Pseudarthrosis following adult spinal deformity surgery may be predicted with preoperative MRI adipose tissue features: an artificial intelligence study on raw 3D imaging
Abstract
Aim: The purpose of this study is to investigate the utility of incorporating magnetic resonance imaging (MRI) into an artificial intelligence (AI) model to preoperatively predict pseudarthrosis for patients undergoing adult spinal deformity (ASD) surgery.
Methods: A retrospective cohort study was conducted on patients undergoing ASD surgery at Vanderbilt University Medical Center with at least 2 years of follow-up. We first collected demographic variables and measured traditional radiographic variables with Surgimap software. The primary outcome of interest was pseudarthrosis, defined as mechanical pain without evidence of bony union with or without a rod fracture. Next, cohort differences between patients diagnosed with and without pseudarthrosis were evaluated with t-tests for continuous variables and chi-squared tests for categorical variables using Bonferroni-Holm multiple comparison correction. Using a subpopulation of patients with preoperative thoracic MRI available, a three-dimensional convolutional neural network (3D-CNN) with five-fold nested cross-validation was developed to predict pseudarthrosis - accuracy was evaluated with the Youden index. Finally, class activation mapping (CAM) was conducted to visualize the MRI features utilized by the model for accurate classifications.
Results: Of 191 patients undergoing ASD surgery, the demographic and traditional radiographic variables were collected, and only age was observed to be significantly different between the patients diagnosed with pseudarthrosis (69.9 ± 10.1 years old) and those without (60.9 ± 19.9), with a t-test P-value of 0.003. The 3D-CNN demonstrated an average Youden index of 0.49 ± 0.25 on the withheld data, with a P-value of 5.50e-3 compared to an equivocal null model. Finally, CAM consistently revealed posterior adipose tissue to be most important in preoperatively predicting pseudarthrosis.
Conclusion: Adipose tissue features in MRI, independent of body mass index (BMI), may be useful for preoperatively predicting pseudarthrosis. Overall, this work demonstrates the capabilities of raw imaging AI in spine surgery and can serve as the basis for a deeper biological inquiry into the pathogenesis of pseudarthrosis.
Keywords
INTRODUCTION
Pseudarthrosis (or nonunion) is defined as the failure of bone to fuse following surgical fixation and is a common complication of adult spinal deformity (ASD) surgery, with incidence rates ranging from 5%-35%[1,2]. Pseudarthrosis is associated with recurrent pain and neurologic symptoms, can be a reason for reoperation, and can occur with or without rod fracture[3]. Despite its prevalence and contribution to patient morbidity, the risk factors for pseudarthrosis are difficult to characterize. A preoperative risk factor is thought to be age, with multiple studies suggesting that patients over the age of 55 experience higher rates of pseudarthrosis[4-6]. Additionally, an intraoperative risk factor is thought to be fusion to the sacrum[7]. However, there remains debate in the literature about these risk factors, and few validated tools are available for the surgeon to preoperatively prognosticate pseudarthrosis occurrence.
Due to the difficulty in prognostication, more advanced artificial intelligence (AI) modeling techniques have been developed to augment surgical decision workflows for ASD surgery[8-16]. Specifically, Scheer et al. developed a decision tree model from 82 variables that achieved 91% accuracy in predicting pseudarthrosis following ASD surgery[17]. This high level of accuracy demonstrates its promise for clinical application. An underutilized extension of this framework is to utilize raw imaging data to augment predictive models. Of interest, AI models that ingest raw imaging can be directly interpreted to gain insight into nuanced patient characteristics impossible to capture in demographic variables. One imaging modality of high interest is magnetic resonance imaging (MRI) due to the detailed soft tissue signal captured. Thus, these advanced imaging models can aid preoperative decision-making, but more importantly, they can provide insight into the biological variables that may drive pseudarthrosis pathogenesis.
With the above considerations, this work aims to characterize the raw preoperative MRI features that may predict the occurrence of pseudarthrosis. In a cohort of patients undergoing ASD surgery at the major academic medical center, we sought to: (1) develop an AI model that utilizes raw preoperative MRI to predict pseudarthrosis following ASD surgery; and (2) interpret the model with class activation mapping (CAM) to understand the imaging features used to classify pseudarthrosis.
METHODS
Patient population
The study included a population of 191 patients who underwent ASD surgery at a single institution from 2009-2021 and had at least 2-year follow-up. A subpopulation of 59 patients had presurgical thoracic MRI available for raw imaging deep learning analysis. The electronic medical record was mined for demographic variables outlined in Table 1. Pseudarthrosis was defined with a combination of clinical semiology and radiographic evidence of fusion failure captured on coronal and sagittal computed tomography (CT) scan, with or without rod fracture. Every symptomatic rod fracture in our series was given a diagnosis of pseudarthrosis as well. Next, each patient’s scoliosis radiographs were de-identified and processed with Surgimap v2.3.2.1 (Nemaris Inc, Methuen, Massachusetts, USA) to acquire traditional radiographic measurements [Table 2]. To evaluate any correlation between the demographic/radiographic variables and pseudarthrosis incidence, two-population t-tests for continuous variables and chi-squared tests for categorical variables were conducted with Bonferroni-Holm multiple comparison correction.
Demographic and surgical variables by pseudarthrosis
Demographic and surgical variables | Total cohort (N = 191) | No Pseud. (N = 143) | Pseud. (N = 48) | P-value |
Age at surgery, mean ± SD | 63.1 ± 18.4 | 60.9 ± 19.9 | 69.9 ± 10.1 | 0.003 |
BMI, mean ± SD | 28.8 ± 7.0 | 28.8 ± 7.3 | 29.0 ± 8.2 | 0.874 |
Gender female, n (%) | 146 (76.4) | 108 (75.5) | 38 (79.2) | 0.607 |
Comorbidities, n (%) | ||||
Diabetes | 26 (13.6) | 15 (10.5) | 11 (22.9) | 0.030 |
COPD | 48 (25.1) | 32 (22.4) | 16 (33.3) | 0.130 |
Heart failure | 24 (12.6) | 16 (11.2) | 8 (16.7) | 0.322 |
Hypertension | 122 (63.9) | 86 (60.1) | 36 (75.0) | 0.064 |
Osteoporosis | 40 (20.9) | 32 (22.4) | 8 (16.7) | 0.400 |
Surgical variables | ||||
Previous fusion, n (%) | 56 (29.3) | 44 (30.8) | 12 (25.0) | 0.447 |
Pelvic fixation, n (%) | 150 (78.5) | 106 (74.1) | 44 (91.7) | 0.010 |
TIL, mean ± SD | 10.6 ± 3.0 | 10.4 ± 3.1 | 10.2 ± 3.0 | 0.697 |
UIV Region, n (%) | ||||
Upper thoracic | 71 | 57 | 14 | - |
Thoracolumbar | 120 | 86 | 34 | 0.185 |
Traditional radiographic measurements by pseudarthrosis
Radiographic measurements degrees mean ± SD (unless otherwise noted) | Total cohort (N = 191) | No pseud. (N = 143) | Pseud. (N = 48) | P-value |
Coronal measurements | ||||
C7PL, mm | 2.2 ± 36.3 | 2.4 ± 34.9 | 1.6 ± 40.4 | 0.898 |
Major curve apex deviation, mm | -2.1 ± 34.5 | -1.8 ± 34.6 | -3.3 ± 34.4 | 0.804 |
Major curve cobb angle | -4.0 ± 34.4 | -3.8 ± 34.2 | -4.6 ± 35.0 | 0.885 |
Small curve apex deviation, mm | 0.3 ± 18.0 | -0.4 ± 17.5 | 2.6 ± 19.3 | 0.34 |
Small curve cobb angle | 3.6 ± 24.7 | 3.4 ± 24.4 | 4.2 ± 25.8 | 0.848 |
T1 tilt | 0.8 ± 6.3 | 1.1 ± 6.4 | -0.4 ± 6.1 | 0.156 |
Thoracic curve apex deviation, mm | -0.3 ± 10.2 | -0.0 ± 9.5 | -1.2 ± 12.3 | 0.585 |
Thoracic curve cobb angle | -0.9 ± 16.0 | -0.9 ± 16.1 | -1.0 ± 15.4 | 0.979 |
Sagittal measurements | ||||
C2 slope | 18.1 ± 13.8 | 17.9 ± 13.0 | 18.8 ± 15.9 | 0.688 |
CL | 9.2 ± 15.8 | 9.3 ± 16.5 | 8.6 ± 13.7 | 0.783 |
CPA | 28.1 ± 14.4 | 26.9 ± 14.6 | 31.8 ± 13.2 | 0.043 |
CTPA | 2.9 ± 1.6 | 3.0 ± 1.5 | 2.7 ± 2.0 | 0.312 |
L1-L4 angle | -7.0 ± 19.0 | -8.7 ± 19.1 | -2.0 ± 17.9 | 0.033 |
L1-S1, mm | 175.7 ± 22.0 | 177.4 ± 20.7 | 170.5 ± 24.7 | 0.062 |
L1PA | 12.0 ± 11.5 | 11.5 ± 11.7 | 13.9 ± 10.7 | 0.251 |
L4-S1 angle | -30.7 ± 15.6 | -30.7 ± 14.4 | -30.7 ± 18.8 | 0.995 |
LL | 32.4 ± 24.8 | 34.2 ± 22.9 | 26.9 ± 29.0 | 0.076 |
PI | 53.5 ± 16.7 | 53.4 ± 16.5 | 53.9 ± 17.0 | 0.847 |
PI-LL | 20.4 ± 22.8 | 19.2 ± 23.2 | 24.0 ± 21.2 | 0.208 |
PT | 25.5 ± 12.0 | 24.6 ± 12.4 | 28.0 ± 10.2 | 0.093 |
SS | 28.0 ± 14.1 | 28.7 ± 13.7 | 25.9 ± 14.8 | 0.225 |
C2-C7 cSVA, mm | 29.2 ± 14.7 | 29.2 ± 13.4 | 29.1 ± 18.1 | 0.965 |
C7-S1 SVA, mm | 70.6 ± 69.3 | 65.1 ± 66.7 | 86.9 ± 74.2 | 0.061 |
T1SPI | -0.3 ± 6.8 | -0.8 ± 6.3 | 1.1 ± 8.1 | 0.099 |
T1 slope | 27.7 ± 11.0 | 27.6 ± 10.9 | 28.1 ± 11.2 | 0.771 |
T1-CL | 18.6 ± 13.3 | 18.3 ± 12.7 | 19.5 ± 15.1 | 0.572 |
T1-L1, mm | 306.9 ± 34.5 | 306.0 ± 35.0 | 309.6 ± 32.6 | 0.54 |
T1-S1, mm | 483.1 ± 44.0 | 483.4 ± 44.9 | 482.2 ± 41.1 | 0.871 |
T2-T5 angle | 9.8 ± 10.2 | 10.5 ± 10.0 | 7.6 ± 10.4 | 0.085 |
T5-T12 angle | 26.5 ± 18.4 | 26.2 ± 18.3 | 27.6 ± 18.5 | 0.654 |
T9SPI | 10.0 ± 7.4 | 10.0 ± 7.2 | 9.9 ± 8.2 | 0.925 |
TK | 30.7 ± 19.7 | 30.1 ± 19.3 | 32.5 ± 20.8 | 0.469 |
TL | 11.8 ± 18.1 | 10.1 ± 18.3 | 16.9 ± 16.7 | 0.026 |
TPA | 25.2 ± 14.3 | 23.8 ± 14.6 | 29.1 ± 12.7 | 0.028 |
MRI deep learning
Next, a three-dimensional convolutional neural network (3D-CNN) was developed to input raw thoracic MRIs, demographic variables, and Surgimap measured variables [Figure 1][18]. Only patients with MRI available were included in this study. MRI images were resliced to the three dimensions of 256 × 256 × 20 voxels, histogram equalized, and augmented using random flips, noise, bias field, blur, and affine/elastic deformations to a total of 1,080 images. Five-fold nested cross-validation with a train/validate/test split ratio of 70%/20%/10% was used to prevent overfitting and evaluate the generalizability of the model[19]. Importantly, all splits were conducted at the patient level. A Youden index (sensitivity + specificity - 100%) was calculated for all completely withheld test partitions for each fold. The Youden index reflects the true positive, true negative, false positive, and false negative rate of the model on completely withheld validation data. The mean Youden index of all folds was tested against an equivocal null model with a Youden index value of 0 using a single population Student’s t-test[20].
Imaging feature attention mapping
Finally, to interpret the model and elucidate MRI features used for correct classification, the CNN architecture was modified to accommodate gradient class activation mapping (Grad-CAM)[21]. This technique highlights regions of the raw image that were important for classification (“hotspots”, Figure 1). The MRI hotspots were then qualitatively analyzed across the cohort to infer important tissue types for accurate prediction of pseudarthrosis.
RESULTS
Demographic variables poorly correlate with pseudarthrosis
In our cohort of 191 patients who underwent ASD surgery, 48 (25.1%) had pseudarthrosis compared to 143 (74.9%) who did not, and 37 (19.4%) overall developed rod fracture - consistent with previously reported cohorts[22]. Specifically, rod fractures were seen in 29 (60.4%) of the pseudarthrosis. All pseudarthrosis required reoperation. Upon demographic analysis, we only observed age to be significantly different between patients who were diagnosed with pseudarthrosis (69.9 ± 10.1 years old) versus those without (60.9 ± 19.9), with a P-value of 0.003. Furthermore, the radiographic variables captured by Surgimap were not observed to be significantly different between the groups after multiple comparison corrections. Please see Tables 1 and 2 for the full extent of preoperative variables considered. Thus, in alignment with past literature, the occurrence of pseudarthrosis did not exhibit a distinct demographic or traditional radiographic signature.
Pseudarthrosis can be predicted with raw preoperative MRI
To explore the potential of AI to non-linearly utilize the millions of data points present in raw MRI, we implemented a custom 3D-CNN. Across the five-fold nested cross-validation, the completely withheld testing data were classified by the model with a Youden index ranging from 0.30 to 0.80 (mean 0.49, 95% confidence interval ± 0.25, Figure 2). A single population t-test against a null hypothesis of a Youden index of 0.00, representing an equivocal model, was significant with a P-value of 5.50e-3. These results indicate that the 3D-CNN model was accurate in predicting pseudarthrosis following ASD surgery with at least two years of follow-up and has the potential to generalize well to a larger population.
Superficial adipose tissue appears to be most important for classification
Of greatest interest to this work were the MRI features used by the 3D-CNN model to gain insight into the pathogenesis of pseudarthrosis. Upon model interrogation with GradCAM, the most important MRI features for classification of pseudarthrosis following ASD surgery appear to be posterior adipose tissue - i.e., the majority of GradCAM feature maps highlight various aspects of superficial adipose tissue posterior to the spinous processes (example subjects in Figure 3). Notably, there is no significant difference in body mass index (BMI) between the pseudarthrosis cohort (28.8 ± 7.3) and the non-pseudarthrosis cohort (29.0 ± 8.2), with a t-test P-value of 0.874. Notably, of the 48 patients who developed pseudarthrosis, 24 (50.0%) also had proximal junctional kyphosis (PJK). However, in our past work, we found that posterior musculature was most predictive of PJK[18]. Thus, these results indicate that there is an important radiologic signature within these adipose regions that enables the 3D-CNN model to accurately classify pseudarthrosis, independent of total adipose content estimated by BMI and independent of radiographic features that predict PJK.
DISCUSSION
The current study demonstrated the accuracy of using a 3D-CNN on raw thoracic MRI to predict pseudarthrosis following ASD surgery. More importantly, the imaging features associated with pseudarthrosis were elucidated to be mainly posterior adipose tissue - with a predominance of the upper thoracic region. Interestingly, except for age, our cohort did not demonstrate any demographic or traditional radiographic measurement difference between those who developed pseudarthrosis and those who did not. Thus, it is noteworthy that the 3D-CNN heavily utilized adipose tissue of the imaging to develop the classification despite the pseudarthrosis cohort not being significantly more overweight (P = 0.874). This observation leads the authors to surmise that there exists a subtle MRI signature in the adipose tissue that the model used for classification. Furthermore, the imaging hotspots are not consistently at a region of the largest adipose collection; thus, it is likely that the 3D-CNN model is detecting an intra-adipose or adipose-adjacent signal. Future work could focus on using image segmentation techniques to better quantify the exact types of tissue present within GradCAM hotspots. Finally, posterior upper thoracic adipose tissue is typically distant from the region of pseudarthrosis, which in our cohort was predominately in the low lumbar region. Thus, it can be surmised that the network learned a global signature of pseudarthrosis predilection, not captured by demographic variables, as opposed to a local tissue abnormality at the future site of pseudarthrosis.
Overall, this work serves as an augmentation to existing models to preoperatively predict pseudarthrosis[17]. Previously identified risk factors to predict pseudarthrosis include alcoholism, smoking, fusion location, vertebral bone quality score, diabetes, sarcopenia, advanced age, and potentially graft material[1,23-26]. Not all of these factors were readily available in our dataset. However, it is notable that our demographic analysis of available metrics did not reveal any significant differences between pseudarthrosis and non-pseudarthrosis cohorts except for age. Thus, by incorporating raw MRI, one can leverage high-dimensional data that are often readily available in clinical databases with minimal manual extraction required. With the incorporation of readily available imaging, this technique does not rely on meticulous database mining and is free from the problems of patient bias when reporting metrics such as alcohol use and smoking status. Furthermore, this work provides potential insight into the biological underpinnings of pseudarthrosis development and could serve as background evidence for future studies exploring the role of global adipose tissue characteristics in those who develop pseudarthrosis.
It is well-documented that posterior musculature and sarcopenia appear to be strongly correlated with mechanical complications following ASD surgery[27]. Thus, it is notable that the current work, and our past work using similar techniques to predict PJK using MRI have both revealed imaging risk factors of soft tissue - adipose tissue and posterior musculature, respectively. This contributes to the growing body of literature focusing on soft tissue characteristics as driving factors for mechanical complications, as opposed to bony anatomy. The authors do not discount the importance of bone integrity for consideration of ASD surgery, but rather aim to outline the additional importance of soft tissue health when considering a large deformity operation.
Limitations to this technique include the ever-present potential for overfitting, the computing resources required, and the technical expertise required to run the analysis algorithms. An important next step would be to test these methods on an external cohort. Another consideration with this methodology is that the thoracic MRI did not capture the top of the implanted construct in a few subjects. This can be seen as both a potential weakness and potential strength of this study because the results were robust despite this consideration - this indicates that there is possibly a global imaging feature that the 3D-CNN detects to aid accurate classification. Finally, the proper de-identification of raw data is paramount to model creation to ensure patient privacy when deploying trained models.
Overall, the use of machine learning in medical imaging has garnered attention but has still been limited in scope compared to tabular data machine learning and large language models. We aimed to demonstrate the potential of a simple classification scheme on available 3D MRIs to predict the development of pseudarthrosis following ASD surgery. Beyond the cross-validated accuracy of the model, our approach has the benefit of providing a level of interpretation by outlining imaging features used by the model to make classification decisions. Overall, this work demonstrates the capabilities of raw imaging AI in spine surgery and can serve as the basis for a deeper biological inquiry into the pathogenesis of pseudarthrosis.
DECLARATIONS
Authors’ contributions
Conceptualization, data acquisition, data analysis, results interpretation, and manuscript preparation: Johnson GW, Chanbour H
Conceptualization, data analysis, results interpretation, and manuscript preparation: Doss DJ
Conceptualization, results interpretation, and manuscript preparation: Makhoul GS
Conceptualization, data acquisition, data analysis, results interpretation, and manuscript preparation: Abtahi AM, Stephens BF, Zuckerman SL
Availability of data and materials
All data and code are available upon reasonable request to the corresponding author.
Financial support and sponsorship
None.
Conflicts of interest
Stephens BF receives institutional research support from Nuvasive and Stryker Spine. Zuckerman SL reports being an unaffiliated neurotrauma consultant for the National Football League. Abtahi AM receives institutional research support from Stryker Spine. The other authors declared that there are no conflicts of interest.
Ethical approval and consent to participate
All procedures performed in studies involving human participants were in accordance with the ethical standards of the institutional and/or national research committee and with the 1964 Helsinki Declaration and its later amendments or comparable ethical standards. IRB: Approval Attained (#211290).
Consent for publication
Not applicable.
Copyright
© The Author(s) 2024.
REFERENCES
1. How NE, Street JT, Dvorak MF, et al. Pseudarthrosis in adult and pediatric spinal deformity surgery: a systematic review of the literature and meta-analysis of incidence, characteristics, and risk factors. Neurosurg Rev 2019;42:319-36.
2. Deyo RA, Mirza SK, Martin BI, Kreuter W, Goodman DC, Jarvik JG. Trends, major medical complications, and charges associated with surgery for lumbar spinal stenosis in older adults. JAMA 2010;303:1259-65.
3. Buchholz AL, Quinn JC, Shaffrey CI. Postoperative spinal deformities: kyphosis, nonunion, and loss of motion segment. In: Complications in neurosurgery. 2019. pp. 325-30. Available from: http://103.203.175.90:81/fdScript/RootOfEBooks/E%20Book%20Collection%202021%20-%20A/ENGLISH/Complications%20in%20Neurosurgery.pdf. [Last accessed on 7 Nov 2024].
4. Kim YJ, Bridwell KH, Lenke LG, Rhim S, Cheh G. Pseudarthrosis in long adult spinal deformity instrumentation and fusion to the sacrum: prevalence and risk factor analysis of 144 cases. Spine 2006;31:2329-36.
5. Kim YJ, Bridwell KH, Lenke LG, Rinella AS, Edwards C 2nd. Pseudarthrosis in primary fusions for adult idiopathic scoliosis: incidence, risk factors, and outcome analysis. Spine 2005;30:468-74.
6. Kim YJ, Bridwell KH, Lenke LG, Cho KJ, Edwards CC 2nd, Rinella AS. Pseudarthrosis in adult spinal deformity following multisegmental instrumentation and arthrodesis. J Bone Joint Surg Am 2006;88:721-8.
7. Pateder DB, Park YS, Kebaish KM, et al. Spinal fusion after revision surgery for pseudarthrosis in adult scoliosis. Spine 2006;31:E314-9.
8. Joshi RS, Haddad AF, Lau D, Ames CP. Artificial intelligence for adult spinal deformity. Neurospine 2019;16:686-94.
9. Joshi RS, Lau D, Ames CP. Artificial intelligence for adult spinal deformity: current state and future directions. Spine J 2021;21:1626-34.
10. Lenke LG. Commentary: artificial intelligence for adult spinal deformity. Neurospine 2019;16:695-6.
11. Perez-Breva L, Shin JH. Artificial intelligence in neurosurgery: a comment on the possibilities. Neurospine 2019;16:640-2.
12. Durand WM, Lafage R, Hamilton DK, et al; International Spine Study Group (ISSG). Artificial intelligence clustering of adult spinal deformity sagittal plane morphology predicts surgical characteristics, alignment, and outcomes. Eur Spine J 2021;30:2157-66.
13. Durand WM, Daniels AH, Hamilton DK, et al; International Spine Study Group. Artificial intelligence models predict operative versus nonoperative management of patients with adult spinal deformity with 86% accuracy. World Neurosurg 2020;141:e239-53.
14. Scheer JK, Smith JS, Schwab F, et al; International Spine Study Group. Development of a preoperative predictive model for major complications following adult spinal deformity surgery. J Neurosurg Spine 2017;26:736-43.
15. Scheer JK, Osorio JA, Smith JS, et al; International Spine Study Group. Development of validated computer-based preoperative predictive model for proximal junction failure (PJF) or clinically significant PJK with 86% accuracy based on 510 ASD patients with 2-year follow-up. Spine 2016;41:E1328-35.
16. Pellisé F, Serra-Burriel M, Smith JS, et al; International Spine Study Group, European Spine Study Group. Development and validation of risk stratification models for adult spinal deformity surgery. J Neurosurg Spine 2019;31:587-99.
17. Scheer JK, Oh T, Smith JS, et al; International Spine Study Group. Development of a validated computer-based preoperative predictive model for pseudarthrosis with 91% accuracy in 336 adult spinal deformity patients. Neurosurg Focus 2018;45:E11.
18. Johnson GW, Chanbour H, Ali MA, et al. Artificial intelligence to preoperatively predict proximal junction kyphosis following adult spinal deformity surgery: soft tissue imaging may be necessary for accurate models. Spine 2023;48:1688-95.
19. Kernbach JM, Staartjes VE. Foundations of machine learning-based clinical prediction modeling: part II - generalization and overfitting. In: Staartjes VE, Regli L, Serra C, editors. Machine learning in clinical neuroscience. Springer, Cham; 2022. pp. 15-21.
21. Selvaraju RR, Cogswell M, Das A, Vedantam R, Parikh D, Batra D. Grad-CAM: visual explanations from deep networks via gradient-based localization. Int J Comput Vis 2020;128:336-59.
22. Adogwa O, Buchowski JM, Lenke LG, et al. Comparison of rod fracture rates in long spinal deformity constructs after transforaminal versus anterior lumbar interbody fusions: a single-institution analysis. J Neurosurg Spine 2020;32:42-9.
23. Passias PG, Bortz C, Alas H, et al. Alcoholism as a predictor for pseudarthrosis in primary spine fusion: an analysis of risk factors and 30-day outcomes for 52,402 patients from 2005 to 2013. J Orthop 2019;16:36-40.
24. Marques MF, Fiere V, Obeid I, et al; Société Française de Chirurgie Rachidienne, SFCR. Pseudarthrosis in adult spine deformity surgery: risk factors and treatment options. Eur Spine J 2021;30:3225-32.
25. Kawabata A, Yoshii T, Sakai K, et al. Identification of predictive factors for mechanical complications after adult spinal deformity surgery: a multi-institutional retrospective study. Spine 2020;45:1185-92.
26. Kuo CC, Soliman MAR, Aguirre AO, et al. Vertebral bone quality score independently predicts proximal junctional kyphosis and/or failure after adult spinal deformity surgery. Neurosurgery 2023;92:945-54.
Cite This Article
How to Cite
Johnson, G. W.; Chanbour, H.; Doss, D. J.; Makhoul, G. S.; Abtahi, A. M.; Stephens, B. F.; Zuckerman, S. L. Pseudarthrosis following adult spinal deformity surgery may be predicted with preoperative MRI adipose tissue features: an artificial intelligence study on raw 3D imaging. Art. Int. Surg. 2024, 4, 401-10. http://dx.doi.org/10.20517/ais.2024.40
Download Citation
Export Citation File:
Type of Import
Tips on Downloading Citation
Citation Manager File Format
Type of Import
Direct Import: When the Direct Import option is selected (the default state), a dialogue box will give you the option to Save or Open the downloaded citation data. Choosing Open will either launch your citation manager or give you a choice of applications with which to use the metadata. The Save option saves the file locally for later use.
Indirect Import: When the Indirect Import option is selected, the metadata is displayed and may be copied and pasted as needed.
About This Article
Special Issue
Copyright
Data & Comments
Data
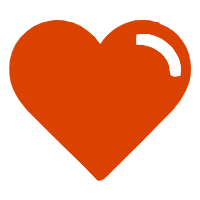
Comments
Comments must be written in English. Spam, offensive content, impersonation, and private information will not be permitted. If any comment is reported and identified as inappropriate content by OAE staff, the comment will be removed without notice. If you have any queries or need any help, please contact us at support@oaepublish.com.