Artificial intelligence as an adjunctive tool in hand and wrist surgery: a review
Abstract
Artificial intelligence (AI) is currently utilized across numerous medical disciplines. Nevertheless, despite its promising advancements, AI’s integration in hand surgery remains in its early stages and has not yet been widely implemented, necessitating continued research to validate its efficacy and ensure its safety. Therefore, this review aims to provide an overview of the utilization of AI in hand surgery, emphasizing its current application in clinical practice, along with its potential benefits and associated challenges. A comprehensive literature search was conducted across PubMed, Embase, Medline, and Cochrane libraries, adhering to the Preferred reporting items for systematic reviews and meta-analyses (PRISMA) guidelines. The search focused on identifying articles related to the application of AI in hand surgery, utilizing multiple relevant keywords. Each identified article was assessed based on its title, abstract, and full text. The primary search identified 1,228 articles; after the application of inclusion/exclusion criteria and manual bibliography search of included articles, a total of 98 articles were covered in this review. AI’s primary application in hand and wrist surgery is diagnostic, which includes hand and wrist fracture detection, carpal tunnel syndrome (CTS), avascular necrosis (AVN), and osteoporosis screening. Other applications include residents’ training, patient-doctor communication, surgical assistance, and outcome prediction. Consequently, AI is a very promising tool that has numerous applications in hand and wrist surgery, though further research is necessary to fully integrate it into clinical practice.
Keywords
INTRODUCTION
Recent advancements in artificial intelligence (AI) have significantly driven its integration into numerous medical and surgical fields, enhancing diagnostic accuracy and improving patient care. The notion of AI was initially introduced by John McCarthy, an American computer scientist, in 1956[1]. Since then, AI has branched out into various fields, such as machine learning (ML), deep learning (DL), natural language processing (NLP), and robotics[2]. ML makes predictions based on the detection of patterns in data. When trained on labeled data, it is considered supervised learning and its implications in medicine include data classification for diagnostic purposes and outcome predictions. On the other hand, unsupervised learning consists of ML algorithms trained with unlabeled data. The goal of unsupervised learning is to identify hidden patterns within the data without predefined outcomes[3]. Clinical applications for such algorithms include the identification of disease risk factors[4]. Given these various applications and the significant potential of ML in improving care, extensive efforts are underway to facilitate its integration in clinical settings[5]. As for DL, it is a branch of ML that uses multiple layers of neural networks to enhance the accuracy of pattern recognition[2]. It is particularly useful in the analysis of medical images, facilitating the diagnosis process[6]. Therefore, to date, medical imaging is the medical field that has benefitted the most from AI development[7,8]. In this area, ML is used to enhance diagnostic accuracy and efficiency. Finally, NLP, another branch of ML, has the potential to understand and interpret words and provide a response[2,6].
In surgical fields, AI has been shown to increase precision, reduce errors, and optimize preoperative planning and operating room workflow[9]. Furthermore, it has the capability to predict surgical outcomes and postoperative complications[10]. Considering its significant potential to improve patient care, increased research is currently being done to determine its application in different surgical fields, including plastic surgery. AI is becoming increasingly valuable in plastic surgery, especially for tasks requiring visual diagnosis, such as assessing preoperative and postoperative aesthetics[11]. Similarly, ML algorithms have also been used to improve outcome assessments in various procedures, such as rhinoplasty[12,13]. Additionally, facial recognition tools, a subtype of supervised learning in ML, have the potential to demonstrate the projected results of aesthetic surgeries, thereby assisting in managing patient expectations[2,14].
Few systematic reviews have explored the role of AI in plastic surgery in recent years[2,3,11,13]. While these reviews provide medical professionals with valuable insights into the emerging applications of AI across various fields of plastic surgery, they also highlight the need for further research in certain areas. Notably, Mantelakis et al. noted a significant gap in AI research related to hand surgery and the use of ML in this domain[3]. In addition, three[2,3,11] of the four systematic reviews were limited to articles published up to 2020, and the fourth[13] covered articles up to 2021. Given the rapidly increasing literature on the applications of AI in medicine, the aim of this systematic review was to provide a comprehensive analysis of its application within the field of hand and wrist surgery.
METHODS
A comprehensive literature search was conducted across PubMed, Embase, Medline and Cochrane libraries, adhering to the preferred reporting items for systematic reviews and meta-analyses (PRISMA) guidelines[15]. The search focused on identifying articles related to the application of AI in hand surgery, using multiple relevant keywords. Each identified article was assessed based on its title, abstract, and full text. The primary search was conducted on August 6, 2024, utilizing the following keywords in articles’ titles and abstracts: “Artificial Intelligence” OR “Computer-Aid” OR “Machine learning” OR “ChatGPT” along with specific terms related to hand surgery such as “hand surgery” OR “wrist surgery” OR “plastic surgery” OR “wrist” OR “finger” OR “Peripheral Nerve Surgery” OR “scaphoid” OR “carpal bone” OR “thumb”. A variety of terms for AI were included to ensure comprehensive coverage of ML-related articles in the field of hand surgery.
In total, 1,228 articles were identified and screened using the Covidence platform. Two reviewers initially screened the articles based on the relevance of titles and abstracts. All articles that did not refer to the application of AI to specific concepts related to hand or wrist surgery were excluded. Two hundred and twenty-five articles advanced to full-text screening. The inclusion and exclusion criteria applied are included in Table 1. Excluded articles included duplicates, letters to the editor, systematic reviews, non-English publications, content unrelated to hand or wrist surgery, and articles published over a decade ago. A full-text review conducted by a single reviewer led to the extraction of 90 articles, which were subsequently confirmed by a second reviewer. Each included study then underwent a manual bibliographic review to identify other relevant studies that were not included in the primary search. This process led to the inclusion of eight additional articles, bringing the total number of articles covered in this review to 98.
Inclusion and exclusion criteria of the systematic review
Inclusion criteria | Exclusion criteria |
Articles on the integration of AI in hand and wrist surgery Articles published between 2014-2024 | Letters to the editor Systematic reviews Articles not related to hand or wrist surgery Languages other than English Articles related to prosthetic hand or arm |
The focus of this study was to explore the application of ML in the diagnosis and management of various hand conditions, including hand and wrist fractures, peripheral nerve injuries, carpal tunnel syndrome (CTS), osteoarthritis (OA), and triangular fibrocartilage complex (TFCC) disorders. By examining these innovative technologies, this study seeks to assist hand surgeons in integrating ML into their practice. Therefore, an emphasis is placed on evaluating the performance of AI as well as its potential to enhance resident training and improve patient communication. However, this research does not address rehabilitation or the use of prosthetic arms and hands following nerve injury or amputations [Figure 1].
RESULTS
Use of large language model in AI
This review identified ten articles that focused on AI’s performance in executing various tasks relating to hand surgery.
A common area in which AI’s performances were evaluated was answering hand surgery multiple-choice exam questions. Thibaut et al. compared ChatGPT-3.5’s performance to Google’s Bard Chatbot[16]. Both large language models (LLMs) were tasked with answering 18 questions from the European Board of Hand Surgery (EBHS). This study showed that both platforms failed to obtain a passing score and did not adapt their response even after the authors provided the correct answer. A similar study carried out in 2024 tasked ChatGPT-3.5 and ChatGPT-4 with answering the 2021 and 2022 Self-Assessment Examinations (SAE) of the American Society for Surgery of the Hand (ASSH)[17]. ChatGPT-4 performed significantly better, with an overall score of 68.9%, compared to ChatGPT 3.5’s 58.0%. These findings align with Ghanem et al.’s study, which reported that ChatGPT-4 achieved an overall passing score of 61.98% in the ASSH 2019 exam[18]. Despite ChatGPT’s improvement with newer versions, most studies highlighted it is limited by its incapacity to take clinical context into consideration[17-20].
In contrast to other studies, Leypold et al. showed that ChatGPT-4 was successful in understanding upper limb surgical scenarios and in identifying relevant treatment options[21]. These results do not entirely align with the findings of Seth et al., who showed that although the information provided by ChatGPT was accurate, it was mostly superficial and limited to well-studied information[20]. This suggests that ChatGPT might not be able to interpret more complex cases.
Similarly, Seth et al. conducted two more studies to evaluate ChatGPT’s knowledge and reliability for common hand surgery conditions, focusing on CTS[22] and scaphoid fracture management[23]. The same methodology was used to assess both conditions. The authors concluded that the algorithm showed a good understanding of the questions and provided logical answers that were easily understandable. Nevertheless, some responses were deemed superficial, lacking detailed explanations, and occasionally, the AI model included references to nonexistent publications.
Ajmera et al. explored a different aspect of ChatGPT’s performance by assessing its performance in generating anatomical images of six different joints, including the wrist[24]. This study concluded that ChatGPT’s performance was below average, with significant anatomical errors such as incorrect articulations, missing or fake bones.
AI-assisted fracture detection
The main application of AI in hand and wrist surgery remains the detection of fractures. Identified studies predominantly addressed distal radius fractures, ulna styloid fractures, distal ulna fractures, and scaphoid fractures.
In 2017, Olczak et al. were one of the first to demonstrate that DL networks performed similarly to senior orthopedic surgeons in identifying fractures. Their study used a dataset of 256,458 wrist, hand, and ankle radiographs to train and test five DL networks. The best-performing network achieved an 83% accuracy in identifying fractures, comparable to senior orthopedic surgeons[25]. Similarly, Lee et al. found that AI assistance improved inexperienced radiologists’ accuracy in scaphoid fracture detection[26]. Lindsey et al. also used a DL network to detect wrist fractures, aiming to assist physicians in their diagnosis[27]. Overall, there was a 47.0% reduction in misinterpretation rate. These findings are consistent with Cohen et al.’s study, which noted an increase in wrist fracture detection by non-specialized radiologists when assisted by AI[28]. While these studies show promise, it is important to note that the sensitivity of AI is not perfect and that their performance might be reduced with more complex fractures. In 2022, Hardalaç et al. combined five ensemble models to create the “wrist fracture detection-combo (WFD-C)”, which had the highest detection rate compared to other models, with an 86.39% average precision[29].
Lysdahlgaard explored a different approach for AI automated fracture detection by investigating the potential of heat maps[30]. Using the MURA dataset, 20 ML models were used to interpret heat maps generated from X-rays. The overall accuracy for all the models combined was 81% for wrist radiographs. Alammar et al. also used the MURA dataset to collect radiographs of the humerus and wrist to enhance the performance of a pre-trained convolutional neural network (CNN) model, originally adapted from ImageNet[31]. The proposed algorithm achieved an AUC of 0.856, thereby outperforming ImageNet models that did not receive additional training. Similarly, in 2024, Jacques et al. compared the performance of 23 radiologists with varying levels of experience with and without AI assistance[32]. BoneView (Gleamer), the DL model used in this study, achieved an AUC of 0.764 for fracture detection. AI assistance enhanced radiologists’ sensitivity by 4.5% but did not affect their specificity.
Distal radius fracture
Kim and MacKinnon were among the firsts to apply a CNN specifically to the detection of distal radius fracture. In their study, a pretrained Inception v3 network was enhanced using a set of lateral wrist radiographs[33]. While this study successfully demonstrated a proof of concept where the model achieved an AUC of 0.954, researchers acknowledged that incorporating a second imaging view could potentially improve the model’s diagnostic performance. In 2019, Gan et al. further explored the application of AI in distal radius fracture detection by using anterior-posterior (AP) views instead of lateral projection[34]. They trained the Inception-v4 model as a diagnostic tool and the Faster region-based convolutional neural network (R-CNN) model as an auxiliary algorithm tasked with identifying regions of interest within the radiographs. The Inception-v4 model achieved an AUC of 0.96 and an overall diagnostic accuracy of 93%. Their findings also suggest that AI achieves detection rates comparable to those of an experienced orthopedic surgeon when using AP radiographs. Similarly, Thian et al. utilized the Inception-ResNet and the Faster R-CNN models, training them on 7356 postero-anterior (PA) and lateral wrist radiographs to detect fractures[35]. The combined model achieved a diagnostic accuracy of 91.2% for radius fractures and 96.3% for ulna fractures on the PA and lateral projections, respectively. Oka et al. trained a different DL model, VGG16, on 498 AP images and 485 lateral radiographs of the distal radius, as well as 491 images of the styloid process of the ulna. The model demonstrated a diagnostic accuracy of 98.0% (AUC 0.991) for distal radius fractures and 91.1% (AUC 0.991) for fractures of the styloid process[36].
Russe et al. also conducted a study evaluating various AI models for detecting distal radius fractures by using three models: a classification model to recognize fractures images, a segmentation model to locate precise fracture boundaries within images, and a detection model to identify fractures[37]. This model achieved high accuracies, up to 97%, and effective fracture localization. Likewise, Zhang et al. developed and evaluated a DL algorithm for diagnosing distal radius fractures based on X-ray images[38]. Their study included a total of 3,276 wrist X-ray films. The DL model achieved a high accuracy of 97.03%, with a sensitivity of 95.70% and a specificity of 98.37%, outperforming both orthopedic and radiology attending physicians. Anttila et al. employed a segmentation-based U-net model, which accurately identified distal radius fracture with an AUC of 0.97 for radiographs without casts[39]. Accuracy was better in PA views compared to lateral views.
As for Mert et al., they evaluated ChatGPT 4’s capability in detecting distal radius fractures through radiological images, comparing it with human specialists (a hand surgery resident, a medical student) and an AI system (Gleamer BoneViewTM)[40]. The results indicate that ChatGPT 4 demonstrates good diagnostic accuracy, significantly outperforming the medical student, but was outperformed by both the hand surgery resident and Gleamer BoneViewTM.
Scaphoid fracture
Our review identified 11 articles focusing on the role of AI in detecting scaphoid fractures, with two specially examining its potential in identifying occult fractures.
In 2020, Ozkaya et al. conducted a study to evaluate a CNN model’s ability to detect scaphoid fractures using AP wrist radiographs[41]. The CNN model achieved an AUC of 0.840, performing comparably to the less experienced orthopedic surgeon, and it surpassed the ED physician (0.760 AUC), but was outperformed by the expert hand surgeon (0.920 AUC).
Similarly, Hendrix et al. compared a self-developed CNN model’s performance in detecting scaphoid fractures on AP and PA hand radiographs to that of 11 radiologists[42]. The segmentation CNN achieved a Dice coefficient of 0.974 while the fracture detection CNN achieved an AUC of 0.87, performing comparably to the radiologists. It is important to note that in this study, radiologists were limited to a single view for fracture detection, whereas multiple views are generally used in clinical settings.
In a follow-up study, Hendrix et al. expanded on their previous work by using a larger dataset to train the same CNN model and compared its performance to five MSK radiologists[43]. In this study, the CNN model achieved an AUC of 0.88, slightly outperforming the radiologists. The inclusion of ulnar-deviated and oblique views enhanced the model’s accuracy. The results also demonstrated that the CNN model reduced the reading time for four out of five radiologists by 49.4%. Nevertheless, it was noted that AI integration did not significantly improve most radiologists’ diagnostic accuracy.
In 2021, Tung et al. also published on this topic by comparing multiple CNN models in detecting scaphoid fractures[44]. Among the models without additional transfer learning training, DN121 had the highest AUC with 0.810, while VGG16 demonstrated the highest precision with 100% accuracy and 1.00 specificity. After the application of transfer learning, RN101 achieved the highest AUC with 0.950. Yang et al. also proposed a combination of two CNN models for scaphoid area segmentation and fracture detection[45]. The Faster R-CNN was used to identify the fracture region, followed by ResNet to detect the presence of fracture. The study utilized a dataset of scaphoid radiographs, which included 31 images of occult fractures. The proposed algorithm achieved an AUC of 0.917 for scaphoid fracture detection.
Scaphoid fracture prediction
Bulstra et al. trained five ML models to calculate scaphoid fracture probability using clinical and demographic features such as mechanism of injury, sex, age, affected side, and examination maneuvers[46]. All models achieved an AUC above 0.72, with Boosted decision tree outperforming the others with an AUC of 0.77. Pain over the scaphoid on ulnar deviation and male sex were the predictors with the highest correlation to scaphoid fractures. Using these features, an algorithm was developed suggesting that patients with radial-sided wrist pain, negative radiographs, and a fracture probability of 10% or more should undergo further imaging. When applied to the study’s patients, the algorithm achieved 100% sensitivity and reduced the need for additional imaging by 36% without overlooking any fractures.
Occult fracture detection
Langerhuizen et al. advanced research in this field by exploring AI’s potential in detecting occult scaphoid fractures, aiming to enhance radiologists’ detection capabilities[47]. The single-step pretrained CNN used in this study achieved an AUC of 0.77, performing similarly to orthopedic surgeons but with a lower specificity. The model was also able to detect five out of six occult scaphoid fractures that were missed by human experts, but it struggled with detecting fractures that were obvious to human observers. Yoon et al. also explored the detection of occult scaphoid fractures by developing a three-step model[48]. The first CNN was tasked with segmentation, while the second model was used to detect scaphoid fracture. As for the third, its role was to analyze the cases that were considered negative by the previous AI, aiming to detect overlooked fractures. This CNN model successfully detected 90.9% of occult fractures, correctly identifying 20 out of 22 cases.
Raisuddin et al. published a study focusing on the detection of occult distal radius fractures, requiring CT imaging for detection. In this study, the authors developed a DL model, Deep Wrist, and evaluated its performance in challenging cases[49]. To validate the model’s efficacy, it was initially tested on a general population test set, where it achieved a diagnostic accuracy of 99% and an AUC of 0.99. However, when tested on the occult fracture dataset, the model’s accuracy dropped to 64%. The model performed slightly better when both lateral and AP views were used together, compared to using the lateral view alone.
Reducing X-ray projections
Building upon the established efficacy of AI in detecting fractures on radiographs, Janisch et al. explored CNN’s potential to reduce the standard X-ray requirements for diagnosing torus fractures of the distal radius[50]. Currently, common practice often requires at least two complementary projections, AP and lateral views of the wrist. This traditional approach, while thorough, results in increased radiation exposure and patient discomfort. Three CNNs were trained on a pediatric dataset and achieved AUCs ranging from 0.945 to 0.980. EfficientNet-B4 emerged as the most accurate, outperforming radiologists and pediatric surgeons.
Applications in pediatric
Zech et al. have made significant contributions to the application of DL models in pediatric fracture detection, publishing three articles. The first study analyzed the performance of an open-source AI algorithm in the detection of pediatric upper extremity fractures based on 53,896 radiographs[51]. In this study, attendings’ accuracy in detecting fractures improved slightly with AI. In contrast, radiology and pediatric residents showed significant improvement with AI. AI showed its superiority especially in identifying non-obvious fractures (non-displaced or non-angulated). The second study focused on wrist injuries. The Faster R-CNN model accurately identified distal radius fractures with an AUC of 0.92[52]. Additionally, the use of AI significantly improved residents’ fracture detection rates from 69% to 92%. To further enhance the model’s performance, Ilie et al. conducted a subsequent, more comprehensive study utilizing a database of 58,846 upper extremity fracture images[53]. This successfully improved the model’s diagnostic accuracy across various fracture types and anatomical regions, notably by an increase of the AUC to 0.96.
A compilation of published articles focusing on AI-driven fracture detection in the hand or wrist (including the distal radius and ulna, carpal bones, and fingers) is presented in Table 2.
Compilation of published articles focusing on automated fracture detection in the hand or wrist using ML models
Study | Fracture site | Dataset size (view) | ML model used | AUC | Sensitivity | Specificity |
Olczak et al. (2017)[25] | Wrist or hand or ankle | 256,000 | Multiple DL networks (BVLC Reference CaffeNet network, VGG CNN S network, VGG CNN, Network-in-network) | 0.83 | - | - |
Lee et al. (2023)[26] | Distal radius, ulnar styloid or scaphoid | 5,618 (AP, lat, and oblique) | e-CRF, self-developed AI model | Distal radius: 0.903 Ulnar styloid: 0.925 Scaphoid: 0.808 | Distal radius: 0.97 Ulnar styloid:0.98 Scaphoid: 0.87 | Distal radius: 0.83 Ulnar styloid:0.87 Scaphoid: 0.74 |
Lindsey et al. (2018)[27] | Wrist | 34,990 (PA, lat) | Self-developed deep neural network | 0.954 | 0.94 | 0.95 |
Cohen et al. (2023)[28] | Distal radius or ulnar styloid or distal ulna or metacarpal or scaphoid or carpal bone | 1,917 (AP, lat, oblique, specific views of the carpus) | BoneView (Gleamer) DCNN algorithm | - | 0.83 | 0.96 |
Hardalaç et al. (2022)[29] | Distal radius or distal ulna | 542 | WFD-C, deep-learning-based object detection model | 0.864 | - | - |
Alammar et al. (2023)[31] | Humerus or wrist | 10,558 | TL adaption of ImageNet models | Humerus: 0.879 Wrist: 0.856 | Humerus: 0.87 Wrist: 0.89 | Humerus: 0.87 Wrist: 0.93 |
Jacques et al. (2024)[32] | Distal radius or distal ulna or carpal bones or scaphoid or finger | 788 | BoneView (Gleamer) | 0.764 | 0.70 | 0.89 |
Kim and MacKinnon (2018)[33] | Distal radius or distal ulna | 1,489 (lat) | Inception v3 network, DCNNs | 0.954 | 0.90 | 0.88 |
Gan et al. (2019)[34] | Distal radius | 2340 wrist (AP) | Inception-v4 | 0.96 | 0.90 | 0.96 |
Oka et al. (2021)[36] | Distal radius, styloid process of ulna | 1,464 (AP, lat) | VGG16 | Distal radius: 0.99 Ulna: 0.96 | Distal radius: 0.99 Ulna: 0.92 | Distal radius: 0.97 Ulna: 0.90 |
Russe et al. (2024)[37] | Distal radius | 2,856 (AP, lat) | Xception | 0.97 | 0.95 | 0.95 |
Zhang et al. (2023)[38] | Distal radius | 6,536 (AP, lat) | Ensemble model of RetinaNet, Faster RCNN and Cascade RCNN | 0.97 | 0.96 | 0.98 |
Anttila et al. (2023)[39] | Distal radius | 3,785 (PA, lat) | Self-developed DL algorithm developed as standalone MATLAB application | 0.95 | 0.86 | 0.89 |
Mert et al. (2024)[40] | Distal radius | 150 (AP, lat) | ChatGPT 4 | 0.93 | 0.88 | 0.98 |
Ozkaya et al. (2022)[41] | Scaphoid | 390 (AP) | Pre-trained ResNet50 network | 0.84 | 0.76 | 0.92 |
Hendrix et al. (2021)[42] | Scaphoid | 4,229 (AP, PA) | Self-developed fracture detection and segmentation CNN | 0.87 | 0.78 | 0.84 |
Hendrix et al. (2023)[43] | Scaphoid | 19,111 (AP, PA, ulnar-deviated and oblique) | Self-developed fracture detection and segmentation CNN | 0.88 | 0.72 | 0.93 |
Tung et al. (2021)[44] | Scaphoid | 356 | VGG16, VGG19, RN50, RN101, RN152, DN121, DN169, DN201, Inv, ENB0 | RN101: 0.950 DN201: 0.910 | RN101: 0.889 DN201: 0.944 | RN101: 0.889 DN201: 0.861 |
Yang et al. (2022)[45] | Scaphoid | 361 | ResNet | 0.917 | 0.735 | 0.920 |
Langerhuizen et al. (2020)[47] | Scaphoid | 300 (scaphoid series) | Open source pretrained CNN (Visual Geometry Group, Oxford, United Kingdom) | 0.77 | 0.84 | 0.60 |
Yoon et al. (2021)[48] | Scaphoid | 11,838 (PA, scaphoid view) | DCNN based on the EfficientNetB3 architecture | Fracture detection: 0.955 Occult fracture detection: 0.81 | 0.87 | 0.92 |
Raisuddin et al. (2021)[49] | Distal radius | 4,497 (AP, lat) | DeepWrist | General test: 0.990 Occult fractures: 0.84 | General test: 0.97 Occult fractures: 0.60 | General test: 0.87 Occult fractures:0.92 |
Zech et al. (2023)[51]* | Distal radius | 395 (AP) | Faster R-CNN model | 0.92 | 0.88 | 0.89 |
Ilie et al. (2023)[53] | Finger, hand, wrist, forearm, elbow, humerus, shoulder, clavicule | 58,846 | Faster R-CNN | 0.96 | 0.91 | 0.89 |
Watanabe et al. (2019)[54] | Distal radius or distal ulna | 7,356 (PA, lat) | Inception-ResNet Faster R-CNN | 0.918 (PA) 0.933 (lat) | 0.957 (PA) 0.967 (lat) | 0.825 (PA) 0.864 (lat) |
Orji et al. (2022)[55] | Finger | 8,170 | ComDNet-512 (deep neural network-based hybrid model) | 0.894 | 0.94 | 0.85 |
AI as an adjuvant in ultrasound fracture detection
While X-rays remain the gold standard for diagnosing distal radius fractures, the use of ultrasound (US) in emergency departments (ED) has gained popularity due to its accessibility, minimal training requirements, and capacity to assess surrounding soft tissues.
Zhang et al. were the first to explore the potential of US for fracture detection, aiming to reduce unnecessary radiation exposure for children without fractures[56]. In their study, they used a three-dimensional ultrasound (3DUS) as a diagnosis tool for patients presenting with wrist tenderness before undergoing X-rays. The findings demonstrated that 3DUS had a diagnostic accuracy of 96.5% for distal radius fractures, establishing it as a reliable method for fracture detection in a pediatric setting. Moreover, the CNN model trained to interpret US images detected all fractures with 100% sensitivity and 87% specificity, matching the sensitivity of the pediatric MSK radiologist.
In 2023, Knight et al. also assessed the diagnostic accuracy of 3DUS for the detection of distal radius fractures while also comparing it to two-dimensional ultrasound (2DUS)[57]. AI models, ResNet34 and Densenet121, were trained on 16,865 images for 2DUS and 15,882 images for 3DUS. Densenet121 had a higher accuracy than ResNet34 with 2DUS (0.94 vs. 0.89), while ResNet34 achieved perfect accuracy with 3DUS (1.00), compared to 0.94 for DenseNet121. Overall, AI’s ability to accurately read images was demonstrated, performing comparably to experts in the field with over a decade of experience.
AI-assisted OA diagnosis and management
In Caratsch et al.’s study, an automated ML model was used for distal hand osteoarthritis (DIP-OA) detection and classification on radiographs[58]. The ML platform used for this study was Giotto [learn to forecast (L2F)], which achieved an overall accuracy of 75%, but its precision decreased for higher grades of OA. Similarly, Overgaard et al. used a CNN-based model (U-Net++) to assess OA severity according to the EULAR-OMERACT grading system (EOGS)[59]. The AI model achieved strong agreement with expert judgments, slightly outperforming previous studies. Moreover, this model provided visual explanations by marking bone (red), synovium (blue), and osteophytes (pink) on the images, aiding clinicians in understanding how the AI arrived at its assessments.
Loos et al. published an article exploring the potential of AI in predicting pain and hand function improvement one year post thumb carpometacarpal OA surgery[60]. Among the models used, the random forest model showed superior performance in predicting pain outcomes using 27 variables, but it still produced a relatively poor AUC of 0.59. On the other hand, gradient boosting machine (GBM) outperformed other models in predicting hand function outcomes, achieving an AUC of 0.74.
CTS diagnosis and management
CTS, the most prevalent compressive mononeuropathy, significantly impacts patients’ quality of life. This justifies hand surgeons’ exploration of AI applications to enhance the diagnostic accuracy and management of this condition which frequently requires surgical decompression to improve symptoms.
Symptoms, physical examination and electromyography (EMG) remain the gold standard for CTS diagnosis and severity assessment. Nevertheless, no widely used screening test has been implemented. In 2021, Watanabe et al. explored the accuracy of an application designed for CTS screening[54]. Their app requires users to draw spirals using a stylus while a pretrained algorithm analyzes the trajectory and the pressure applied during the drawing. The application achieved a sensitivity of 82% and a specificity of 71% in diagnosing CTS, which was inferior to other previously developed apps.
Koyama et al. also developed an application programmed with an anomaly detection algorithm to screen for CTS based on patients’ difficulty with thumb opposition[61]. The app, available for download on smartphones, was able to diagnose CTS, achieving an AUC of 0.86, demonstrating a performance comparable to traditional physical examination methods. To enhance the model’s accuracy, the data were subsequently modified to focus only on the directions that corresponded with thumb opposition.
US is widely used for the diagnosis of CTS. Faeghi et al. explored the diagnostic accuracy of a computer-aided diagnosis (CAD) system developed using radiomics features extracted from US images of the median nerve[62]. The CAD system outperformed both radiologists in this study by achieving an AUC of 0.926. Shinohara et al. also investigated the role of DL in diagnosing CTS using US images[63]. The primary focus of their study was to bypass the traditional method of measuring the median nerve’s cross-sectional area (CSA). The authors applied transfer learning to three pretrained AI models. The algorithm achieved an accuracy of 0.96, thereby demonstrating its potential to detect CTS without relying on CSA measurements. Despite these promising outcomes, the study faced limitations due to the lack of external validation and the relatively small dataset. To address these limitations, Mohammadi et al. conducted a similar study to that of Faeghi et al., incorporating a larger dataset of 416 median nerves extracted from two countries, Iran and Colombia, which was used to train and evaluate multiple DL models[62,64]. The highest-performing algorithm achieved an AUC of 0.910 in the internal validation test and an AUC of 0.890 in the external validation test.
In 2023, Kim et al. also published on this topic. Their study compared ML analysis to conventional quantitative grayscale analysis of US images for diagnosing CTS[65]. The conventional quantitative analysis evaluated the mean echo intensity (EI) by calculating mean thenar EI/mean hypothenar EI ratio. Their findings indicate that hands affected by CTS had a higher EI ratio. However, this method had poor performance metrics, achieving an AUC of 0.755. In contrast, the ML model significantly outperformed the conventional method, achieving an AUC of 0.89.
Similarly, Kuroiwa et al. investigated the role of DL in US diagnosis of CTS[66]. Their study introduced an innovative approach that focuses on measuring the volume of the median nerve to diagnose CTS on US images in contrast to CSA measurement. The DL prediction model used achieved a Dice score of 0.80, which is highly comparable to the manual tracing, which had a Dice score of 0.76. Additionally, compared to a human reader, the DL model achieved a 0.99 accuracy rate with an AUC of 0.91 with the test data set.
Electrophysiological nerve conduction studies (NCS) have long been the gold standard in diagnosing and classifying CTS. Tsamis et al. explored different AI models’ ability to automatically classify and accurately diagnose this condition[67]. Five ML models were trained with common electrodiagnostic features, as well as additional physiological and mathematical characteristics. Support vector machine (SVM) achieved the highest accuracy rate and demonstrated its superiority when classifying disease severity, outperforming both NSC and clinical diagnosis.
Bakalis et al. conducted a study comparing AI’s role in diagnosing CTS through motor versus sensory nerve conduction approaches[68]. For the motor approach, various CNNs were employed to analyze motor signals recorded from the participants’ median nerve and to subsequently classify subjects into patients or controls. CONV2D outperformed other CNNs, achieving an overall accuracy rate of 94%. In the sensory approach, the RF model excelled with a 97.12% accuracy in diagnosing the severity of CTS and excluding other mononeuropathies, making it the top performer in this section.
In 2023, Elseddik et al. published a study focusing on the development of a ML model designed to determine CTS severity[69]. The proposed model demonstrated its ability to accurately diagnose CTS and classify its severity, even when presented with data from other conditions with overlapping symptoms. Additionally, the AI model was able to precisely predict patient improvement probability following median nerve hydrodissection, making it a potentially useful tool for preoperative patient expectation management. Similarly, Park et al. conducted a study to assess AI’s efficacy in classifying the severity of CTS using personal, clinical, and imaging features[70]. All the models in the study had an overall accuracy rate of over 70%.
In 2022, Harrison et al. explored AI’s ability to predict which patients would benefit most from carpal tunnel decompression (CTD)[71]. The highest-performing model for predicting functional and symptomatic improvement was Extreme Gradient Boosting (XGBoost), which achieved an accuracy rate of 71.8% and 75.9% for functional and symptomatic improvement, respectively. Hoogendam et al. also focused on developing a ML model to predict symptom improvement following CTD[72]. GBM was the highest-performing model, achieving an AUC of 0.723.
Loos et al. compared the ability of hand surgeons to predict symptom improvement after CTD with that of AI models[73]. The hand surgeons achieved an accuracy rate of 0.65 with an AUC of 0.62. In contrast, the AI prediction model achieved a higher accuracy rate of 0.78 with an AUC of 0.77. It is important to note that the AI prediction model had access to several patient-reported outcome measures to complete its task. This information is not routinely compiled by hand surgeons, which could contribute to the difference in performance noted between the two groups.
AI-assisted surgery
AI as an adjunctive in wrist arthroscopy
Orgiu et al. developed an AI algorithm to help identify carpal bone structures during wrist arthroscopy[74]. The researchers collected and labeled images from 20 procedures to train and test a DeepLabv3+ classification algorithm. Their model achieved an average Dice score of 89%, indicating that it can effectively assist in identifying carpal bone structures during wrist arthroscopy. Nevertheless, the algorithm’s performance varied among different bones, with some such as the capitate and triquetrum achieving high accuracy rates, while others including the scaphoid and lunate showed moderate results.
Robotics in microsurgery
Henn et al. provide an overview of the current status, advancements, challenges, and future prospects of robotic surgery in plastic surgery[75]. The da Vinci surgical system is highlighted as the most popular platform, widely used across multiple surgical disciplines for its articulated robotic arms and enhanced imaging capabilities. Specific applications in plastic and hand surgery include automated or assisted microvascular anastomosis. Despite its benefits, there are several limitations to the adoption of robotic surgery, including its high initial costs and the need for specialized training for its effective use, as well as the ethical and legal concerns regarding accountability and patient safety.
Integrating AI in hand and wrist surgery training
In 2023, Mohapatra et al. explored the role of AI, specifically LLM, such as ChatGPT, in the training of plastic surgery residents[76]. The authors identified several teaching assistant (TA) tasks that LLMs can perform, including generating interactive case studies, simulating preoperative consultations, and formulating ethical considerations. ChatGPT was found to be capable of assisting faculty with classroom instructions, grading papers, and providing feedback on assignments. Clarity and usefulness constituted AI’s biggest strengths, particularly in simulating preoperative consultations. However, when analyzing ChatGPT’s ability to provide step-by-step guidance for procedures such as microsurgical arterial anastomosis, evaluators noted that while AI provided accurate steps, it omitted some critical components and generated certain inaccurate statements, potentially leading to resident confusion.
AI-assisted patient education
Our review identified five articles focused on the use of AI for patient communication purposes. Jagiella-Lodise et al. evaluated the widest variety of conditions, including CTS, Dupuytren contracture, De Quervain tenosynovitis, trigger finger and metacarpal arthritis[77]. The authors looked to evaluate the accuracy and the completeness of answers provided by ChatGPT 3.5 when questioned on symptoms, pathology, management, surgical indications, recovery time, insurance coverage, and worker’s compensation availability. Their findings suggest that ChatGPT’s overall answers were adequate, but not complete, which led to a lack of comprehension. Amen et al. also tasked ChatGPT with answering common questions asked by patients suffering from CTS[78]. The authors concluded that ChatGPT provided overall reliable and easily understandable answers for patients. Moreover, the algorithm did not provide any patient-specific advice, but instead directed individuals to consult healthcare professionals. Nevertheless, some AI recommendations lacked evidence, and the “black box” concern, which refers to the lack of transparency regarding the source of the information generated by AI, remains a challenge.
In 2023, Croen et al. compared ChatGPT 3.5’s answers to those of Google Web Search regarding frequently asked questions about CTD[79]. Although ChatGPT’s answers were more detailed and were based on multiple academic sources, they were significantly more difficult to understand. Pohl et al. highlighted similar results when comparing ChatGPT’s answers to MedMD and Mayo Clinic regarding various types of hand surgeries including CTD[80]. Similarly, when asked to provide answers at a fourth-grade reading level, ChatGPT generated answers at an average of a tenth-grade reading level. Browne et al. also found that ChatGPT-4 reduced the reading level of information related to hand procedures by a mean of two grade levels, reaching a sixth-grade reading level[81].
Other diagnostic applications
Avascular necrosis detection
Avascular necrosis (AVN) of the lunate is a rare and potentially asymptomatic condition, but its delayed diagnosis and treatment can lead to decreased hand function. To address this, Wernér et al. conducted a study investigating a DL model’s potential to diagnose AVN of the lunate using radiographs[82]. A DL model was developed by the authors within the AI environment Aiforia Create (version 5.5) and was trained to detect AVN of the lunate. The model achieved an AUC of 0.94 and accurately detected AVN in 28 out of 30 cases. The model was outperformed by a hand surgeon and a radiologist but demonstrated significant screening potential.
TFCC injuries prediction
Visualizing the TFCC remains a significant challenge in hand surgery. In 2022, Lin et al. explored the potential of DL for predicting TFCC injuries based on magnetic resonance imaging (MRI) scans[83]. Two CNNs, MRNet and ResNet50, were trained and tasked with detecting the presence of TFCC injuries. ResNet50 significantly outperformed MRNet and both radiologists.
Enchondroma diagnosis
Enchondromas are common benign bone masses that can cause pain and edema in the hand. Their presence also increases the risk of bone fractures. In 2023, Anttila et al. investigated the capability of DL to detect enchondroma on hand radiographs[84]. The DL model achieved an AUC of 0.95, with a diagnosis accuracy of 0.93, but was slightly outperformed by all three clinical experts.
Ganglion cysts identification
Ganglion cysts, commonly found in the hand and wrist, present a diagnostic challenge as they are often hypoechoic. To address this issue, Kim et al. explored the potential application of AI models for diagnosing this condition[85]. The authors developed a DL model composed of two sequential algorithms, which achieved a diagnostic accuracy of 75.43%. The results also indicate that the two-step process enhances the model’s performance and reduces false positive rates, thereby improving the diagnostic accuracy of small hypoechoic ganglion cysts.
Carpal instability identification
Carpal instability frequently occurs as a consequence of acute trauma, such as scaphoid or distal radius fractures, often due to tears in the scapholunate (SL) ligament. Therefore, early identification of carpal instability is crucial to avoid deterioration of this condition. Nevertheless, signs of carpal instability are often unnoticed on conventional radiographs[86]. In response to this diagnostic challenge, Hendrix et al. developed an AI model to identify and assess signs of carpal instability on X-rays[87]. The model demonstrated mean absolute errors (MAE) of 0.65 mm in measuring SL distances, 7.9 degrees for SL angles, and 5.9 degrees for capitolunate angles. Furthermore, the algorithm achieved an AUC of 0.87 for carpal arc interruption detection, outperforming four out of five experts.
Peripheral nerve injuries
Gu et al. conducted a study focusing on the remote screening of peripheral nerve injuries[88]. Three gestures, each corresponding to a specific nerve, were developed by an expert in the field to detect functional abnormalities caused by radial, ulnar, or median nerve injury. The authors trained multiple algorithms, all of which achieved an accuracy rate above 95% for all three gestures, demonstrating their efficacy in detecting abnormalities in the radial, ulnar, and median nerves.
Prolonged postoperative opioid use prediction
It is well known that opioid use is common after hand surgery. To address this, Baxter et al. conducted a study exploring the potential of AI in predicting prolonged opioid use post-hand surgery[89]. Their results indicate that AI, with further training, can potentially be used to identify patients at risk of prolonged opioid use, with one of the models achieving an AUC of 0.84.
AI LIMITATIONS
Despite the rapid advancement in the field of AI and its promising performance in controlled settings, several challenges must be addressed before its full integration into hand surgery. One significant limitation of many studies examining AI’s role in fracture detection is the lack of validation using external datasets, often due to the small size and homogeneity of the samples. This limitation arises from data privacy and the absence of large, labeled datasets across multiple institutions, as well as the need for expert labeling in supervised learning[33]. Additionally, methodological variations, concerns about applicability, risks of bias, and differences in diagnostic protocols between centers further complicate the integration of AI into clinical practice, highlighting the necessity of standardized guidelines to ensure the quality and reliability of AI-driven models and provide structured and consistent methodologies[46]. Moreover, most existing studies are retrospective, which, while useful for demonstrating proof of concept, fall short of establishing the robust evidence required for clinical application. Therefore, prospective studies are needed to confirm the performance metrics and to demonstrate AI’s potential to enhance patient management and outcomes.
Moreover, while LLMs have shown their potential in accessing, interpreting, and synthesizing extensive amounts of information, they still struggle with complex cases that require the integration of nuanced clinical contexts. Despite advancements in newer versions, these models are not yet fully equipped to meet the clinical needs of patients and healthcare providers. Similarly, to ensure the effective integration of AI models into academic contexts, there is a need for further training to enhance the validity of AI-generated content and to improve the transparency of the sources from which this information is derived.
Finally, it is also essential to acknowledge that at this stage of its evolution, AI does not possess the capability to replace humans. Numerous ethical and liability concerns must be thoroughly examined before such a possibility can even be considered. As AI technology continues to advance, it is crucial to develop clear guidelines and establish a robust regulatory framework in parallel, ensuring that these innovations are integrated responsibly and ethically.
SIMILAR WORK
We would like to highlight previous studies conducted on the usefulness of AI in the field of hand and wrist surgery. Firstly, a 2023 article published by Miller et al. aimed to educate hand surgeons on AI, its current applications, and its potential integration into their practice[90]. This paper explored various roles of AI, including fracture detection, decision-making processes, and outcome prediction. The authors described AI’s potential to enhance diagnostic accuracy, facilitate orthopedic surgery referrals, and aid in scan assessments. Similarly, our review offers a more comprehensive analysis of AI’s potential in detecting a wider range of hand and wrist fractures including distal radius and ulna fractures using various imaging techniques such as US and CT scans. In addition, AI applications in pediatric settings were also described in our review.
Keller et al.’s literature review is the only one, to our knowledge, that specifically explored the application of AI in hand surgery[91]. Their primary search identified 435 articles, with 235 ultimately included in their final analysis. Their findings were categorized based on the roles of AI, which included automated image analysis of anatomic structures, fracture detection, various other applications, and those loosely related to hand surgery. This paper was essentially intended for hand surgeons and therapists, as it also explored aspects of hand rehabilitation. Considering the rapid evolution of AI and the fact that Keller et al.’s review was conducted in July 2021, our review aims to update surgeons on the innovations and advancements made since then. Moreover, our review was conducted from an academic plastic surgery perspective, which justifies the inclusion of AI’s role in education and patient communication, microsurgery, and a deep dive into CTS. Therefore, our work builds on Keller et al.’s foundation but also expands the scope.
In addition, the literature includes other systematic reviews solely focused on the evaluation of AI in fracture detection. For instance, Kraus et al., in 2023, identified ten studies on AI’s performance in detecting scaphoid fractures using X-rays[92]. Our review identified and analyzed nine of these ten studies, using performance metrics such as AUCs. Similarly, reviews by Oeding et al. and Singh et al. focused on AI’s effectiveness in scaphoid fracture detection, yielding results consistent with our findings[93,94].
Nevertheless, this study faced some limitations. First, determining what type of articles to include was challenging due to how vast the field of hand surgery is and the rapid pace of AI advancements. This was addressed by exclusively including articles of clinical relevance to hand surgeons, which justifies the exclusions of certain articles such as those related to rehabilitation and prosthetics for arms and hands. Various combinations of keywords were used in searches on databases before identifying the most effective keywords to identify the largest number of pertinent articles.
Second, the decision to only include articles published in the last decade narrows the scope of this review regarding earlier contributions. Most articles covered in this review were published in 2023 and 2024, with the inclusion of only one article each from 2017 and 2018. Nevertheless, the objective of this study was to highlight the latest innovations in AI within hand and wrist surgery. Therefore, this limitation may impact readers’ understanding of AI’s full evolution, as earlier milestones are shallowly addressed.
Third, to cover as many aspects of AI applications in hand surgery as possible, a lot of topics were included in this paper. However, we recognize that conducting systematic reviews on each subject separately would result in more extensive coverage. This is a potential avenue for future work, where more focused reviews could be conducted.
CONCLUSION
The integration of AI into medicine marks the beginning of a transformative phase for this disciple. Despite its current limited use in daily clinical practice, particularly in hand surgery, it is undeniable that AI holds significant potential to revolutionize the field in the coming years. This review highlights the evolution and expansion of diverse AI technologies. Nevertheless, further research is imperative to explore the practical advantages of AI in clinical settings. In particular, expanding and diversifying the training datasets for AI models to include a wider range of patient demographics and imaging modalities is crucial. This area presents a promising avenue for future work, where more targeted studies could provide deeper insights.
DECLARATIONS
Authors’ contributions
Conception and design of this study, initiated the literature search, conducted the initial title and abstract screening, screening the full text, writing and editing the manuscript: Dababneh S, Efanov JI
Initial title and abstract screening, writing and editing the manuscript: Colivas J, Dababneh N
Availability of data and materials
Not applicable.
Financial support and sponsorship
None.
Conflicts of interest
All authors declared that there are no conflicts of interest.
Ethical approval and consent to participate
Not applicable.
Consent for publication
Not applicable.
Copyright
© The Author(s) 2024.
REFERENCES
1. Mintz Y, Brodie R. Introduction to artificial intelligence in medicine. Minim Invasive Ther Allied Technol 2019;28:73-81.
2. Jarvis T, Thornburg D, Rebecca AM, Teven CM. Artificial intelligence in plastic surgery: current applications, future directions, and ethical implications. Plast Reconstr Surg Glob Open 2020;8:e3200.
3. Mantelakis A, Assael Y, Sorooshian P, Khajuria A. Machine learning demonstrates high accuracy for disease diagnosis and prognosis in plastic surgery. Plast Reconstr Surg Glob Open 2021;9:e3638.
4. Lopez C, Tucker S, Salameh T, Tucker C. An unsupervised machine learning method for discovering patient clusters based on genetic signatures. J Biomed Inform 2018;85:30-9.
5. Jiang F, Jiang Y, Zhi H, et al. Artificial intelligence in healthcare: past, present and future. Stroke Vasc Neurol 2017;2:230-43.
6. Ahmadi N, Niazmand M, Ghasemi A, Mohaghegh S, Motamedian SR. Applications of machine learning in facial cosmetic surgeries: a scoping review. Aesthetic Plast Surg 2023;47:1377-93.
7. Lakhani P, Prater AB, Hutson RK, et al. Machine learning in radiology: applications beyond image interpretation. J Am Coll Radiol 2018;15:350-9.
8. Pesapane F, Codari M, Sardanelli F. Artificial intelligence in medical imaging: threat or opportunity? Radiologists again at the forefront of innovation in medicine. Eur Radiol Exp 2018;2:35.
9. Zhou XY, Guo Y, Shen M, Yang GZ. Application of artificial intelligence in surgery. Front Med 2020;14:417-30.
10. Loftus TJ, Tighe PJ, Filiberto AC, et al. Artificial intelligence and surgical decision-making. JAMA Surg 2020;155:148-58.
11. Spoer DL, Kiene JM, Dekker PK, et al. A systematic review of artificial intelligence applications in plastic surgery: looking to the future. Plast Reconstr Surg Glob Open 2022;10:e4608.
12. Dorfman R, Chang I, Saadat S, Roostaeian J. Making the subjective objective: machine learning and rhinoplasty. Aesthet Surg J 2020;40:493-8.
13. Eldaly AS, Avila FR, Torres-Guzman RA, et al. Simulation and artificial intelligence in rhinoplasty: a systematic review. Aesthetic Plast Surg 2022;46:2368-77.
14. Kanevsky J, Corban J, Gaster R, Kanevsky A, Lin S, Gilardino M. Big data and machine learning in plastic surgery: a new frontier in surgical innovation. Plast Reconstr Surg 2016;137:890e-7e.
15. Tricco AC, Lillie E, Zarin W, et al. PRISMA extension for scoping reviews (PRISMA-ScR): checklist and explanation. Ann Intern Med 2018;169:467-73.
16. Thibaut G, Dabbagh A, Liverneaux P. Does Google’s Bard Chatbot perform better than ChatGPT on the European hand surgery exam? Int Orthop 2024;48:151-8.
17. Arango SD, Flynn JC, Zeitlin J, et al. The performance of ChatGPT on the American society for surgery of the hand self-assessment examination. Cureus 2024;16:e58950.
18. Ghanem D, Nassar JE, El Bachour J, Hanna T. ChatGPT earns American Board Certification in Hand Surgery. Hand Surg Rehabil 2024;43:101688.
19. Crook BS, Park CN, Hurley ET, Richard MJ, Pidgeon TS. Evaluation of online artificial intelligence-generated information on common hand procedures. J Hand Surg Am 2023;48:1122-7.
20. Seth I, Sinkjær Kenney P, Bulloch G, Hunter-Smith DJ, Bo Thomsen J, Rozen WM. Artificial or augmented authorship? A conversation with a chatbot on base of thumb arthritis. Plast Reconstr Surg Glob Open 2023;11:e4999.
21. Leypold T, Schäfer B, Boos A, Beier JP. Can AI think like a plastic surgeon? Evaluating GPT-4’s clinical judgment in reconstructive procedures of the upper extremity. Plast Reconstr Surg Glob Open 2023;11:e5471.
22. Seth I, Xie Y, Rodwell A, et al. Exploring the role of a large language model on carpal tunnel syndrome management: an observation study of ChatGPT. J Hand Surg Am 2023;48:1025-33.
23. Seth I, Lim B, Xie Y, Hunter-Smith DJ, Rozen WM. Exploring the role of artificial intelligence chatbot on the management of scaphoid fractures. J Hand Surg Eur Vol 2023;48:814-8.
24. Ajmera P, Nischal N, Ariyaratne S, et al. Validity of ChatGPT-generated musculoskeletal images. Skeletal Radiol 2024;53:1583-93.
25. Olczak J, Fahlberg N, Maki A, et al. Artificial intelligence for analyzing orthopedic trauma radiographs. Acta Orthop 2017;88:581-6.
26. Lee KC, Choi IC, Kang CH, et al. Clinical validation of an artificial intelligence model for detecting distal radius, ulnar styloid, and scaphoid fractures on conventional wrist radiographs. Diagnostics 2023;13:1657.
27. Lindsey R, Daluiski A, Chopra S, et al. Deep neural network improves fracture detection by clinicians. Proc Natl Acad Sci U S A 2018;115:11591-6.
28. Cohen M, Puntonet J, Sanchez J, et al. Artificial intelligence vs. radiologist: accuracy of wrist fracture detection on radiographs. Eur Radiol 2023;33:3974-83.
29. Hardalaç F, Uysal F, Peker O, et al. Fracture detection in wrist x-ray images using deep learning-based object detection models. Sensors 2022;22:1285.
30. Lysdahlgaard S. Utilizing heat maps as explainable artificial intelligence for detecting abnormalities on wrist and elbow radiographs. Radiography 2023;29:1132-8.
31. Alammar Z, Alzubaidi L, Zhang J, Li Y, Lafta W, Gu Y. Deep transfer learning with enhanced feature fusion for detection of abnormalities in X-ray images. Cancers 2023;15:4007.
32. Jacques T, Cardot N, Ventre J, Demondion X, Cotten A. Commercially-available AI algorithm improves radiologists’ sensitivity for wrist and hand fracture detection on X-ray, compared to a CT-based ground truth. Eur Radiol 2024;34:2885-94.
33. Kim DH, MacKinnon T. Artificial intelligence in fracture detection: transfer learning from deep convolutional neural networks. Clin Radiol 2018;73:439-45.
34. Gan K, Xu D, Lin Y, et al. Artificial intelligence detection of distal radius fractures: a comparison between the convolutional neural network and professional assessments. Acta Orthop 2019;90:394-400.
35. Thian YL, Li Y, Jagmohan P, Sia D, Chan VEY, Tan RT. Convolutional neural networks for automated fracture detection and localization on wrist radiographs. Radiol Artif Intell 2019;1:e180001.
36. Oka K, Shiode R, Yoshii Y, Tanaka H, Iwahashi T, Murase T. Artificial intelligence to diagnosis distal radius fracture using biplane plain X-rays. J Orthop Surg Res 2021;16:694.
37. Russe MF, Rebmann P, Tran PH, et al. AI-based X-ray fracture analysis of the distal radius: accuracy between representative classification, detection and segmentation deep learning models for clinical practice. BMJ Open 2024;14:e076954.
38. Zhang J, Li Z, Lin H, et al. Deep learning assisted diagnosis system: improving the diagnostic accuracy of distal radius fractures. Front Med 2023;10:1224489.
39. Anttila TT, Karjalainen TV, Mäkelä TO, et al. Detecting distal radius fractures using a segmentation-based deep learning model. J Digit Imaging 2023;36:679-87.
40. Mert S, Stoerzer P, Brauer J, et al. Diagnostic power of ChatGPT 4 in distal radius fracture detection through wrist radiographs. Arch Orthop Trauma Surg 2024;144:2461-7.
41. Ozkaya E, Topal FE, Bulut T, Gursoy M, Ozuysal M, Karakaya Z. Evaluation of an artificial intelligence system for diagnosing scaphoid fracture on direct radiography. Eur J Trauma Emerg Surg 2022;48:585-92.
42. Hendrix N, Scholten E, Vernhout B, et al. Development and validation of a convolutional neural network for automated detection of scaphoid fractures on conventional radiographs. Radiol Artif Intell 2021;3:e200260.
43. Hendrix N, Hendrix W, van Dijke K, et al. Musculoskeletal radiologist-level performance by using deep learning for detection of scaphoid fractures on conventional multi-view radiographs of hand and wrist. Eur Radiol 2023;33:1575-88.
44. Tung Y, Su J, Liao Y, et al. High-performance scaphoid fracture recognition via effectiveness assessment of artificial neural networks. Appl Sci 2021;11:8485.
45. Yang TH, Horng MH, Li RS, Sun YN. Scaphoid fracture detection by using convolutional neural network. Diagnostics 2022;12:895.
46. Bulstra AEJ; Machine Learning Consortium. A machine learning algorithm to estimate the probability of a true scaphoid fracture after wrist trauma. J Hand Surg Am 2022;47:709-18.
47. Langerhuizen DWG, Bulstra AEJ, Janssen SJ, et al. Is deep learning on par with human observers for detection of radiographically visible and occult fractures of the scaphoid? Clin Orthop Relat Res 2020;478:2653-9.
48. Yoon AP, Lee YL, Kane RL, Kuo CF, Lin C, Chung KC. Development and validation of a deep learning model using convolutional neural networks to identify scaphoid fractures in radiographs. JAMA Netw Open 2021;4:e216096.
49. Raisuddin AM, Vaattovaara E, Nevalainen M, et al. Critical evaluation of deep neural networks for wrist fracture detection. Sci Rep 2021;11:6006.
50. Janisch M, Apfaltrer G, Hržić F, et al. Pediatric radius torus fractures in x-rays - how computer vision could render lateral projections obsolete. Front Pediatr 2022;10:1005099.
51. Zech JR, Ezuma CO, Patel S, et al. Artificial intelligence improves resident detection of pediatric and young adult upper extremity fractures. Skeletal Radiol 2024.
52. Smith AM, Forder JA, Annapureddy SR, Reddy KSK, Amis AA. The porcine forelimb as a model for human flexor tendon surgery. J Hand Surg Br 2005;30:307-9.
53. Ilie VG, Ilie VI, Dobreanu C, Ghetu N, Luchian S, Pieptu D. Training of microsurgical skills on nonliving models. Microsurgery 2008;28:571-7.
54. Watanabe T, Koyama T, Yamada E, Nimura A, Fujita K, Sugiura Y. The accuracy of a screening system for carpal tunnel syndrome using hand drawing. J Clin Med 2021;10:4437.
55. Orji C, Reghefaoui M, Saavedra Palacios MS, et al. Application of artificial intelligence and machine learning in diagnosing scaphoid fractures: a systematic review. Cureus 2023;15:e47732.
56. Zhang J, Boora N, Melendez S, Rakkunedeth Hareendranathan A, Jaremko J. Diagnostic accuracy of 3D ultrasound and artificial intelligence for detection of pediatric wrist injuries. Children 2021;8:431.
57. Knight J, Zhou Y, Keen C, et al. 2D/3D ultrasound diagnosis of pediatric distal radius fractures by human readers vs artificial intelligence. Sci Rep 2023;13:14535.
58. Caratsch L, Lechtenboehmer C, Caorsi M, et al. Detection and grading of radiographic hand osteoarthritis using an automated machine learning platform. ACR Open Rheumatol 2024;6:388-95.
59. Overgaard BS, Christensen ABH, Terslev L, Savarimuthu TR, Just SA. Artificial intelligence model for segmentation and severity scoring of osteophytes in hand osteoarthritis on ultrasound images. Front Med 2024;11:1297088.
60. Loos NL, Hoogendam L, Souer JS, et al; the Hand-Wrist Study Group. Machine learning can be used to predict function but not pain after surgery for thumb carpometacarpal osteoarthritis. Clin Orthop Relat Res 2022;480:1271-84.
61. Koyama T, Sato S, Toriumi M, et al. A screening method using anomaly detection on a smartphone for patients with carpal tunnel syndrome: diagnostic case-control study. JMIR Mhealth Uhealth 2021;9:e26320.
62. Faeghi F, Ardakani AA, Acharya UR, et al. Accurate automated diagnosis of carpal tunnel syndrome using radiomics features with ultrasound images: a comparison with radiologists’ assessment. Eur J Radiol 2021;136:109518.
63. Shinohara I, Inui A, Mifune Y, et al. Using deep learning for ultrasound images to diagnose carpal tunnel syndrome with high accuracy. Ultrasound Med Biol 2022;48:2052-9.
64. Mohammadi A, Torres-Cuenca T, Mirza-Aghazadeh-Attari M, Faeghi F, Acharya UR, Abbasian Ardakani A. Deep radiomics features of median nerves for automated diagnosis of carpal tunnel syndrome with ultrasound images: a multi-center study. J Ultrasound Med 2023;42:2257-68.
65. Kim SW, Kim S, Shin D, et al. Feasibility of artificial intelligence assisted quantitative muscle ultrasound in carpal tunnel syndrome. BMC Musculoskelet Disord 2023;24:524.
66. Kuroiwa T, Jagtap J, Starlinger J, et al. Deep learning estimation of median nerve volume using ultrasound imaging in a human cadaver model. Ultrasound Med Biol 2022;48:2237-48.
67. Tsamis KI, Kontogiannis P, Gourgiotis I, Ntabos S, Sarmas I, Manis G. Automatic electrodiagnosis of carpal tunnel syndrome using machine learning. Bioengineering 2021;8:181.
68. Bakalis D, Kontogiannis P, Ntais E, Simos YV, Tsamis KI, Manis G. Carpal tunnel syndrome automated diagnosis: a motor vs. sensory nerve conduction-based approach. Bioengineering 2024;11:175.
69. Elseddik M, Mostafa RR, Elashry A, et al. Predicting CTS diagnosis and prognosis based on machine learning techniques. Diagnostics 2023;13:492.
70. Park D, Kim BH, Lee SE, et al. Machine learning-based approach for disease severity classification of carpal tunnel syndrome. Sci Rep 2021;11:17464.
71. Harrison CJ, Geoghegan L, Sidey-Gibbons CJ, Stirling PHC, McEachan JE, Rodrigues JN. Developing machine learning algorithms to support patient-centered, value-based carpal tunnel decompression surgery. Plast Reconstr Surg Glob Open 2022;10:e4279.
72. Hoogendam L, Bakx JAC, Souer JS, Slijper HP, Andrinopoulou ER, Selles RW; Hand Wrist Study Group. Predicting clinically relevant patient-reported symptom improvement after carpal tunnel release: a machine learning approach. Neurosurgery 2022;90:106-13.
73. Loos NL, Hoogendam L, Souer JS, et al; Hand-Wrist Study Group. Algorithm versus expert: machine learning versus surgeon-predicted symptom improvement after carpal tunnel release. Neurosurgery 2024;95:110-7.
74. Orgiu A, Karkazan B, Cannell S, Dechaumet L, Bennani Y, Grégory T. Enhancing wrist arthroscopy: artificial intelligence applications for bone structure recognition using machine learning. Hand Surg Rehabil 2024:101717.
75. Henn D, Trotsyuk AA, Barrera JA, et al. Robotics in plastic surgery: it’s here. Plast Reconstr Surg 2023;152:239-49.
76. Mohapatra DP, Thiruvoth FM, Tripathy S, et al. Leveraging large language models (LLM) for the plastic surgery resident training: do they have a role? Indian J Plast Surg 2023;56:413-20.
77. Jagiella-Lodise O, Suh N, Zelenski NA. Can patients rely on ChatGPT to answer hand pathology-related medical questions? Hand 2024:15589447241247246.
78. Amen TB, Torabian KA, Subramanian T, Yang BW, Liimakka A, Fufa D. Quality of ChatGPT responses to frequently asked questions in carpal tunnel release surgery. Plast Reconstr Surg Glob Open 2024;12:e5822.
79. Croen BJ, Abdullah MS, Berns E, et al. Evaluation of patient education materials from large-language artificial intelligence models on carpal tunnel release. Hand 2024:15589447241247332.
80. Pohl NB, Derector E, Rivlin M, et al. A quality and readability comparison of artificial intelligence and popular health website education materials for common hand surgery procedures. Hand Surg Rehabil 2024;43:101723.
81. Browne R, Gull K, Hurley CM, Sugrue RM, O’Sullivan JB. ChatGPT-4 can help hand surgeons communicate better with patients. J Hand Surg Glob Online 2024;6:436-8.
82. Wernér K, Anttila T, Hulkkonen S, Viljakka T, Haapamäki V, Ryhänen J. Detecting avascular necrosis of the lunate from radiographs using a deep-learning model. J Imaging Inform Med 2024;37:706-14.
83. Lin KY, Li YT, Han JY, et al. Deep learning to detect triangular fibrocartilage complex injury in wrist MRI: retrospective study with internal and external validation. J Pers Med 2022;12:1029.
84. Anttila TT, Aspinen S, Pierides G, Haapamäki V, Laitinen MK, Ryhänen J. Enchondroma detection from hand radiographs with an interactive deep learning segmentation tool - a feasibility study. J Clin Med 2023;12:7129.
85. Kim KB, Song DH, Park HJ. Intelligent automatic segmentation of wrist ganglion cysts using DBSCAN and fuzzy C-means. Diagnostics 2021;11:2329.
86. Buul MM, Bos KE, Dijkstra PF, van Beek EJ, Broekhuizen AH. Carpal instability, the missed diagnosis in patients with clinically suspected scaphoid fracture. Injury 1993;24:257-62.
87. N, Hendrix W, Maresch B, et al. Hendrix N, Hendrex W, Maresch B, et al. Artificial intelligence for automated detection and measurements of carpal instability signs on conventional radiographs. Eur Radiol 2024.
88. Gu F, Fan J, Cai C, et al. Automatic detection of abnormal hand gestures in patients with radial, ulnar, or median nerve injury using hand pose estimation. Front Neurol 2022;13:1052505.
89. Baxter NB, Ho AZ, Byrd JN, Fernandez AC, Singh K, Chung KC. Predicting persistent opioid use after hand surgery: a machine learning approach. Plast Reconstr Surg 2024;154:573-80.
90. Miller R, Farnebo S, Horwitz MD. Insights and trends review: artificial intelligence in hand surgery. J Hand Surg Eur Vol 2023;48:396-403.
91. Keller M, Guebeli A, Thieringer F, Honigmann P. Artificial intelligence in patient-specific hand surgery: a scoping review of literature. Int J Comput Assist Radiol Surg 2023;18:1393-403.
92. Kraus M, Anteby R, Konen E, Eshed I, Klang E. Artificial intelligence for X-ray scaphoid fracture detection: a systematic review and diagnostic test accuracy meta-analysis. Eur Radiol 2024;34:4341-51.
93. Oeding JF, Kunze KN, Messer CJ, et al. Diagnostic performance of artificial intelligence for detection of scaphoid and distal radius fractures: a systematic review. J Hand Surg Am 2024;49:411-22.
Cite This Article
How to Cite
Dababneh, S.; Colivas J.; Dababneh N.; Efanov J. I. Artificial intelligence as an adjunctive tool in hand and wrist surgery: a review. Art. Int. Surg. 2024, 4, 214-32. http://dx.doi.org/10.20517/ais.2024.50
Download Citation
Export Citation File:
Type of Import
Tips on Downloading Citation
Citation Manager File Format
Type of Import
Direct Import: When the Direct Import option is selected (the default state), a dialogue box will give you the option to Save or Open the downloaded citation data. Choosing Open will either launch your citation manager or give you a choice of applications with which to use the metadata. The Save option saves the file locally for later use.
Indirect Import: When the Indirect Import option is selected, the metadata is displayed and may be copied and pasted as needed.
About This Article
Special Issue
Copyright
Data & Comments
Data
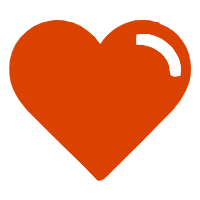
Comments
Comments must be written in English. Spam, offensive content, impersonation, and private information will not be permitted. If any comment is reported and identified as inappropriate content by OAE staff, the comment will be removed without notice. If you have any queries or need any help, please contact us at support@oaepublish.com.