced inflammation[15,47,48]. Alkaline phosphatase is also produced by bacteria, and it has been estimated that 20%-30% of faecal alkaline phosphatase activity is derived from bacteria[49,50]. Our IAP measurement may have suffered from neutral pH, as its activity is optimal at pH 10, but dilution with PBS rather than an alkaline buffer enabled the simultaneous analysis of multiple biomarkers from the same faecal water sample. In our data, IAP levels correlated with other markers of inflammation, but IAP increased together with IgA and LTF - molecules whose role is to limit inflammation by binding and eliminating microbes and antigens. A correlation between IAP and IgA has been shown before in mice and humans[50], suggesting that they may be regulated by common factors, or as suggested by Lassenius et al., immunoglobulin secretion may be stimulated by IAP[50]. Bacteroides appeared as the main putatively stimulatory bacterium of IAP at 6 months, and members of Proteobacteria and Negativicutes at 12 months. IAP counters the inhibitory effect of ATP on bacterial growth and is believed to affect the balance of gut microbes, as IAP KO mice are not colonised with Escherichia coli and have increased Clostridia levels[47,51,52]. Our data confirm similar associations in human infants. IAP thus emerges as a potential regulator of gut microbiota in human infants, appearing to be stimulated by Gram-negative bacteria and to inhibit the growth of Gram-positive bacteria.
BPI is a microbicidal protein with endotoxin-neutralising abilities produced by neutrophils[16]. We found BPI to be generally stimulated by the overall bacterial load, specifically by Proteobacteria, while its expression appeared to be attenuated by Lactobacillus at 6 months and by members of Clostridia at 12 months. BPI appeared to inhibit bacterial growth only at 12 months, when it was especially effective against Bilophila, known for its inflammatory effects[53]. BPI’s generally negative associations with bacterial growth fit its function as a microbicidal peptide. Our data indicate that BPI activity may mature after the introduction of solid foods, as it is not stimulated by high bacterial abundance nor inhibitory against bacteria at 6 months.
Markers of inflammation
Cal is a calcium-, zinc-, and manganese-binding protein secreted by neutrophils. It competes with bacteria (and fungi) for metals, thereby inhibiting their growth[19]. So far, it has been shown to inhibit the growth of diverse pathogens, including S. aureus, Acinetobacter baumannii, Helicobacter pylori, Salmonella enterica, Staphylococcus epidermidis, Staphylococcus lugdunensis, Enterococcus faecalis, Pseudomonas aeruginosa, and Shigella flexneri[54]. It is also proposed to inhibit bacterial binding of L. monocytogenes and S. enterica[55]. Its abundance in faeces is a marker of inflammation and IBD[56,57]. Most literature agrees that Cal levels in healthy infants are higher than in adults due to many factors, including fluctuation of microbiota[58]. In fact, lower Cal levels are associated with dysregulated microbiota and feeding intolerance in infants[57]. The levels tend to drop with age, stabilising at adult levels around a year of age[59]. We found higher Cal levels in C-section-born infants compared to vaginally born infants [Table 1]. Different studies have found higher Cal levels either in C-section-born infants[60] or vaginally born babies[58,61]. The differences may be due to differences in gut microbiota compositions, since we found faecal Cal to respond to microbial populations.
At 6 months, Lachnospiraceae and Akkermansia appeared to inhibit the secretion of Cal, while Akkermansia growth seemed to be inhibited by high Cal levels. Bifidobacteria were also negatively associated with Cal levels (albeit not significantly, therefore not shown), which is clinically relevant as supplementation with bifidobacteria has been proposed as a Cal-lowering intervention in babies[62]. At 12 months, Pseudoruminococcus and Prevotella appeared to stimulate Cal, and Cal seemed to have only positive effects on bacterial growth - most strongly on Pseudoruminococcus and Lactobacillus.
LTF is an iron-sequestering protein with antibacterial and antiviral activity that is found in breastmilk and is secreted by neutrophils in the gut in response to inflammation[20,63]. Because we found LTF to correlate with Cal, most of the LTF in our samples is likely gut-derived rather than originating in breastmilk. LTF has been proposed to reduce inflammation, neutralise endotoxins, and, most importantly, aid in commensal colonisation[63-65]. However, most of these studies are done in vitro or look at immune biomarkers rather than bacteria. Apart from Haemophilus at 6 months and Varibaculum at 12 months, we did not observe significant effects of LTF on gut microbes. This has also been reported after administering oral LTF to toddlers[66].
HD5 is an antimicrobial peptide produced by Paneth cells, known to selectively kill pathogens and preserve commensals[17]. It is increased in the inflamed colon of children with IBD and associated with atopic dermatitis[67,68]. We found that it correlates with ECP, suggestive of a common regulatory system, although ECP is produced by eosinophils[18]. Bacteria with known anti-inflammatory properties, such as Bifidobacterium (6 months) and Roseburia and Prevotella (12 months), appeared to reduce its expression, while Streptococcus, Megasphaera, and Clostridioides (6 months) appeared to stimulate it. Lactate has been shown to inhibit HD5[69], but we did not find associations between lactic acid bacteria and HD5. At 6 months, high levels of HD5 appeared to have a negative impact on Akkermansia. The association was not significant at 12 months, when high HD5 appeared to reduce the growth of Haemophilus, which contains potential pathogens[70-72]. In addition, at 12 months, HD5 appeared to stimulate the growth of several commensal bacteria, including Lactobacillus.
LCN2 is an iron-sequestering protein secreted by many cell types in the gut, including neutrophils, that inhibits bacterial growth by competing for iron and also has inflammatory effects[73]. LCN2 behaved generally differently from the other neutrophil-secreted markers, changing inversely with IAP. Previously, LCN2 has been shown to correlate with Cal in adult IBD patients[74], which we did not observe in this sample of healthy infants. Due to the association of LCN2 with low protein and bacteria concentrations and low pH, as well as with increasing abundances of small intestine organisms (Veillonellaceae and Streptococcacae[75]) and decreasing abundances of colon-dwelling organisms (Roseburia[75]), we suggest that LCN2 may be a potential indicator of fast gut transit in infants.
ECP is an eosinophil granulocyte-secreted protein that mediates the inflammatory response of the host to microbes and parasites and is elevated in the serum of individuals with atopic diseases such as allergic rhinitis and asthma[18]. Levels of ECP also correlate with the disease severity of ulcerative colitis[76]. It is cytotoxic by inducing apoptosis yet is also involved in immune modulation and tissue repair[18]. ECP has antibacterial effects[18]. In our data, it correlated with IAP and HD5. ECP appeared to be stimulated by Streptococcaceae and Clostridioides, while Collinsella seemed to reduce its expression at 6 months. Associations at 12 months were weaker, but Enterococcus appeared to stimulate it and some members of Clostridia and Haemophilus to inhibit it. ECP seemed to inhibit Akkermansia growth at 12 months. Lysozyme is an antimicrobial peptide that cleaves peptidoglycan, the major component of Gram-positive bacterial cell walls[77]. It is an important component of human breastmilk, yet it has seemingly conflicting properties: both increased and decreased levels have offered protection against colitis in previous studies[78-80]. The effect of lysozyme levels is, therefore, likely to depend on the microbiota. Changes in lysozyme levels were associated with bacterial population growth. At 6 months, bacterial growth appeared to inhibit lysozyme, while at 12 months, we observed both stimulatory and inhibitory associations. Lysozyme has been linked with increased IgA in piglets[81], which we confirmed. While lysozyme has been associated with increasing lactobacilli in piglets, we did not find lysozyme to be a strong regulator of the microbiota in human infants[79,81,82].
Zonulin is a protein that reversibly increases the permeability of tight junctions and serves as a biomarker for intestinal barrier integrity[83]. Elevated zonulin levels have been associated with many intestinal diseases where barrier dysfunction is involved, such as celiac disease, IBD, and necrotising enterocolitis[84]. Its correlation with gut microbiota has been studied in a variety of different settings, and it is elevated in combination with higher levels of gram-negative strains or opportunistic pathogens, such as Clostridium, while a higher prevalence of gram-positive bacteria is linked to lower zonulin levels[84]. In our data, zonulin appeared to be stimulated by several Gram-positive genera at 12 months, but not at 6 months. On the other hand, zonulin appeared to stimulate the growth of Flavonifractor, Bilophila, and Blautia, suggesting that these bacteria may benefit from increased permeability or inflammation.
Limitations
This research has analysed 216 samples so far. According to power calculation, with a sample size of 216, we could detect a correlation coefficient of 0.19 or larger at the 0.05 P-value cut-off and with a 0.2 type II error rate. The sample size is thus sufficient to detect modest associations. However, these samples are derived from 8 faecal time series of infants from the same area in Finland, meaning that the correlations found here might not be representative of infants in general and would need to be replicated in an independent cohort to test reproducibility and generalisability of the found associations. Most (3 out of 4) 11-12-month-olds were still breastfed, and we, therefore, do not have sufficient representative data for infants who solely consume solid food or formula milk. We did not control diet, and thus, infants present with a natural variation in microbiome based on variations in their diet in addition to any inherent variation between infants. Future research will expand the variety of infants sampled, enabling a more robust correlation of biomarkers, microbiome, and gut health. While we were able to observe consistent associations between microbial population growth and biomarkers’ abundances and changes, these do not enable causal inference.
Conclusions
Despite the limited sample variety, this study provides a novel perspective on microbiota-host interactions in the infant gut during a critical time of immune system maturation. Our results add a dynamic perspective to host-microbe interactions, suggesting that gut permeability and immune system responses in healthy infants may fluctuate in association with the microbial stimuli, which is likely important in the maintenance of gut homeostasis. We observed a change in the nature of microbe-host interactions from apparent immune tolerance at 6 months toward more tight regulation at 12 months. IAP activity, measured by the Quanti-Blue assay, and muc2, measured by ELISA, emerged as potentially important regulators of gut microbiota in infants. The study demonstrates the utility of biomarker and bacteria profiling from daily stool samples as a potent tool for analysing in vivo associations between the immune system and the gut microbiota.
DECLARATIONS
Authors’ contributions
Made substantial contributions to the conception and design of the study and performed data analysis and interpretation: van Beek N, Korpela K
Performed data acquisition, as well as providing administrative, technical, and material support: Katavisto I, van Beek N, Korpela K, Lehto M, Kolho KL, de Vos WM, Salonen A
Availability of data and materials
The data that support the findings of this study, the taxonomic tables and biomarker concentrations, are available as supplementary data.
Financial support and sponsorship
This work was supported by ERC Starting Grant 101039583 - MICROECO.
Conflicts of interest
All authors declared that there are no conflicts of interest.
Ethical approval and consent to participate
The research was approved by the Helsinki University Hospital Ethics Committee (HUS/2346/2016). Informed consent was obtained from the parents of the study subjects.
Consent for publication
Not applicable.
Copyright
© The Author(s) 2024.
REFERENCES
1. de Vos WM, Tilg H, Van Hul M, Cani PD. Gut microbiome and health: mechanistic insights. Gut 2022;71:1020-32.
2. Bogaert D, van Beveren GJ, de Koff EM, et al. Mother-to-infant microbiota transmission and infant microbiota development across multiple body sites. Cell Host Microbe 2023;31:447-60.e6.
3. Homann CM, Rossel CAJ, Dizzell S, et al. Infants’ first solid foods: impact on gut microbiota development in two intercontinental cohorts. Nutrients 2021;13:2639.
4. Differding MK, Benjamin-Neelon SE, Hoyo C, Østbye T, Mueller NT. Timing of complementary feeding is associated with gut microbiota diversity and composition and short chain fatty acid concentrations over the first year of life. BMC Microbiol 2020;20:56.
5. Hou K, Wu ZX, Chen XY, et al. Microbiota in health and diseases. Signal Transduct Target Ther 2022;7:135.
6. Tanaka M, Nakayama J. Development of the gut microbiota in infancy and its impact on health in later life. Allergol Int 2017;66:515-22.
7. Yu JC, Khodadadi H, Malik A, et al. Innate immunity of neonates and infants. Front Immunol 2018;9:1759.
8. Liu W, Hu D, Huo H, et al. Intestinal alkaline phosphatase regulates tight junction protein levels. J Am Coll Surg 2016;222:1009-17.
9. Jokela R, Ponsero AJ, Dikareva E, et al. Sources of gut microbiota variation in a large longitudinal Finnish infant cohort. EBioMedicine 2023;94:104695.
10. Korpela K, Hurley S, Ford SA, et al; CORAL Study Group. Association between gut microbiota development and allergy in infants born during pandemic-related social distancing restrictions. Allergy 2024;79:1938-51.
11. Sanna S, Kurilshikov A, van der Graaf A, Fu J, Zhernakova A. Challenges and future directions for studying effects of host genetics on the gut microbiome. Nat Genet 2022;54:100-6.
12. Jian C, Luukkonen P, Yki-Järvinen H, Salonen A, Korpela K. Quantitative PCR provides a simple and accessible method for quantitative microbiota profiling. PLoS One 2020;15:e0227285.
13. Jian C, Salonen A, Korpela K. Commentary: how to count our microbes? The effect of different quantitative microbiome profiling approaches. Front Cell Infect Microbiol 2021;11:627910.
14. Pang T, Leach ST, Katz T, Day AS, Ooi CY. Fecal biomarkers of intestinal health and disease in children. Front Pediatr 2014;2:6.
15. Singh SB, Lin HC. Role of intestinal alkaline phosphatase in innate immunity. Biomolecules 2021;11:1784.
16. Theprungsirikul J, Skopelja-Gardner S, Rigby WFC. Killing three birds with one BPI: bactericidal, opsonic, and anti-inflammatory functions. J Transl Autoimmun 2021;4:100105.
17. Bevins CL, Salzman NH. Paneth cells, antimicrobial peptides and maintenance of intestinal homeostasis. Nat Rev Microbiol 2011;9:356-68.
18. Topic RZ, Dodig S. Eosinophil cationic protein--current concepts and controversies. Biochem Med 2011;21:111-21.
19. Damo SM, Kehl-Fie TE, Sugitani N, et al. Molecular basis for manganese sequestration by calprotectin and roles in the innate immune response to invading bacterial pathogens. Proc Natl Acad Sci U S A 2013;110:3841-6.
20. Kell DB, Heyden EL, Pretorius E. The biology of lactoferrin, an iron-binding protein that can help defend against viruses and bacteria. Front Immunol 2020;11:1221.
21. Takeuchi T, Ohno H. IgA in human health and diseases: potential regulator of commensal microbiota. Front Immunol 2022;13:1024330.
22. Johansson MEV, Holmén Larsson JM, Hansson GC. The two mucus layers of colon are organized by the MUC2 mucin, whereas the outer layer is a legislator of host-microbial interactions. Proc Natl Acad Sci U S A 2011;108 Suppl 1:4659-65.
23. Wang L, Llorente C, Hartmann P, Yang AM, Chen P, Schnabl B. Methods to determine intestinal permeability and bacterial translocation during liver disease. J Immunol Methods 2015;421:44-53.
24. Korpela K, Dikareva E, Hanski E, Kolho KL, de Vos WM, Salonen A. Cohort profile: Finnish Health and Early Life Microbiota (HELMi) longitudinal birth cohort. BMJ Open 2019;9:e028500.
25. Salonen A, Nikkilä J, Jalanka-Tuovinen J, et al. Comparative analysis of fecal DNA extraction methods with phylogenetic microarray: effective recovery of bacterial and archaeal DNA using mechanical cell lysis. J Microbiol Methods 2010;81:127-34.
26. Dubois L, Valles-Colomer M, Ponsero A, et al. Paternal and induced gut microbiota seeding complement mother-to-infant transmission. Cell Host Microbe 2024;32:1011-24.e4.
27. Chen S. Ultrafast one-pass FASTQ data preprocessing, quality control, and deduplication using fastp. Imeta 2023;2:e107.
28. Danecek P, Bonfield JK, Liddle J, et al. Twelve years of SAMtools and BCFtools. Gigascience 2021;10:giab008.
29. Hiseni P, Rudi K, Wilson RC, Hegge FT, Snipen L. HumGut: a comprehensive human gut prokaryotic genomes collection filtered by metagenome data. Microbiome 2021;9:165.
30. Katrikorpela. mare. 2016. Available from: https://zenodo.org/records/50310. [Last accessed on 25 Dec 2024].
34. Van den Abbeele P, Deyaert S, Thabuis C, et al. Bridging preclinical and clinical gut microbiota research using the ex vivo SIFR® technology. Front Microbiol 2023;14:1131662.
35. Hugenholtz F, de Vos WM. Mouse models for human intestinal microbiota research: a critical evaluation. Cell Mol Life Sci 2018;75:149-60.
36. Nguyen TL, Vieira-Silva S, Liston A, Raes J. How informative is the mouse for human gut microbiota research? Dis Model Mech 2015;8:1-16.
37. Ballard O, Morrow AL. Human milk composition: nutrients and bioactive factors. Pediatr Clin North Am 2013;60:49-74.
38. Henrick BM, Hutton AA, Palumbo MC, et al. Elevated fecal pH indicates a profound change in the breastfed infant gut microbiome due to reduction of Bifidobacterium over the past century. mSphere 2018;3:e00041-18.
39. Yamamura R, Inoue KY, Nishino K, Yamasaki S. Intestinal and fecal pH in human health. Front Microbiomes 2023;2:1192316.
40. Marchant A, Kollmann TR. Understanding the ontogeny of the immune system to promote immune-mediated health for life. Front Immunol 2015;6:77.
41. Nilsen M, Lokmic A, Angell IL, et al. Fecal microbiota nutrient utilization potential suggests mucins as drivers for initial gut colonization of mother-child-shared bacteria. Appl Environ Microbiol 2021;87:e02201-20.
42. Ruas-Madiedo P, Gueimonde M, Fernández-García M, de los Reyes-Gavilán CG, Margolles A. Mucin degradation by Bifidobacterium strains isolated from the human intestinal microbiota. Appl Environ Microbiol 2008;74:1936-40.
43. Lemme-Dumit JM, Song Y, Lwin HW, et al. Altered gut microbiome and fecal immune phenotype in early preterm infants with leaky gut. Front Immunol 2022;13:815046.
44. Szymanska E, Wierzbicka A, Dadalski M, Kierkus J. Fecal zonulin as a noninvasive biomarker of intestinal permeability in pediatric patients with inflammatory bowel diseases-correlation with disease activity and fecal calprotectin. J Clin Med 2021;10:3905.
45. Mantis NJ, Rol N, Corthésy B. Secretory IgA’s complex roles in immunity and mucosal homeostasis in the gut. Mucosal Immunol 2011;4:603-11.
46. Pabst O, Slack E. IgA and the intestinal microbiota: the importance of being specific. Mucosal Immunol 2020;13:12-21.
47. Fawley J, Gourlay DM. Intestinal alkaline phosphatase: a summary of its role in clinical disease. J Surg Res 2016;202:225-34.
48. Martins RDS, Kooi EMW, Poelstra K, Hulscher JBF. The role of intestinal alkaline phosphatase in the development of necrotizing enterocolitis. Early Hum Dev 2023;183:105797.
49. Malo MS. A high level of intestinal alkaline phosphatase is protective against type 2 diabetes mellitus irrespective of obesity. EBioMedicine 2015;2:2016-23.
50. Lassenius MI, Fogarty CL, Blaut M, et al; FinnDiane Study Group. Intestinal alkaline phosphatase at the crossroad of intestinal health and disease - a putative role in type 1 diabetes. J Intern Med 2017;281:586-600.
51. Estaki M, DeCoffe D, Gibson DL. Interplay between intestinal alkaline phosphatase, diet, gut microbes and immunity. World J Gastroenterol 2014;20:15650-6.
52. Malo MS, Moaven O, Muhammad N, et al. Intestinal alkaline phosphatase promotes gut bacterial growth by reducing the concentration of luminal nucleotide triphosphates. Am J Physiol Gastrointest Liver Physiol 2014;306:826-38.
53. Garrett WS, Onderdonk A. 249 - Bacteroides, Prevotella, Porphyromonas, and Fusobacterium Species (and other medically important anaerobic gram-negative bacilli). In: Mandell, Douglas, and Bennett’s Principles and Practice of Infectious Diseases. Elsevier; 2015. pp. 2773-80.
54. Juttukonda LJ, Skaar EP. Manganese and nutritional immunity. In: Molecular, genetic, and nutritional aspects of major and trace minerals. Elsevier; 2017. pp. 377-87.
55. Nisapakultorn K, Ross KF, Herzberg MC. Calprotectin expression inhibits bacterial binding to mucosal epithelial cells. Infect Immun 2001;69:3692-6.
56. Heinzel S, Jureczek J, Kainulainen V, et al. Elevated fecal calprotectin is associated with gut microbial dysbiosis, altered serum markers and clinical outcomes in older individuals. Sci Rep 2024;14:13513.
57. Hong L, Huang Y, Han J, et al. Dynamics and crosstalk between gut microbiota, metabolome, and fecal calprotectin in very preterm infants: insights into feeding intolerance. Nutrients 2023;15:4849.
58. Lee YM, Min CY, Choi YJ, Jeong SJ. Delivery and feeding mode affects fecal calprotectin levels in infants < 7 months old. Early Hum Dev 2017;108:45-8.
59. Kolho KL, Alfthan H. Concentration of fecal calprotectin in 11,255 children aged 0-18 years. Scand J Gastroenterol 2020;55:1024-7.
60. Sommermeyer H, Bernatek M, Pszczola M, Krauss H, Piatek J. Supporting the diagnosis of infantile colic by a point of care measurement of fecal calprotectin. Front Pediatr 2022;10:978545.
61. Łoniewska B, Adamek K, Węgrzyn D, et al. Analysis of faecal zonulin and calprotectin concentrations in healthy children during the first two years of life. An observational prospective cohort study. J Clin Med 2020;9:777.
62. Cekovic JR, Prodanovic NS, Mijailovic SS, et al. The perinatal factors that influence the excretion of fecal calprotectin in premature-born children. Open Med 2022;17:1275-81.
63. Zhao C, Chen N, Ashaolu TJ. Prebiotic and modulatory evidence of lactoferrin on gut health and function. J Funct Foods 2023;108:105741.
64. Mastromarino P, Capobianco D, Campagna G, et al. Correlation between lactoferrin and beneficial microbiota in breast milk and infant’s feces. Biometals 2014;27:1077-86.
65. Sherman MP, Sherman J, Arcinue R, Niklas V. Randomized control trial of human recombinant lactoferrin: a substudy reveals effects on the fecal microbiome of very low birth weight infants. J Pediatr 2016;173 Suppl:S37-42.
66. González L, Sosa JLP, Mosquito S, et al. Oral lactoferrin administration does not impact the diversity or composition of the infant gut microbiota in a Peruvian cohort. Microbiol Spectr 2023;11:e0009623.
67. Zilbauer M, Jenke A, Wenzel G, et al. Intestinal alpha-defensin expression in pediatric inflammatory bowel disease. Inflamm Bowel Dis 2011;17:2076-86.
68. Savilahti EM, Kukkonen AK, Haahtela T, Tuure T, Kuitunen M, Savilahti E. Intestinal defensin secretion in infancy is associated with the emergence of sensitization and atopic dermatitis. Clin Exp Allergy 2012;42:405-11.
69. Sugi Y, Takahashi K, Kurihara K, et al. α-Defensin 5 gene expression is regulated by gut microbial metabolites. Biosci Biotechnol Biochem 2017;81:242-8.
70. Ekkelenkamp MB, Rooijakkers SHM, Bonten MJM. Chapter 165 - Staphylococci and micrococci. In: Infectious diseases. Elsevier; 2010. pp. 1632-44.
71. Musher DM. Chapter 30 Haemophilus species. In: Medical microbiology. 1996. Available from: https://www.ncbi.nlm.nih.gov/books/NBK8458/. [Last accessed on 25 Dec 2024].
72. Shao Y, Forster SC, Tsaliki E, et al. Stunted microbiota and opportunistic pathogen colonization in caesarean-section birth. Nature 2019;574:117-21.
73. Asaf S, Maqsood F, Jalil J, et al. Lipocalin 2 - not only a biomarker: a study of current literature and systematic findings of ongoing clinical trials. Immunol Res 2023;71:287-313.
74. Zollner A, Schmiderer A, Reider SJ, et al. faecal biomarkers in inflammatory bowel diseases: calprotectin versus lipocalin-2-a comparative study. J Crohns Colitis 2021;15:43-54.
75. Jensen BAH, Heyndrickx M, Jonkers D, et al. Small intestine vs. colon ecology and physiology: why it matters in probiotic administration. Cell Rep Med 2023;4:101190.
76. Hogan SP, Rothenberg ME. Eosinophil function in eosinophil-associated gastrointestinal disorders. Curr Allergy Asthma Rep 2006;6:65-71.
77. Sindi AS, Stinson LF, Lai CT, et al. Human milk lactoferrin and lysozyme concentrations vary in response to a dietary intervention. J Nutr Biochem 2025;135:109760.
78. Rubio CA. The natural antimicrobial enzyme lysozyme is up-regulated in gastrointestinal inflammatory conditions. Pathogens 2014;3:73-92.
79. Zhang C, Xiang C, Zhou K, et al. Intestinal lysozyme1 deficiency alters microbiota composition and impacts host metabolism through the emergence of NAD+-secreting ASTB Qing110 bacteria. mSystems 2024;9:e0121423.
80. Yang B, Wang J, Tang B, et al. Characterization of bioactive recombinant human lysozyme expressed in milk of cloned transgenic cattle. PLoS One 2011;6:e17593.
81. Huang G, Li X, Lu D, et al. Lysozyme improves gut performance and protects against enterotoxigenic Escherichia coli infection in neonatal piglets. Vet Res 2018;49:20.
82. Wu Y, Cheng B, Ji L, et al. Dietary lysozyme improves growth performance and intestinal barrier function of weaned piglets. Anim Nutr 2023;14:249-58.
83. Humphreys C. Intestinal permeability. Textbook of natural medicine. Elsevier; 2020. pp. 166-77.e4.
Cite This Article

How to Cite
van Beek, N.; Katavisto, I.; Lehto, M.; Kolho, K. L.; de Vos, W. M.; Salonen, A.; Korpela, K. Host-microbiota interactions in the infant gut revealed by daily faecal sample time series. Microbiome. Res. Rep. 2025, 4, 13. http://dx.doi.org/10.20517/mrr.2024.45
Download Citation
Export Citation File:
Type of Import
Tips on Downloading Citation
Citation Manager File Format
Type of Import
Direct Import: When the Direct Import option is selected (the default state), a dialogue box will give you the option to Save or Open the downloaded citation data. Choosing Open will either launch your citation manager or give you a choice of applications with which to use the metadata. The Save option saves the file locally for later use.
Indirect Import: When the Indirect Import option is selected, the metadata is displayed and may be copied and pasted as needed.
About This Article
Special Issue
Copyright
Data & Comments
Data
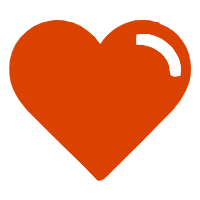
Comments
Comments must be written in English. Spam, offensive content, impersonation, and private information will not be permitted. If any comment is reported and identified as inappropriate content by OAE staff, the comment will be removed without notice. If you have any queries or need any help, please contact us at support@oaepublish.com.