AI in plastic surgery: customizing care for each patient
Abstract
Artificial intelligence (AI) and machine learning (ML) involve the usage of complex algorithms to identify patterns, predict future outcomes, generate new data, and perform other tasks that typically require human intelligence. AI tools have been progressively adopted by multiple disciplines of surgery, enabling increasingly patient-specific care, as well as more precise surgical modeling and assessment. For instance, AI tools such as ChatGPT have been applied to enhance both patient educational materials and patient-surgeon communication. Additionally, AI tools have helped support pre- and postoperative assessment in a diverse set of procedures, including breast reconstructions, facial surgeries, hand surgeries, wound healing operations, and burn surgeries. Further, ML-supported 3D modeling has now been utilized for patient-specific surgical planning and may also be combined with 3D printing technologies to generate patient-customized, implantable constructs. Ultimately, the advent of AI and its intersection with surgical practice have demonstrated immense potential to transform patient care by making multiple facets of the surgical process more efficient, precise, and patient-specific.
Keywords
INTRODUCTION
Artificial intelligence (AI) refers to a class of computer science and engineering technologies that leverage sophisticated algorithms, including machine learning (ML) methods, to perform tasks that typically require human intelligence. Although there are several subtypes of AI, ML has been widely used in the clinical setting and focuses on finding patterns in large datasets and/or performing predictive modeling based on prior training data[1,2]. ML encompasses a plethora of model types, including artificial neural networks (ANN), deep neural networks (DNN), natural language processing (NLP), and computer vision (CV)[3]. Within healthcare, AI tools have demonstrated significant capability across various contexts, including remote patient monitoring, medical diagnostics, risk management, conversation agents, and provision of virtual assistants[1,2]. Therefore, AI is postulated to have the potential to guide the diagnostic process, decrease the likelihood of medical errors, and improve the precision of medical decisions. Additionally, there is growing interest in the ability of ChatGPT, a popular NLP algorithm, to generate patient-facing information[3].
ML applications in healthcare often resemble traditional statistical analysis, although ML algorithms are more adept at handling large, heterogeneous datasets and detecting less strictly formalized relationships between these data[2]. Since the amount of data in electronic health records (EHRs) has recently doubled every two years, AI tools that collect and analyze a number of data unachievable by human power alone will likely bring numerous changes to the medical field[4,5]. For example, one healthcare application of this AI tool is prediction algorithms, applicable in various specialties, where ML is able to provide the probability of outcomes[6]. Therefore, the most successful clinical applications of AI are seen in medical specialties that collect large amounts of standardized data, including image-recognition tasks in dermatology, radiology, pathology, and cardiology, as reflected by the number of Food and Drug Administration (FDA)-approved medical devices across these specialties [Figure 1][7,8]. For instance, in dermatology, ML has demonstrated performance comparable to board-certified dermatologists in the detection of skin lesions from clinical or dermoscopic images[9], as well as recognition of potentially cancerous lesions in radiologic images[10]. In radiology, AI has gained significant prominence, transforming how the specialty is practiced and reducing radiologists’ workloads, particularly by decreasing the time required to interpret X-rays and computed tomography (CT) scans[11-13].
Figure 1. Proportion of FDA-approved AI-enabled medical devices within different medical disciplines, displayed as percentages. FDA: Food and Drug Administration; AI: artificial intelligence.
While many surgical disciplines involve less standardized data compared to imaging-focused medical practices, the field has evolved significantly over the past few years to integrate AI into practice[14]. Specifically, AI can revolutionize plastic surgery by enhancing patient information, patient-surgeon communication, surgical planning, and 3D tissue modeling and printing for surgical applications[14,15]. Therefore, analyzing current applications of AI in surgery is critical to developing novel surgical resources that have the potential to provide patients with the highest-quality healthcare. In this review, we explore how AI has impacted multiple facets of plastic and reconstructive surgery (PRS) and demonstrate ways in which patient-specific care in surgery has been influenced by the adoption of AI tools.
METHODOLOGY
The literature analysis was conducted as a narrative review, utilizing the following databases: Cochrane Library, Embase, Web of Science, and Medline. A search strategy incorporating both MeSH terms and free-text keywords was employed, focusing on the terms “Surgery, Plastic” and “Artificial Intelligence”. The search was limited to articles published within the last 10 years, from 2013 to the present. The objective was to identify all relevant studies that reported on the tailored application of AI in plastic surgery. Articles identified through the search were categorized into three key areas: Patient Preparation and Education, Pre- and Postoperative Assessments, and 3D Tissue Modeling and Printing. Studies that were outside the thematic scope, did not involve the use of computer-based intelligence, or pertained to other surgical or medical fields were excluded. It was conducted in two phases: initially, a screening based on titles and abstracts was performed, followed by a full-text review of the selected articles. Articles that were deemed appropriate underwent a more comprehensive evaluation, while those not meeting the inclusion criteria were excluded.
PATIENT PREPARATION AND EDUCATION
AI increasing patient communication
In PRS, patient-surgeon communication is essential to address expectations of procedures, approach patients’ concerns and goals, and manage patients’ health. Communication between clinicians and patients has a demonstrable impact on patient satisfaction, clinical outcomes, and litigation[16]. However, there is often a discrepancy between surgeons’ level of communication and patient’s level of comprehension. One of the direct applications of AI in transforming medical care is optimizing the creation and delivery of patient information, as well as medical documentation. AI tools, including ChatGPT and artificial intelligence virtual assistants (AIVAs), have recently been utilized to create relevant and tailored medical information to support patients' needs. These NLP systems have proven their ability to increase the readability of medical information, respond to frequently asked surgical questions, and address the benefits and risks of PRS operations[17-24]. By producing patient-relevant information, AI tools can also reduce the need for healthcare workers to respond to frequently asked questions during medical appointments. Additionally, ChatGPT can be utilized 24/7 to address patients’ concerns and promptly provide essential medical information[25]. As a result, chatbots may help decrease the need for in-person medical consultations and increase access to healthcare in rural regions at a reduced cost[26,27].
ChatGPT - enhancing readability of patient education
Readability refers to the ease with which a reader can comprehend written material, with common scoring systems including the Flesch Reading Ease Score, Flesh-Kincaid Grade Level, Gunning Fog Index, Coleman-Liau Index, and Simple Measure of Gobbledygook Index[19,21]. Each scoring system employs a unique mathematical formula to analyze factors such as mean number of sentences, number of syllables per sentence, number of words per sentence, or number of complex words per sentence[19]. Despite the need for readable patient-facing information, multiple studies have demonstrated that the readability level of information for breast reconstruction, burn injuries, hand surgery, and gender-affirming surgery exceeds the sixth-grade readability level recommended by the American Medical Association and National Institute of Health (NIH)[17-19,28]. To increase the readability of online medical material, AI tools such as ChatGPT have recently been evaluated in clinical settings[19,21]. In one study by Wang et al., the researchers performed an online search for “breast reconstruction”[28]. They collected patient information from the top 10 websites based on “hits” and found most of them to exceed the NIH’s readability recommendation[28]. Information provided by the sources was then entered into ChatGPT with the command: “Rephrase this article to a 5th-grade readability level: ‘[Article]’”[28]. Paired t-tests of readability scores for Flesch Reading Ease, Flesch-Kincaid Grade Level, and Simple Measure of Gobbledygook Index were performed, comparing medical information provided by the websites to the same information adjusted by ChatGPT. A value of P < 0.05 indicated statistical significance. Results demonstrated that ChatGPT generally increased the readability of patient-facing PRS information; however, this improvement was statistically significant for only one of the ten websites, specifically “Plasticsurgery.org”[28]. On an important note, readability scores still exceeded 5th-grade levels, highlighting an ongoing need to generate more readable patient-facing information[28]. Despite this current limitation, Wang et al. proved that ChatGPT has the potential to increase the readability of online information and may be utilized by plastic surgeons to help simplify complex online medical material[28]. Similar results were obtained by Baldwin et al., who evaluated ChatGPT’s ability to improve burn first aid information to an 11-year-old literacy level[19]. Baldwin et al. utilized a one-sample one-tailed
Evaluation of ChatGPT-generated patient-facing information
Currently, 80% of Americans use the Internet for medical information, and a new study has determined that 78.4% of patients are open to utilizing ChatGPT for medical diagnoses[20,29,30]. Therefore, ensuring the quality of ChatGPT-generated information for patient safety in an age where people increasingly consult the Internet for healthcare information is critical. In attempts to develop safe and accurate patient-facing information, ChatGPT-generated responses have been evaluated across various divisions of plastic surgery: microsurgery, breast surgery, rhinoplasty, and cleft lip and palate surgery[20-24]. Additionally, to properly determine the quality of ChatGPT-generated information, material currently available from academic and professional sources is often compared against newly created ChatGPT medical information[20-24]. Grading scales and tests frequently utilized by researchers to assess the quality of PRS information generated by ChatGPT against online resources include Likert scales, EQUIP scales, and readability tests[18,19,21]. In one study by Berry et al., ChatGPT-generated responses to frequently asked microsurgery medical questions were compared against information currently provided by the American Society of Reconstructive Microsurgery (ASRM) utilizing paired t-tests[20]. Similar to Wang et al., a value of P < 0.05 indicated statistical significance[28]. Six plastic surgeons were tasked with assessing the comprehensiveness and clarity of the two sources’ responses and selecting the source that provided the highest-quality patient-facing information[20]. Thirty non-medical individuals only indicated their preference. Surprisingly, plastic surgeons scored ChatGPT information significantly higher in terms of comprehensiveness (P < 0.001) and clarity (P < 0.05)[20]. Plastic surgeons and non-medical individuals also chose ChatGPT as the source that provides the highest-quality microsurgical information 70.7% and 55.9% of the time, respectively. Interestingly, the readability scores of ChatGPT responses were considerably worse than ASRM according to the following readability tests: Flesch-Kincaid Grade Level (P < 0.0001), FleschKincaid Readability Ease
Similarly, in a study by Grippaudo et al., ten plastic surgery residents analyzed the quality of ChatGPT-generated breast plastic surgery information utilizing an EQIP scale for the frequently performed procedures: breast reduction, breast reconstruction, and augmentation mammoplasty[21]. The EQUIP scale is made up of 36 yes or no questions with three sections: Content data (Questions 1-18), Identification data (Questions 25-36), and Structure data (Questions 25-36)[21]. Each question has a singular point value and a score above 18 is considered a high score. ChatGPT was proven to create quality breast surgery information. Regarding “Structure data”, ChatGPT thrived in providing clear and comprehensive information for patients. However, one limitation identified by the researchers was that ChatGPT-generated medical information struggled to perform well for the “Identification data” questions, often lacking proper validation or bibliographic references. Despite this limitation, ChatGPT proved to create quality PRS patient-facing information in regard to breast reconstruction, breast reduction, and augmentation mammoplasty. Additionally, in a study by Seth et al., three specialist plastic and reconstructive surgeons evaluated ChatGPT’s ability to create safe and high-quality breast augmentation material by asking plastic surgeons to qualitatively assess ChatGPT-generated responses to six breast augmentation questions. The researchers also performed a literature search to assess the accessibility, informativeness, and accuracy of the responses[22]. ChatGPT was found to provide comprehensive and grammatically accurate responses but lacked personalized advice[22]. Xie et al. discovered similar results to those of Seth et al. when investigating the use of ChatGPT to generate responses to rhinoplasty questions from the American Society of Plastic Surgeons (ASPS) website[23]. Responses were evaluated by four plastic surgeons qualitatively for accuracy, informativeness, and accessibility by plastic surgeons[23]. Surgeons determined that the ChatGPT provided comprehensive and coherent answers, yet ChatGPT was limited in providing personalized advice critical for quality patient consultation[23].
Regarding cleft lip and palate repairs, Fazilat et al. used paired t-tests to compare ChatGPT-generated responses to thirty cleft lip and palate questions with information from four academic and professional sources for quality and readability[31]. Eleven plastic surgeons evaluated the comprehensiveness, clarity, and accuracy of the two sources and selected the sources they preferred to create the highest-quality information[31]. Twenty-nine non-medical individuals only selected the source they preferred. Plastic surgeons scored ChatGPT significantly higher than the academic and professional sources regarding comprehensiveness (P < 0.0001) and clarity (P < 0.001)[31]. Additionally, plastic surgeons and non-medical individuals preferred ChatGPT cleft lip and palate information 60.88% and 60.46% of the time, respectively[31]. The number of inaccuracies in ChatGPT and the academic and professional sources were similar. Additionally, the readability level of both sources exceeded the 6th recommended by the NIH according to the following readability formulas: Flesch-Kincaid Grade Level, Flesch-Kincaid Readability Ease, Gunning Fog Index, Simple Measure of Gobbledygook Index, Coleman-Liau Index, Linsear Write Formula, and Automated Readability Index[31]. The results of this study highlight ChatGPT’s ability to produce quality cleft lip and palate information that plastic surgeons and non-medical individuals prefer against currently available academic and professional sources[31]. Likewise, in a study by Chaker et al., two senior pediatric plastic surgeons qualitatively evaluated the accuracy of ChatGPT-generated cleft lip and palate repair response to common postoperative questions against their expert responses[24]. The two pediatric plastic surgeons determined that the accuracy rate of ChatGPT-generated information was 69% compared to their expert responses, once again demonstrating that ChatGPT has the potential to generate patient education material and can reduce physician workload[24]. Therefore, ChatGPT may be used to produce high-quality information for patients across multiple disciplines, though more personalized output may be needed.
Effectiveness of AIVAs in producing patient educational material
AIVAs utilize NLP to comprehend human speech and provide answers in a conversational form. AIVAs have already been used by major technology companies such as IBM to answer customer inquiries without human assistance[32], and in a similar fashion, Boczar et al. evaluated the AIVAs’ ability to respond to plastic surgery FAQs[32]. Their study trained AIVAs to accurately answer commonly asked questions to ten frequent patient concerns in plastic surgery[32]. Individuals were then asked to complete a Likert scale and indicate if the AIVA response was correct and to evaluate its potential use as a source of patient-facing information[32]. AIVA answered plastic surgery patients’ frequently asked questions correctly 92.3% of the time, while participants believed that only 83.3% of AIVA’s answers were correct[32]. Interestingly, according to the Likert scale, patients were neutral when asked if the technology could replace human assistance[32]. Overall, AIVAs may have a future role in providing accurate information to routine surgical questions, though further refinement is necessary before more widespread adoption by providers and acceptance by patients.
PRE AND POSTOPERATIVE ASSESSMENTS
Breast reconstruction
Patient satisfaction is a central goal of breast reconstruction, and delivering patient-centered treatment during the reconstructive process can help improve the perception of quality of care[16]. ML methods have accordingly enabled more patient-specific care in PRS and other disciplines of surgery [Figure 2]. For example, ML has been used to assist clinicians in reconstructive method selection, preoperative planning, facilitation of postoperative monitoring, enhancement of patient outcomes, and to decrease hospital readmissions [Table 1][33]. In a pilot study by Mavioso et al., the preoperative utility of ML was evaluated for semi-automatic assessment of Angio CT imaging for forty patients scheduled for deep inferior epigastric perforators (DIEP) breast reconstruction[34] [Table 2]. Specifically, Mavioso et al. utilized a paired sample
Benefits and challenges of AI in pre- and postoperative assessments
Phase | Plastic surgery current limitations | AI-based solutions | Benefits of AI | Challenges of AI |
Preoperative | - Imaging and diagnostic - Surgical planning - Personalized visualization - Predictive outcomes | 3D imaging and modeling AI algorithms process patient data to create detailed 3D models for surgical planning | - High precision and customization - Enhanced visualization - Improved patient-surgeon communication | - High cost - Requires extensive training - Data privacy concerns |
AR AR overlays digital information onto the real-world surgical field, enhancing visualization and precision | - Real-time guidance - Supports minimally invasive techniques - Valuable educational tool | - Technical limitations - Integration challenges - High cost | ||
Predictive analytics ML models analyze patient data to predict potential complications and outcomes | - Early identification of risks - Personalized surgical plans - Optimized resource allocation | - Dependent on data quality - Potential for algorithmic bias to training data - Integration complexity | ||
Breast, facial, hand, and wound healing (Skin) assessments AI aids in selecting reconstructive methods, preoperative planning, and evaluating imaging data | - Reduced planning time - Enhanced monitoring - Improved satisfaction and reduced readmissions | - Accuracy issues with small vessels - Discrepancies in vertical component estimation for breast reconstruction | ||
Postoperative | - Managing surgical complications and patient information - Variability in outcomes - Subjective results and evaluation - Patient satisfaction | Telemedicine and remote monitoring AI-driven platforms monitor patient recovery remotely, ensuring continuous communication and support | - Continuous support - Increased accessibility - Improved adherence to protocols | - Technology barriers - Data security concerns - Limited physical examination |
Predictive analytics AI models continue to predict complications based on ongoing patient data, facilitating early interventions | - Timely intervention - Reduced morbidity and mortality - Personalized care | - Data dependency - Algorithmic bias to training data - Complexity in clinical workflow integration | ||
AI-enhanced readability of patient education materials AI tools simplify medical information to improve patient comprehension and adherence to postoperative care instructions | - Increased patient understanding - Better adherence to recovery protocols - Improved outcomes | - Need for further refinement - Ensuring personalized advice - Patient trust and reliability issues | ||
Breast, facial, hand, and wound healing (skin) monitoring Smartphone apps and ML algorithms monitor status and predict complications like infections and functional recovery | - Early detection of complications - Improved monitoring - Higher predictive accuracy | - Technical limitations - Dependence on image quality and data input |
Description of primary research studies analyzed
Section | Author | Title | Year | Journal | Main aim | Main finding |
AI-enhanced readability of patient education | Wang et al.[28] | Artificial intelligence in plastic surgery: ChatGPT as a tool to address disparities in health literacy | 2024 | Plastic and Reconstructive Surgery | (1) Evaluate the readability of 10 online sources of breast reconstruction information against those modified by ChatGPT (2) Assess ChatGPT’s ability to improve the readability of patient-facing medical information | (1) ChatGPT generally improved readability, but only one website showed statistically significant improvement (2) Readability scores still exceeded the 5th-grade level, indicating a need for more simplification of patient information |
Baldwin et al.[19] | An artificial intelligence language model improves readability of burn first aid information | 2024 | Burns | (1) Assess the effectiveness of ChatGPT in improving the readability of burn first aid information (2) Evaluate the changes in the readability of online patient education materials after ChatGPT modification | (1) After ChatGPT modifications, 18% of webpages met an 11-year-old literacy level, compared to only 4% prior to ChatGPT modifications (2) ChatGPT significantly improved readability scores across all readability formulas (P < 0.001) | |
Browne et al.[17] | ChatGPT-4 can help hand surgeons communicate better with patients | 2024 | Journal of Hand Surgery Global Online | (1) Evaluate ChatGPT’s ability to improve the readability of hand surgery information provided by the American Society of Surgery of Hand and the British Society of Surgery of the Hand (2) Use multiple readability formulas to compare pre and post-ChatGPT modified readability scores of hand surgery content | (1) ChatGPT significantly improved the readability of hand surgery information (P < 0.001) across all readability tests (2) ChatGPT-modified information achieved a mean sixth-grade readability level on multiple readability tests | |
Evaluation of AI-generated patient-facing information | Berry et al.[20] | Both patients and plastic surgeons prefer artificial intelligence - generated microsurgical information | 2024 | Journal of Reconstructive Microsurgery | (1) Compare the quality of ChatGPT-generated responses to microsurgery questions against those provided by the ASRM (2) Assess the comprehensiveness, clarity, and readability of ChatGPT’s responses against ASRM content | (1) Surgeons preferred ChatGPT’s responses 70.7% of the time and rated ChatGPT higher in terms of comprehensiveness (P < 0.001) and clarity (P < 0.05) (2) ChatGPT’s responses had significantly worse readability scores across multiple readability formulas compared to ASRM content |
Grippaudo et al.[21] | Quality of the information provided by ChatGPT for patients in breast plastic surgery: are we already in the future? | 2024 | JPRAS Open | (1) Assess the quality of ChatGPT-generated breast plastic surgery information using the EQIP scale (2) Evaluate ChatGPT’s generated patient-facing breast surgery information across content, identification, and structure data | (1) ChatGPT produced high-quality information for breast reconstruction (19/36), reduction (19/36), and augmentation (20/36), scoring above 18 on the EQIP scale (2) ChatGPT information lacked identification since the information did not contain bibliography references or proper validation | |
Seth et al.[22] | Evaluating chatbot efficacy for answering frequently asked questions in plastic surgery: a ChatGPT case study focused on breast augmentation | 2023 | Aesthetic Surgery Journal | (1) Evaluate ChatGPT’s ability to create accurate, informative, and accessible breast augmentation information (2) Qualitatively compare ChatGPT’s responses to breast augmentation questions with established literature | (1) ChatGPT provided comprehensive and grammatically accurate responses to breast augmentation questions (2) ChatGPT-generated information lacked personalized advice | |
Xie et al.[23] | Aesthetic surgery advice and counseling from artificial intelligence: a rhinoplasty consultation with ChatGPT | 2023 | Aesthetic Plastic Surgery | (1) Investigate ChatGPT’s ability to generate accurate, informative, and accessible responses to rhinoplasty questions sourced from the ASPS website (2) Evaluate the quality of ChatGPT’s responses in a clinical setting | (1) ChatGPT provided comprehensive and coherent answers to rhinoplasty questions (2) ChatGPT lacked the ability to generate personalized advice, limiting its usefulness in patient consultations | |
Fazilat et al.[31] | AI-based cleft lip and palate surgical information is preferred by both plastic surgeons and patients in a blind comparison | 2024 | The Cleft Palate Craniofacial Journal | (1) Compare the quality and readability of ChatGPT-generated response to cleft lip and palate questions against those provided by academic and professional sources (2) Evaluate comprehensiveness, clarity, accuracy, and preference | (1) Plastic surgeons rated ChatGPT-generated information higher for comprehensiveness (P < 0.0001) and clarity (P < 0.001), and both plastic surgeons and non-medical individuals preferred ChatGPT 60.88% and 60.46% of the time, respectively (2) ChatGPT and the academic and professional sources exceeded the NIH’s recommended readability level | |
Chaker et al.[24] | Easing the burden on caregivers-applications of artificial intelligence for physicians and caregivers of children with cleft lip and palate | 2024 | The Cleft Palate Craniofacial Journal | (1) Assess the accuracy of AI-generated responses for cleft lip and palate repair postoperative questions by comparing them to expert responses from pediatric plastic surgeons (2) Evaluate ChatGPT’s ability to reduce physician workload by generating patient education material | (1) AI-generated information had a 69% accuracy rate compared to expert responses, showing potential in creating patient education materials (2) Although AI can reduce physician workload, more personalized outputs are necessary for higher-quality patient care | |
Effectiveness of AIVAs in producing patient educational material | Boczar et al.[32] | Artificial intelligent virtual assistant for plastic surgery patient’s frequently asked questions: a pilot study | 2020 | Annals of Plastic Surgery | (1) Evaluate the accuracy of AIVAs in answering frequently asked plastic surgery questions (2) Assess patient perceptions of AIVA responses as a source of patient-facing information | (1) AIVAs answered 92.3% of plastic surgery FAQs correctly, although participants marked only 83.3% of responses as accurate (2) According to a Likert scale, patients were neutral regarding AIVAs’ potential to replace human assistance |
Breast reconstruction | Mavioso et al.[34] | Automatic detection of perforators for microsurgical reconstruction | 2020 | The Breast | (1) Reduce duration and subjectivity of the preoperative Angio CT using CV for DIEP flaps breast reconstruction | (1) Reduced time for Angio CT from 2 h per patient to 30 min (2) Automatic perforator detection was better with the software compared to the radiology team when estimating large vessels (3) Software showed more difficulties estimating the caliber of smaller perforators |
Kiranantawat et al.[35] | The first smartphone application for microsurgery monitoring: SilpaRamanitor | 2014 | Plastic and Reconstructive Surgery | (1) Develop and evaluate a free flap monitoring system using mobile phone technology | (1) The smartphone application is sensitive (94%), specific (98%), and accurate for venous (93%) and arterial occlusion (95%) (2) Potential applications for early detection of flap failure | |
Myung et al.[36] | Validating machine learning approaches for prediction of donor-related complication in microsurgical breast reconstruction: a retrospective cohort study | 2021 | Scientific Reports | (1) Evaluate a ML prediction model for abdominal flap donor site complications in breast reconstruction and determine factors influencing these complications using logistic regression | (1) Neuralnet was identified as the most effective ML package for predicting donor site complications (2) Significant factors affecting complications included the size of the fascial defect, history of diabetes, muscle-sparing type, and adjuvant chemotherapy (3) The risk cutoff for fascial defect was 37.5 cm2, with a high-risk group showing a 26% complication rate compared to 1.7% in the low-risk group | |
Hassan et al.[37] | Artificial intelligence modeling to predict periprosthetic infection and explantation following implant-based reconstruction | 2023 | Plastic and Reconstructive Surgery | (1) Develop, validate and evaluate the use of ML algorithms to predict complications of implant-based reconstructions | (1) ML showed strong discriminatory performance in predicting periprosthetic infection and explantation, with AUC values of 0.73 and 0.78, respectively (2) ML identified 9 and 12 predictors of periprosthetic infection and explantation, respectively | |
Facial surgery | Zhang et al.[39] | Turning back the clock: artificial intelligence recognition of age reduction after facelift surgery correlates with patient satisfaction | 2021 | Plastic and Reconstructive Surgery | (1) Evaluate the effectiveness of facelift surgery in reducing perceived age and patient satisfaction using convolutional neural networks and FACE-Q patient-reported outcomes | (1) Four neural networks accurately estimated preoperative age, with an average accuracy score of 100.8 (2) Patients reported a greater perceived age reduction (-6.7 years) compared to the neural network estimates (-4.3 years) (3) FACE-Q scores indicated high patient satisfaction with facial appearance, quality of life, and overall outcome (4) A positive correlation was found between neural network age reduction estimates and patient satisfaction |
Boonipat et al.[40] | Using artificial intelligence to measure facial expression following facial reanimation surgery | 2020 | Plastic and Reconstructive Surgery | (1) Evaluate the use of ML to measure facial expression before and after facial reanimation surgery using video data | (1) The facial recognition application showed a greater recognition of happy signals in postoperative (42%) vs. preoperative (13%) smile videos (P < 0.0001) compared to 53% in control videos | |
Geisler et al.[41] | A role for artificial intelligence in the classification of craniofacial anomalies | 2021 | Journal of Craniofacial Surgery | (1) Develop CNN models based on the ResNet-50 architecture to classify non-syndromic CS from 2D clinical photographs | (1) CNN model developed showed an overall testing accuracy of 90.6%, demonstrating the potential of ML to detect craniofacial conditions | |
Knoops et al.[42] | A machine learning framework for automated diagnosis and computer-assisted planning in plastic and reconstructive surgery | 2019 | Scientific Reports | (1) Develop the first fully automated large-scale clinical 3DMM for supervised learning in diagnostics, risk stratification, and treatment simulation, and to demonstrate its potential for improving clinical decision making in orthognathic surgery | (1) The developed 3DMM achieves a diagnostic sensitivity of 95.5% and specificity of 95.2% (2) The model simulates surgical outcomes with a mean accuracy of 1.1 ± 0.3 mm (3) The 3DMM framework automates diagnosis and provides patient-specific treatment plans from 3D scans, improving efficiency in clinical decision making | |
Lim et al.[44] | Using generative artificial intelligence tools in cosmetic surgery: a study on rhinoplasty, facelifts, and blepharoplasty procedures | 2023 | Journal of Clinical Medicine | (1) Investigating the capacity of AI tools to generate realistic images pertinent to cosmetic surgery | (1) DALL-E-2, Midjournet and Blue Willow showed a higher representation of females, light skin tones, and with a BMI < 20 (2) AI tools could enhance patient information but must be integrated ethically to ensure comprehensive representation and maintain medical standards | |
Hand surgery | Ozkaya et al.[46] | Evaluation of an artificial intelligence system for diagnosing scaphoid fracture on direct radiography | 2022 | European Journal of Trauma and Emergency Surgery | (1) Determine the diagnostic performance of ML to detect scaphoid fractures on anteroposterior wrist radiographs | (1) ML demonstrated 76% sensitivity, 92% specificity, an AUC of 0.840, a Youden index of 0.680, and an F-score of 0.826 for detecting scaphoid fractures (2) The experienced orthopedic specialist had the highest diagnostic performance based on AUC, while ML performance was comparable to that of a less experienced orthopedic specialist and superior to the ED physician |
Oeding et al.[47] | Diagnostic performance of artificial intelligence for detection of scaphoid and distal radius fractures: a systematic review | 2024 | The Journal of Hand Surgery | (1) Determine the diagnostic efficacy of AI models for detecting scaphoid and distal radius fractures (2) Compare the efficacy to human clinical experts | (1) AI models exhibited strong diagnostic performance, with AUROC values ranging from 0.77 to 0.96 for scaphoid fractures and 0.90 to 0.99 for distal radius fractures. Accuracy ranged from 72.0% to 90.3% for scaphoid fractures and 89.0% to 98.0% for distal radius fractures (2) Compared to clinical experts, 92.9% of the studies found AI models to have comparable or better performance. AI models generally showed poorer performance on occult scaphoid fractures, though models specifically trained for these types of fractures performed significantly better | |
Hoogendam et al.[48] | Predicting clinically relevant patient-reported symptom improvement after carpal tunnel release: a machine learning approach | 2022 | Neurosurgery | (1) Develop a prediction model that estimates the probability of clinically relevant symptom improvement 6 months after CTR (2) Evaluate the model’s discriminative ability and calibration using various ML techniques and apply it to support shared decision making for patients considering CTR | (1) A gradient boosting machine model with 5 predictors was identified as the best balance between discriminative ability and simplicity, achieving an AUC of 0.723 in the holdout data set (2) The model demonstrated good calibration, with a sensitivity of 0.77, specificity of 0.55, positive predictive value of 0.50, and negative predictive value of 0.81 (3) The prediction model, which uses 5 patient-reported predictors (18 questions), has reasonable discriminative ability and good calibration, and is available online to assist in shared decision making for patients considering CTR | |
Loos et al.[49] | Machine learning can be used to predict function but not pain after surgery for thumb carpometacarpal osteoarthritis | 2022 | Clinical Orthopaedics and Related Research | (1) To develop and validate prediction models for clinically important improvement in pain and hand function 12 months after surgery for thumb carpometacarpal osteoarthritis (2) Assess the performance of various predictive models using logistic regression, random forests, and gradient boosting machines to support preoperative decision making | (1) The random forest model for pain prediction showed poor performance with an AUC of 0.59 and poor calibration (2) The gradient boosting machine model for hand function improvement had a good AUC of 0.74 and good calibration, using only the baseline hand function score as a predictor (3) A web application is available for the hand function model, which could aid in clinical decision making, though the pain prediction model is not yet suitable for clinical use | |
Wound healing and burn surgery | Kim et al.[50] | Predicting the severity of postoperative scars using artificial intelligence based on images and clinical data | 2023 | Scientific Reports | (1) Develop and evaluate an AI model using images and clinical data to predict the severity of postoperative scars (2) Compare the performance of this AI model to that of dermatologists | (1) The AI model reached a high level of accuracy (ROC-AUC 0.931 for images alone, 0.938 combined with clinical data) (2) The model also performed at a comparable level to that of 16 dermatologists |
Squiers et al.[51] | Machine learning analysis of multispectral imaging and clinical risk factors to predict amputation wound healing | 2022 | Journal of Vascular Surgery | (1) Develop a ML algorithm using multispectral imaging data and clinical risk factors to predict amputation wound healing and reduce the need for reoperation | (1) The ML algorithm had high sensitivity (91%) and specificity (86%) for prediction of non-healing amputation sites (2) ML algorithms could reduce reoperation rates, improve healing outcomes, and potentially decrease costs and patient length of stay | |
Robb[52] | Potential for machine learning in burn care | 2022 | Journal of Burn Care & Research | (1) Explore the potential implementation of various ML methods (such as linear and logistic regression, deep learning, and neural networks) in burn care within the NHS in the UK (2) Focus on optimizing care through ML applications in burn assessment | (1) The use of ML in burns holds the potential to improve prevention, burns assessment, mortality predictions, and critical care monitoring (2) Successful implementation requires investment in data capture and training (3) ML technology has the potential to improve diagnostic accuracy, objective decision making, and resource allocation | |
Xue et al.[53] | Artificial intelligence - assisted bioinformatics, microneedle, and diabetic wound healing: a “new deal” of an old drug | 2022 | ACS Applied Materials & Interfaces | (1) Explore potential therapeutic agent TSA for diabetic wound healing with AI-assisted bioinformatics (2) Investigate the effectiveness of TSA in targeting HDAC4 (3) Develop a microneedle-mediated patch for TSA delivery to improve treatment efficacy and reduce secondary damage | (1) TSA via microneedle patch reduces inflammation, promotes tissue regeneration, and inhibits HDAC4 in diabetic wound healing (2) This approach offers a minimally invasive and safe treatment method with broad applications in biomedical fields | |
3D and predictive modeling | Knoops et al.[42] | A machine learning framework for automated diagnosis and computer-assisted planning in plastic and reconstructive surgery | 2019 | Scientific Reports | (1) Develop a ML framework for automated diagnosis, risk stratification, and treatment in PRS (2) Enhance precision and efficiency in ML-assisted surgical planning to improve clinical decision making and outcomes | (1) This approach offers high diagnostic accuracy (95.5% sensitivity and 95.2% specificity) and simulates surgical outcomes with a mean accuracy of 1.1 ± 0.3 mmc (2) This framework can automate diagnosis and provide patient-specific training from 3D models |
Knoops et al.[55] | A novel soft tissue prediction methodology for orthognathic surgery based on probabilistic finite element modeling | 2018 | PloS One | (1) Develop a probabilistic FEM to predict postoperative facial soft tissues following orthognathic surgery (2) Addressing the limitations of prediction models by including variability and uncertainty in the prediction process | (1) The probabilistic FEM was validated on 8 patients (2) The FEM accurately predicted changes in the nose and upper lip but underestimated changes in the cheeks and lower lip (3) This model offers patients and surgeons a more comprehensive understanding of surgical impacts | |
3D printing for planning and implantation | Chae et al.[62] | 3D volumetric analysis for planning breast reconstructive surgery | 2014 | Breast Cancer Research and Treatment | (1) Develop a new approach to volumetric analysis for breast reconstructive surgery using 3D photography (2) Improve accuracy in assessing breast volume, shape, and projection compared to traditional 2D photography | (1) Multiple techniques for volumetric analysis for breast asymmetry were reported (2) Breast volumes can be visualized through 3D images, accurately calculated, and produced as 3D haptic models for operative guidance |
ML algorithms can also be valuable for the prompt detection of complications following breast surgery. By analyzing available patient data, these algorithms can identify patterns and determine the associations among relevant variables[1]. Kiranantawat et al. developed the first smartphone application for microsurgery monitoring by training the algorithm with photographic data of fingers undergoing venous or arterial congestion[35]. Across forty-two participants, the application successfully assessed the vascular status of fingers with a sensitivity and specificity of 94% and 98%, respectively[35]. This study suggests that ML could enhance early detection of postoperative flap failure and help optimize monitoring of the flap after surgery. Another study by Myung et al. developed an ML model to determine patient-specific characteristics and surgical factors that lead to an increased risk of donor site complications after the performance of abdominal flaps for breast reconstruction[36]. After analyzing 568 patients, Myung et al. discovered that the algorithm was able to accurately predict complications [area under the curve (AUC): 0.89] and could further be used as a reference for assessing the individual risk associated with abdominal flaps[36].
Additionally, ML has been applied to minimize postoperative infection following implant-based reconstruction, including the development of internal algorithms to guide clinical decisions such as the need for reoperation or introduction of antibiotics[1]. Hassan et al. developed an algorithm using ML to predict periprosthetic infection and explantation[37]. The study demonstrated that ML models can provide a higher predictive accuracy compared to multivariate logistic regression for periprosthetic infection and explantation[37,38]. Therefore, ML could help reduce postoperative burden and promote better outcomes in breast reconstruction.
Facial surgery
In the context of facial surgery, photographic data provides a means to assess surgical success or failure, and it can also be used as a tool for enhancing communication between patient and physician. ML has been explored in combination with photographic data to maintain proper standardization of procedures and offer more precise postoperative assessment[39]. Using pre- and postoperative pictures, Zhang et al. showed that neural networks could identify preoperative age and facial age reduction following facelift surgery[39]. A positive correlation between the algorithmically determined result and patient satisfaction after facelift was identified, representing a validated method of quantifying postoperative results and efficacy for plastic surgeons[39]. In another study, Boonipat et al. used ML to assess postoperative facial expression improvement after facial reanimation surgery[40]. Recording of facial expressions was performed for each patient in a video clip and analyzed with ML software to detect facial expressions[40]. ML algorithms were found to be capable of reading facial emotional expressions and providing a quantification of those expressions. These tools may thus be helpful in assessing facial palsy and the success of postoperative outcomes[40]. Moreover, corrective procedures, the use of neurotoxins, or soft tissue fillers could utilize ML as an assessment tool for photographic or recorded data[39,40].
ML has also been applied to perform automatic detection of craniofacial conditions, facilitating early diagnosis based on photographic images and annotated datasets. In an early study by Geisler et al., neural networks have successfully achieved an overall testing accuracy of 90.6% for the detection of craniosynostosis, opening the field for earlier diagnosis and minimizing the need for CT scans[41]. Another study by Knoops et al. described a computer-assisted model framework involving supervised learning for diagnostic, predictive outcome and treatment stimulation in craniofacial surgery[42]. The algorithm was trained on non-ionizing 3D face scans of healthy faces and orthognathic patients, and it provided an accurate classification with a 95.5% sensitivity and 95.2% specificity[42]. The algorithm was also able to stimulate patient-specific postoperative outcomes with a mean accuracy of 1.1 +/- 0.3 mm. compared to conventional surgical planning, suggesting that the model could predict the postoperative shape of the face in a single step and reduce the time for the planning process[42].
In addition to data classification, the advent of generative AI tools such as DALL-E2 can enable the creation of various types of synthetic images or text on demand[43]. Cosmetic surgery, given its inherently visual nature, can, therefore, take advantage of generative AI to simulate post-surgery results even prior to the procedure. In one study by Lim et al., DALL-E2, Midjourney and Blue Willow were evaluated in their utility to provide images clinically relevant after cosmetic surgery[44]. In future cases, surgeons could virtually simulate different interventions and examine the AI outcomes with patients for preoperative scoring and evaluation, which may help surgeons fine-tune the planned procedure and aim for specific modeled outcomes.
Hand surgery
Similar to radiology and other imaging-dominated disciplines, AI has been applied in hand surgery for fracture detection[45]. Despite the performance of standard clinical examination and X-ray characterization, scaphoid fractures, representing 15% of acute wrist fractures, are missed initially in nearly 16% of cases[45]. Therefore, there is an existing need for ML algorithms to improve the detection of scaphoid fractures, wrist fractures, and other cases within emergency departments. In a recent study by Ozkaya et al., an ANN model for scaphoid fracture detection on anteroposterior wrist radiographs was compared to three physicians (two orthopedic specialists and one physician in the emergency department)[46]. The ANN showed a 76% sensitivity and 92% specificity, which exceeded the performance of emergency department physicians, but still lagged behind that of an experienced orthopedic specialist[46]. However, the addition of clinical examination findings in the algorithm, as well as lateral views, could enhance the sensitivity of the ML algorithm. Further, Oeding et al. discovered that recent AI models have demonstrated excellent performance in detecting scaphoid fractures and radius fractures, with AUC values of 0.77-0.96 and 0.90-0.99, respectively[47]. The majority of AI models have currently demonstrated comparable or better performance than many clinical experts, and further improvements in speed and performance may result from larger data sets, more powerful computing resources, and increasingly open-source code toolboxes[47].
Based on patient-specific data such as age, smoking status, dominant hand, occupation, and subtype of fracture, ML could also provide useful information in the acute setting, such as deciding whether to perform one type of hand surgery over another (e.g., replantation vs. amputation)[48,49]. Recently, two studies by Hoogendam et al. and Loos et al. from the Hand and Wrist Study Group in the Netherlands have introduced user-friendly graphical applications, supported by ML algorithms, for predicting postoperative function[48,49]. These ML approaches were applied to data from 2,119 patients with carpal tunnel syndrome (CTS) and 2,653 patients with thumb carpometacarpal osteoarthritis[48,49]. These applications calculated the probability of functional improvement 6 months after CTS surgery and 12 months after first carpometacarpal joint surgery, based on preoperative patient-reported outcome measures (PROMs). While these ML algorithms are freely available, it is crucial to note that such online applications often lack external validation, which is essential for ML algorithms to be generalized to other patient cohorts.
Wound healing and burn surgery
While postoperative wound assessment and management are essential to ensure optimal treatment, no “gold standard” for scar evaluation currently exists[50]. Challenges in scar evaluation may arise from the variability in clinical assessments and difficulty in achieving consistent accuracy in follow-up evaluations. A recent study by Kim et al. introduced DNN models to classify postoperative scars based on scar severity. This model was trained with both an image-based AI model and a model based on clinical variables related to postoperative scars, such as patient demographic data, scar age, and symptoms[50]. Four scar severity groups were successfully classified using these image-based AI models at a performance level comparable to that of board-certified dermatologists, underscoring the efficacy of AI in clinical assessments[50]. A pilot study by Squiers et al. separately demonstrated the utility of combining image-based analysis with ML risk factor assessment in predicting the healing outcomes of primary amputation wounds[51]. The level of amputation was determined by the subject’s surgeon prior to imaging, and was based on clinical judgment such as patient history, physical exam, and any perfusion studies[51]. Multispectral imaging of the subjects’ lower extremity planned for amputation was also conducted on postoperative day 30[51]. Analysis of multispectral imaging demonstrated greater effectiveness in predicting primary amputation wound healing relative to surgeon judgment, with an 88% accuracy rate compared to 56%[51]. If further evaluation and/or external validation confirm these findings, this type of ML tool may enhance the decision-making process in wound healing treatments.
Certain complex wounds, such as those from diabetes and burns, are particularly susceptible to complications and delayed healing due to impaired circulation and increased risk of infection. Recently, advancements in burn management have been integrated with AI tools to enhance the treatment of burn wounds[19]. DL-based analysis models were able to identify the depth of early burn wounds using inputs based on clinical photographs of the wound. Furthermore, in a study by Robb et al., ANNs provided accurate diagnoses of burn injuries based on color attributes and successfully classified burns into standardized categories, achieving a diagnostic accuracy of approximately 80%[52]. Apart from their beneficial role in burn assessment, AI algorithms have the potential to aid in clinical decision making by accurately predicting clinical outcomes in wound healing, such as the need for skin grafts or amputation[52]. Diabetic wounds similarly pose significant challenges in the clinic due to their higher complications and slow healing, which may be addressed using AI techniques for modeling and therapeutic discovery[53]. In one early example, Xue et al. used AI tools to identify a novel therapeutic agent for diabetic wound healing by predicting molecular interactions between the drug Trichostatin A and its receptors at the wound site[53]. Ultimately, AI tools can support the personalized treatment of wounds and burns by integrating clinical data, patient-specific risk factors, biological modeling, and prediction of potential postoperative complications.
3D TISSUE MODELING AND PRINTING
3D and predictive modeling
Given the unique size and geometry of each patient’s surgical site, 3D modeling has been utilized for patient-specific surgical planning[42]. Similarly, the advent of 3D printing technologies has enabled the design of increasingly site- and patient-specific constructs for surgical implantation[54]. Digital models of a surgical site can be reconstructed using traditional medical imaging techniques such as CT or magnetic resonance imaging (MRI)[42]. In addition to supporting the preoperative planning of incisions, positioning, and other factors, these models can utilize AI methods to simulate patient-specific changes in tissue geometry (e.g., facial shape) that may result from a procedure[42,55]. In one example, Knoops et al. utilized finite element modeling to develop patient-specific predictions of maxillofacial transformation based on preoperative and postoperative CT scans[55]. The authors also utilized experimental design to infer the contributions of input parameters, including Young’s modulus and viscoelasticity, to soft tissue displacement[55]. In other examples, surgeon-scientists have utilized generative AI to produce 3D models of facial shapes based on 2D medical images[42,56-58]. For instance, a 3D morphable model trained on over 4,000 faces has been applied for the diagnosis, risk stratification, and treatment simulation of jaw surgery patients[42]. Ultimately, ML algorithms have demonstrated the potential to help improve surgical outcomes and reduce medical costs by creating patient-specific predictive models prior to operation[56].
3D printing for planning and implantation
In addition to 3D modeling, 3D printing may be utilized in combination with AI to generate increasingly patient-specific models and implants. On the printing and technological side, ML can be utilized to identify the optimal printing parameters to generate a desired shape and/or internal architecture[59]. Hierarchical ML algorithms, for instance, have been used to identify optimal material formulations, process variables, and fiber geometries for the production of silicone implants and other constructs[60,61]. With the support of AI methods, patient-specific models of the breast, vasculature, craniofacial tissue, and more have accordingly been fabricated by 3D printing for purposes of preoperative planning[54]. Chae et al., for instance, used CT and MRI scans to visualize and print breast tissue models for mastectomies[62]. In addition to supporting the planning process, “bedside” 3D printing has been explored in some early instances for the development of patient-specific implants[54]. In one notable example, Lei et al. utilized ML models to design cochlear implants with optimal electro-anatomical properties given the patient’s specific inner ear geometry[63]. Ultimately, 3D printing methods can produce more geometrically complex and site-specific constructs compared to traditional fabrication methods, particularly when paired with AI models.
DISCUSSION
Plastic surgery is a growing field, with cosmetic and surgical procedures recently seeing more than a 5% annual increase, according to the ASPS[64]. As the specialty advances with recent innovations such as minimally invasive treatments, organ transplantation, super microsurgery, and the integration of AI, it continues to encounter challenges related to patient information, pre- and postoperative assessments, and preoperative planning[64]. The subjective nature of plastic surgery often leads to variability in result assessments, and it requires tailored procedures to accommodate individual/ethnic features as well as excellent comprehension between the surgeon and the patient to obtain satisfaction with results[16,65].
AI is considered an innovation in plastic surgery, characterized as “something new or a modification to an existing product, idea, or field”[64]. Applications of AI tools have the potential to significantly address the limitations of plastic surgery by improving the efficiency and precision of surgical procedures, diagnostic analytics, and patient outcomes. ML algorithms can also analyze patient-specific data to create highly personalized treatment plans and predict surgical success in a more accurate manner[66]. Virtual planning, 3D modeling, and patient-specific cutting guides allow for increased precision, decreased operative time, and improved cosmetic outcomes[67]. Additionally, autonomous surgical robots are emerging as a novel AI-based healthcare technology. These robots have the potential to be trained using cadavers, similar to students learning through dissecting cadavers, allowing the robots to experience a full-contact ML environment[68]. These advancements are particularly beneficial to plastic surgery due to the intricate and complex nature of these procedures. While these tools have shown to be promising, the benefits closely depend on the quality of input data and the ability to address potential inherent biases within AI models. The use of large datasets and varying patient demographics could affect the accuracy of AI predictions and introduce certain biases if the training data are not diverse. Ethical considerations, such as ensuring patient data privacy and maintaining transparency of AI-driven decision making, must also be considered for the successful integration of AI into clinical practice[69].
Given these challenges, it is essential to standardize the methodologies for using AI in surgical practice and patient care. Future studies should focus on developing uniform protocols for data collection and analysis, which can be achieved by implementing standardized imaging techniques, applying consistent data annotation, and establishing clear criteria for validating the accuracy of AI tools. These protocols would help support the production of reliable and comparable results by AI, regardless of the clinical setting or patient demographic. In addition to standardization, future research should prioritize conducting longitudinal studies to best assess the sustainability and long-term outcomes of AI-assisted procedures. These efforts will help researchers pinpoint areas where AI provides significant benefits and where further improvements can be made.
CONCLUSION
With ongoing increases in healthcare data collection and the efficacy of AI tools, the utility of AI models for improving patient-specific care continues to grow. AI applications may become integral to various areas of plastic surgery, including breast reconstruction, craniofacial surgery, hand surgery, burns and wound healing surgery. Further, AI can assist surgeons in providing more detailed preoperative counseling to patients and may also improve patient-surgeon communication. Automated postoperative simulations may also be increasingly utilized before surgery to help patients understand the expectations of an operation, answer patients’ questions, and ultimately improve postoperative satisfaction. In the future, these personalized simulations may be combined with 3D modeling and printing techniques to create patient-specific constructs for reconstructive procedures. However, it is crucial to ensure that the adoption of these technologies does not negatively impact the patient-surgeon relationship by decreasing physical examination, exposing the patient to data security concerns, or introducing any other unintended consequences. Nonetheless, the utilization of AI in surgery continues to grow rapidly, and these new tools have already demonstrated the potential to enable more time-efficient, precise, and patient-specific clinical care.
DECLARATIONS
Authors’ contributions
Made substantial contributions to the conception and design of the study: Brenac C, Fazilat AZ, Guo JL
Writing and editing: Brenac C, Fazilat AZ, Guo JL, Fallah M, Kawamoto-Duran D, Sunwoo PS
Made the figures: Brenac C
Conception and review of the manuscript: Wan DC, Guo JL, Longaker MT
Availability of data and materials
Not applicable.
Financial support and sponsorship
Brenac C is supported by the University of Claude Bernard Lyon 1, “Année recherche”.
Conflicts of interest
All authors declared that there are no conflicts of interest.
Ethical approval and consent to participate
Not applicable.
Consent for publication
Not applicable.
Copyright
© The Author(s) 2024.
REFERENCES
1. Maita KC, Avila FR, Torres-Guzman RA, et al. The usefulness of artificial intelligence in breast reconstruction: a systematic review. Breast Cancer 2024;31:562-71.
2. Guo JL, Januszyk M, Longaker MT. Machine learning in tissue engineering. Tissue Eng Part A 2023;29:2-19.
3. Choi E, Leonard KW, Jassal JS, Levin AM, Ramachandra V, Jones LR. Artificial intelligence in facial plastic surgery: a review of current applications, future applications, and ethical considerations. Facial Plast Surg 2023;39:454-9.
4. Sacristán JA, Dilla T. No big data without small data: learning health care systems begin and end with the individual patient. J Eval Clin Pract 2015;21:1014-7.
5. Hume KM, Crotty CA, Simmons CJ, Neumeister MW, Chung KC. Medical specialty society-sponsored data registries: opportunities in plastic surgery. Plast Reconstr Surg 2013;132:159e-67e.
6. Davenport T, Kalakota R. The potential for artificial intelligence in healthcare. Future Healthc J 2019;6:94-8.
7. Phillips M, Marsden H, Jaffe W, et al. Assessment of accuracy of an artificial intelligence algorithm to detect melanoma in images of skin lesions. JAMA Netw Open 2019;2:e1913436.
8. Joshi G, Jain A, Araveeti SR, Adhikari S, Garg H, Bhandari M. FDA-approved artificial intelligence and machine learning (AI/ML)-enabled medical devices: an updated landscape. Electronics 2024;13:498.
9. Esteva A, Kuprel B, Novoa RA, et al. Dermatologist-level classification of skin cancer with deep neural networks. Nature 2017;542:115-8.
10. Koh DM, Papanikolaou N, Bick U, et al. Artificial intelligence and machine learning in cancer imaging. Commun Med 2022;2:133.
11. Barreiro-Ares A, Morales-Santiago A, Sendra-Portero F, Souto-Bayarri M. Impact of the rise of artificial intelligence in radiology: what do students think? Int J Environ Res Public Health 2023;20:1589.
12. Shin HJ, Han K, Ryu L, Kim EK. The impact of artificial intelligence on the reading times of radiologists for chest radiographs. NPJ Digit Med 2023;6:82.
13. Yacoub B, Varga-Szemes A, Schoepf UJ, et al. Impact of artificial intelligence assistance on chest CT interpretation times: a prospective randomized study. AJR Am J Roentgenol 2022;219:743-51.
14. Farid Y, Fernando Botero Gutierrez L, Ortiz S, et al. Artificial intelligence in plastic surgery: insights from plastic surgeons, education integration, ChatGPT’s survey predictions, and the path forward. Plast Reconstr Surg Glob Open 2024;12:e5515.
15. Duong TV, Vy VPT, Hung TNK. Artificial intelligence in plastic surgery: advancements, applications, and future. Cosmetics 2024;11:109.
16. Ho AL, Klassen AF, Cano S, Scott AM, Pusic AL. Optimizing patient-centered care in breast reconstruction: the importance of preoperative information and patient-physician communication. Plast Reconstr Surg 2013;132:212e-20e.
17. Browne R, Gull K, Hurley CM, Sugrue RM, O’Sullivan JB. ChatGPT-4 can help hand surgeons communicate better with patients. J Hand Surg Glob Online 2024;6:436-8.
18. Berry CE, Fazilat AZ, Churukian AA, et al. Quality assessment of online resources for gender-affirming surgery. Plast Reconstr Surg Glob Open 2023;11:e5306.
19. Baldwin AJ. An artificial intelligence language model improves readability of burns first aid information. Burns 2024;50:1122-7.
20. Berry CE, Fazilat AZ, Lavin C, et al. Both patients and plastic surgeons prefer artificial intelligence-generated microsurgical information. J Reconstr Microsurg 2024;40:657-64.
21. Grippaudo FR, Nigrelli S, Patrignani A, Ribuffo D. Quality of the information provided by ChatGPT for patients in breast plastic surgery: are we already in the future? JPRAS Open 2024;40:99-105.
22. Seth I, Cox A, Xie Y, et al. Evaluating chatbot efficacy for answering frequently asked questions in plastic surgery: a ChatGPT case study focused on breast augmentation. Aesthet Surg J 2023;43:1126-35.
23. Xie Y, Seth I, Hunter-Smith DJ, Rozen WM, Ross R, Lee M. Aesthetic surgery advice and counseling from artificial intelligence: a rhinoplasty consultation with ChatGPT. Aesthetic Plast Surg 2023;47:1985-93.
24. Chaker SC, Hung YC, Saad M, Golinko MS, Galdyn IA. Easing the burden on caregivers- applications of artificial intelligence for physicians and caregivers of children with cleft lip and palate. Cleft Palate Craniofac J 2024.
25. Sharma SC, Ramchandani JP, Thakker A, Lahiri A. ChatGPT in plastic and reconstructive surgery. Indian J Plast Surg 2023;56:320-5.
26. Altamimi I, Altamimi A, Alhumimidi AS, Altamimi A, Temsah MH. Artificial intelligence (AI) chatbots in medicine: a supplement, not a substitute. Cureus 2023;15:e40922.
27. Ahmed SK, Hussein S, Aziz TA, Chakraborty S, Islam MR, Dhama K. The power of ChatGPT in revolutionizing rural healthcare delivery. Health Sci Rep 2023;6:e1684.
28. Wang A, Kim E, Oleru O, Seyidova N, Taub PJ. Artificial intelligence in plastic surgery: ChatGPT as a tool to address disparities in health literacy. Plast Reconstr Surg 2024;153:1232e-4e.
29. Daraz L, Morrow AS, Ponce OJ, et al. Can patients trust online health information? A meta-narrative systematic review addressing the quality of health information on the internet. J Gen Intern Med 2019;34:1884-91.
30. Shahsavar Y, Choudhury A. User intentions to use ChatGPT for self-diagnosis and health-related purposes: cross-sectional survey study. JMIR Hum Factors 2023;10:e47564.
31. Fazilat AZ, Berry CE, Churukian A, et al. AI-based cleft lip and palate surgical information is preferred by both plastic surgeons and patients in a blind comparison. Cleft Palate Cran J 2024.
32. Boczar D, Sisti A, Oliver JD, et al. Artificial intelligent virtual assistant for plastic surgery patient’s frequently asked questions: a pilot study. Ann Plast Surg 2020;84:e16-21.
33. Soh CL, Shah V, Arjomandi Rad A, et al. Present and future of machine learning in breast surgery: systematic review. Br J Surg 2022;109:1053-62.
34. Mavioso C, Araújo RJ, Oliveira HP, et al. Automatic detection of perforators for microsurgical reconstruction. Breast 2020;50:19-24.
35. Kiranantawat K, Sitpahul N, Taeprasartsit P, et al. The first smartphone application for microsurgery monitoring: SilpaRamanitor. Plast Reconstr Surg 2014;134:130-9.
36. Myung Y, Jeon S, Heo C, et al. Validating machine learning approaches for prediction of donor related complication in microsurgical breast reconstruction: a retrospective cohort study. Sci Rep 2021;11:5615.
37. Hassan AM, Biaggi-Ondina A, Asaad M, et al. Artificial intelligence modeling to predict periprosthetic infection and explantation following implant-based reconstruction. Plast Reconstr Surg 2023;152:929-38.
38. Bennett SP, Fitoussi AD, Berry MG, Couturaud B, Salmon RJ. Management of exposed, infected implant-based breast reconstruction and strategies for salvage. J Plast Reconstr Aesthet Surg 2011;64:1270-7.
39. Zhang BH, Chen K, Lu SM, et al. Turning back the clock: artificial intelligence recognition of age reduction after face-lift surgery correlates with patient satisfaction. Plast Reconstr Surg 2021;148:45-54.
40. Boonipat T, Asaad M, Lin J, Glass GE, Mardini S, Stotland M. Using artificial intelligence to measure facial expression following facial reanimation surgery. Plast Reconstr Surg 2020;146:1147-50.
41. Geisler EL, Agarwal S, Hallac RR, Daescu O, Kane AA. A role for artificial intelligence in the classification of craniofacial anomalies. J Craniofac Surg 2021;32:967-9.
42. Knoops PGM, Papaioannou A, Borghi A, et al. A machine learning framework for automated diagnosis and computer-assisted planning in plastic and reconstructive surgery. Sci Rep 2019;9:13597.
43. Marcus G, Davis E, Aaronson S. A very preliminary analysis of DALL-E 2. arXiv. [Preprint.] May 2, 2022 [accessed on 2024 Sep 30]. Available from: https://doi.org/10.48550/arXiv.2204.13807.
44. Lim B, Seth I, Kah S, et al. Using generative artificial intelligence tools in cosmetic surgery: a study on rhinoplasty, facelifts, and blepharoplasty procedures. J Clin Med 2023;12:6524.
45. Bäcker HC, Wu CH, Strauch RJ. Systematic review of diagnosis of clinically suspected scaphoid fractures. J Wrist Surg 2020;9:81-9.
46. Ozkaya E, Topal FE, Bulut T, Gursoy M, Ozuysal M, Karakaya Z. Evaluation of an artificial intelligence system for diagnosing scaphoid fracture on direct radiography. Eur J Trauma Emerg Surg 2022;48:585-92.
47. Oeding JF, Kunze KN, Messer CJ, et al. Diagnostic performance of artificial intelligence for detection of scaphoid and distal radius fractures: a systematic review. J Hand Surg Am 2024;49:411-22.
48. Hoogendam L, Bakx JAC, Souer JS, Slijper HP, Andrinopoulou ER, Selles RW. Hand Wrist Study Group. Predicting clinically relevant patient-reported symptom improvement after carpal tunnel release: a machine learning approach. Neurosurgery 2022;90:106-13.
49. Loos NL, Hoogendam L, Souer JS, et al; the Hand-Wrist Study Group. Machine learning can be used to predict function but not pain after surgery for thumb carpometacarpal osteoarthritis. Clin Orthop Relat Res 2022;480:1271-84.
50. Kim J, Oh I, Lee YN, et al. Predicting the severity of postoperative scars using artificial intelligence based on images and clinical data. Sci Rep 2023;13:13448.
51. Squiers JJ, Thatcher JE, Bastawros DS, et al. Machine learning analysis of multispectral imaging and clinical risk factors to predict amputation wound healing. J Vasc Surg 2022;75:279-85.
53. Xue Y, Chen C, Tan R, et al. Artificial intelligence-assisted bioinformatics, microneedle, and diabetic wound healing: a “new deal” of an old drug. ACS Appl Mater Interfaces 2022;14:37396-409.
54. Chae MP, Rozen WM, McMenamin PG, Findlay MW, Spychal RT, Hunter-Smith DJ. Emerging applications of bedside 3D printing in plastic surgery. Front Surg 2015;2:25.
55. Knoops PGM, Borghi A, Ruggiero F, et al. A novel soft tissue prediction methodology for orthognathic surgery based on probabilistic finite element modelling. PLoS One 2018;13:e0197209.
56. Huff TJ, Ludwig PE, Zuniga JM. The potential for machine learning algorithms to improve and reduce the cost of 3-dimensional printing for surgical planning. Expert Rev Med Devices 2018;15:349-56.
57. Booth J, Roussos A, Zafeiriou S, Ponniah A, Dunaway D. A 3D morphable model learnt from 10,000 faces. In: 2016 IEEE Conference on Computer Vision and Pattern Recognition (CVPR); 2016 Jun 27-30; Las Vegas, USA. IEEE; 2016. pp. 5543-52.
58. Dai H, Pears N, Smith W, Duncan C. A 3D morphable model of craniofacial shape and texture variation. In: 2017 IEEE International Conference on Computer Vision (ICCV); 2017 Oct 22-29; Venice, Italy. IEEE; 2017. pp. 3104-12.
59. Goh GD, Sing SL, Yeong WY. A review on machine learning in 3D printing: applications, potential, and challenges. Artif Intell Rev 2021;54:63-94.
60. Menon A, Póczos B, Feinberg AW, Washburn NR. Optimization of silicone 3D printing with hierarchical machine learning. 3D Print Addit Manuf 2019;6:181-9.
61. Conev A, Litsa EE, Perez MR, Diba M, Mikos AG, Kavraki LE. Machine learning-guided three-dimensional printing of tissue engineering scaffolds. Tissue Eng Part A 2020;26:1359-68.
62. Chae MP, Hunter-Smith DJ, Spychal RT, Rozen WM. 3D volumetric analysis for planning breast reconstructive surgery. Breast Cancer Res Treat 2014;146:457-60.
63. Lei IM, Jiang C, Lei CL, et al. 3D printed biomimetic cochleae and machine learning co-modelling provides clinical informatics for cochlear implant patients. Nat Commun 2021;12:6260.
64. Asghari A, O’Connor MJ, Attalla P, et al. Game changers: plastic and reconstructive surgery innovations of the last 100 years. Plast Reconstr Surg Glob Open 2023;11:e5209.
65. Lao WWK, Hsieh TY, Ramirez AE. Differences and similarities between eastern and western rhinoplasty: features and proposed algorithms. Ann Plast Surg 2021;86:S259-64.
66. Mir MA, Maurya R. Precision and progress: machine learning advancements in plastic surgery. Cureus 2023;15:e41952.
67. Pool C, Moroco A, Lighthall JG. Utilizing virtual surgical planning and patient-specific cutting guides in microtia repair with autologous costal cartilage graft. Plast Reconstr Surg 2024;154:569e-72e.
68. O’Sullivan S, Leonard S, Holzinger A, et al. Operational framework and training standard requirements for AI‐empowered robotic surgery. Int J Med Robot 2020;16:1-13.
Cite This Article
How to Cite
Brenac, C.; Fazilat, A. Z.; Fallah, M.; Kawamoto-Duran, D.; Sunwoo, P. S.; Longaker, M. T.; Wan, D. C.; Guo, J. L. AI in plastic surgery: customizing care for each patient. Art. Int. Surg. 2024, 4, 296-315. http://dx.doi.org/10.20517/ais.2024.49
Download Citation
Export Citation File:
Type of Import
Tips on Downloading Citation
Citation Manager File Format
Type of Import
Direct Import: When the Direct Import option is selected (the default state), a dialogue box will give you the option to Save or Open the downloaded citation data. Choosing Open will either launch your citation manager or give you a choice of applications with which to use the metadata. The Save option saves the file locally for later use.
Indirect Import: When the Indirect Import option is selected, the metadata is displayed and may be copied and pasted as needed.
About This Article
Special Issue
Copyright
Data & Comments
Data
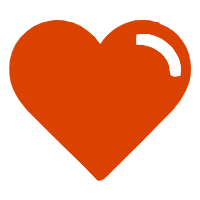
Comments
Comments must be written in English. Spam, offensive content, impersonation, and private information will not be permitted. If any comment is reported and identified as inappropriate content by OAE staff, the comment will be removed without notice. If you have any queries or need any help, please contact us at support@oaepublish.com.