Navigating artificial intelligence in spine surgery: implementation and optimization across the care continuum
Abstract
The field of spine surgery has long been characterized by innovations and technological advancements. The integration of artificial intelligence (AI) into spine surgery represents one of the latest technical developments in the field. The ability of AI to rapidly analyze datasets improves decision making, risk assessment, intraoperative precision, and postoperative management, all of which contribute to increasing personalized spine care and improving outcomes. However, the successful implementation of AI faces regulatory and privacy challenges that must be addressed before its full potential can be realized. Here, we provide a detailed analysis of the current applications and future prospects of AI in spine surgery, highlighting both the opportunities and challenges in this evolving field.
Keywords
INTRODUCTION
Artificial intelligence (AI) is increasingly being adapted for healthcare purposes, including analyzing complex data, identifying patterns, and making predictions and decisions [Supplementary Table 1][1,2]. A new frontier in AI has emerged with the genesis of generative AI models, which can create new content, including text and images, based on input data. These models could be leveraged to generate tailored surgical plans, create patient education packets, and assist in clinical documentation. Estimates project that AI solutions could potentially save up to $360 billion dollars of US healthcare expenditure (5%-10%) annually[3]. Here, we provide a comprehensive perspective on the existing applications as well as the frontiers and challenges for AI integration in spine surgery, including preoperative planning, intraoperative care, and postoperative management.
PREOPERATIVE PLANNING AND PATIENT SELECTION
Imaging analysis
AI algorithms excel at accurately analyzing spine imaging data, enabling the detection and characterization of pathologies with remarkable precision. For example, deep learning models have been developed for the automated detection of vertebral compression fractures on computed tomography (CT) or magnetic resonance imaging (MRI) scans[4]. Al Arif et al. used a training set of 138 X-rays and a test set of 172 images to identify vertebral centers and outlines with an average error of only 1.81 and 1.69 mm, respectively[5]. Doerr et al. used a region-based convolutional neural network to train and validate a deep learning model that can predict and classify a patient’s thoracolumbar trauma based on CT imaging alone, reducing the need to pursue additional costly and time-consuming MRI imaging for assessment[6]. AI can thereby help rapidly identify and triage patients in emergent settings and expedite the time to surgical intervention[7].
Risk stratification and surgical planning
Machine learning algorithms can predict the risk of complications, such as surgical site infections, venous thromboembolism, and reoperation, during and after spinal procedures[8-13]. Pellisé et al. utilized data from 1,612 patients across two independent prospective databases on adult spinal deformity to develop prognostic models for major complications, readmissions, and reoperations. The models can be used preoperatively to identify patients at greatest risk of postoperative complications and improve the patient counseling process[14].
While AI is still in its early stages, it has shown significant potential when trained on robust and extensive retrospective data. For example, machine learning algorithms have shown that they can outperform surgeons’ gestalt in predicting the risk of complications after emergency general surgery, including mortality, bleeding, and pneumonia[15]. AI-driven predictive risk models can also incorporate biopsychosocial patient factors including demographics, comorbidities, frailty, laboratory values, and imaging data, as well as surgical details including approach, spinal levels, and instrumentation - all of which are critical cues in spine surgery[16-18]. For instance, Goedmakers et al. developed a deep learning algorithm to predict adjacent segment disease following anterior cervical discectomy and fusion surgery, using only preoperative cervical MRI scans. The algorithm achieved a 95% accuracy rate, significantly outperforming expert neurosurgeons and neuroradiologists, who achieved only 58% accuracy[19].
Patient engagement and education
Large language models can simplify the reading levels of consent forms from a collegiate level to a seventh-grade level, allowing for more patient accessibility and understanding[20,21]. Moreover, AI-powered virtual assistants and chatbots can be harnessed as valuable care companions capable of providing knowledge to patients on demand[22,23]. Boczar et al. created an AI-powered virtual assistant that correctly answered 92% of patient questions regarding plastic surgery in a sample of 30 participants and 294 questions[24]. AI systems can also provide personalized education materials, track patient-reported outcomes, and monitor adherence to preoperative instructions, without requiring additional appointments or an advanced understanding of medical terminology[22,23]. However, caution must be taken to ensure that patients are not being misinformed by these systems.
Furthermore, AI systems, leveraging tools such as natural language processing, could also be integrated into clinic settings to automate patient intake[25]. Such “virtual scribes” can automatically generate comprehensive clinical notes and summarize patient-provider interactions, reducing administrative burden. Such safeguards include regularly updating the AI’s knowledge base to ensure it reflects the latest clinical guidelines, implementing clear disclaimers that patients are interacting with a virtual assistant rather than a clinician, and ensuring the AI defers to human oversight in complex or ambiguous cases.
Expanding the diagnostic armamentarium
Beyond the analysis of static imaging data, AI holds significant potential to enhance spinal diagnostics by incorporating dynamic and longitudinal patient characteristics. Machine learning models and video capture tools have been used to identify abnormal gait and compensation patterns and estimate biomechanical variables, such as joint loading and range of motion, that are not easily discernible through traditional clinical evaluation[26,27]. By integrating this dynamic data, clinicians can gain deeper insights into the underlying causes of spinal disorders and tailor interventions accordingly.
INTRAOPERATIVE SPINE CARE
Navigation and surgical accuracy
AI technologies can significantly enhance the spine operative experience. For example, surgical navigation systems powered by AI can seamlessly integrate with robotics to offer real-time guidance during complex spinal procedures[28]. While the regulatory burden remains high, this is due to the need for rigorous validation to ensure patient safety and efficacy in high-stakes environments. The justification for adopting these technologies lies in their potential to greatly improve surgical precision, reduce complications, and enhance patient outcomes, which outweighs the hurdles posed by regulatory requirements. Future applications of AI can enhance existing navigation systems, allowing for minimized intraoperative errors and surgical risk via real-time corrections to unexpected changes[29].
AI can also simulate procedures for educational training and create individualized models based on patient imaging. These models allow trainees to practice surgery on complex anatomical variations, serving as a powerful training tool[30-33]. In addition to benefitting trainees and health professionals, these models help patients better understand their conditions, facilitating more informed discussions about treatment options and fostering engagement in the decision-making process.
Intraoperative documentation
In the operating room, AI techniques can streamline documentation. In plastic surgery, ChatGPT templates have been shown to generate operative notes over 42 times faster than traditional methods[34]. Furthermore, with integration into billing and insurance information, AI can streamline the prior authorization process by extracting and organizing patient information, reducing delays in patient care. Zaidat et al. demonstrated a class-by-class accuracy between 77%-87% using a dataset of 902 operative notes of spine patients to generate billing codes[35]. While AI has made tremendous progress in improving administrative efficiency, it still faces challenges with redundancy, inaccuracy, and hallucinations. Regular audits and human oversight are essential to prevent these errors.
POSTOPERATIVE MONITORING AND REHABILITATION
AI continues to play a vital role in the postoperative phase, facilitating efficient recovery and optimizing patient outcomes through solutions such as personalized rehabilitation plans[36]. Lee et al. showed that an AI-based real-time motion feedback system improved strength and engagement during rehabilitation in spinal cord injury patients[37]. Similarly, models have been applied to identify patients who may need prolonged postoperative opioid prescriptions. Karhade et al. trained numerous models on a database of 5,413 patients and accurately predicted sustained postoperative opioid dependence between 90 and 180 days[38].
Leveraging longitudinal patient data, including clinical outcomes, activity levels, and patient-reported measures, AI can predict the trajectory of spinal conditions as well as the risk of complications or disease progression[39,40]. These predictive models can help identify high-risk patients, optimize surgical indications, and guide proactive management strategies[41].
FUTURE FRONTIERS IN SPINE CARE DATA OPTIMIZATION AND ANNOTATION
With patients generating gigabytes of data, the sheer volume presents challenges to clinicians. AI technologies can facilitate the interpretation of high-quality, structured data from diverse sources within the clinical environment, making them readily available for further analysis[42]. AI tools are also particularly adept at extracting relevant data from large, unstructured datasets, a common challenge in medical settings. When discussing the performance of AI models, metrics such as precision, recall, and specificity are vital for evaluating their effectiveness in various tasks. These metrics help quantify how well an AI model identifies relevant data and minimizes errors.
Additionally, AI significantly enhances dataset annotation by automatically labeling imaging datasets, surgical videos, and other medical data with high accuracy[43]. This capability accelerates the training process for retrospective analyses, thereby increasing research efficiency and identifying areas for improvement in the field.
Looking forward, the development of virtual scribes or “co-pilots” opens exciting possibilities. For patients, AI-powered co-pilots can serve as personalized guides through the care continuum, providing education and answering questions in real time. For surgeons, AI co-pilots can augment the surgical process by providing robust decision support, analyzing intraoperative metrics, and suggesting surgical approaches[44]. We foresee a future where AI co-pilots integrate into the existing architecture of the spine surgery ecosystem [Figure 1]. In addition to offering real-time information to surgeons, co-pilots could assist with elevating critical non-technical roles, including improving communication, aiding with surgical team efficiency, and maintaining situational awareness[45,46].
Figure 1. Data integration in AI-driven spine surgery. This figure illustrates the flow of multiple data collection points into advanced AI systems. The integrated AI processes these diverse inputs to generate valuable clinical outputs that inform patient care. Figure created with BioRender.com. AI: Artificial intelligence.
CHALLENGES
While the implementation of AI in spine surgery holds immense promise, several challenges must be addressed to fully realize its potential. A primary concern is the reliance on high-quality, standardized data. High-quality data are essential for training accurate AI models, and standardization ensures that these models can be applied broadly and effectively across different clinical settings[2,47]. With the paramount importance of privacy and data security, compliance with regulations such as the Health Insurance Portability and Accountability Act (HIPAA) of 1996 is necessary to safeguard patient information.
Furthermore, maintaining trust in AI technology requires transparency and accountability. Skepticism of AI is often centered around the “black box” nature of its operations, where users cannot see how decisions are made. The decision-making process of AI systems should be clear and understandable to clinicians and patients alike. By familiarizing themselves with how these technologies function, they can better grasp the decision-making processes of AI systems. For example, machine learning allows computers to learn from data and improve over time, while deep learning, a subset of machine learning, mimics how the human brain processes information using layers of algorithms to analyze complex data.
A critical issue in the development of AI algorithms is addressing and mitigating biases that could lead to disparities in care. AI models should be trained on diverse and representative datasets to ensure they are applicable to a wide range of clinical and patient populations. To detect and address potential biases, it is essential to implement robust validation techniques, including bias audits and continuous monitoring during deployment. Engaging diverse stakeholders in the design and review processes can further help identify and rectify biases, ensuring fairness and equity in AI-driven treatments.
CONCLUSION
AI’s potential is vast and multifaceted, ranging from enhancing diagnostic accuracy to optimizing postoperative care. Its applications can lead to significant cost reductions, improved therapeutic outcomes, and enhanced quality of patient care. However, realizing this potential requires addressing challenges in data quality, standardization, and ethical implementation. By advancing and actively engaging in the ongoing discourse surrounding AI technologies, we can ensure that AI serves as a transformative force in spine surgery, ushering in a new era of personalized, precise, and proactive spine care.
DECLARATIONS
Authors’ contributions
Conceptualization, design, synthesis, writing, and editing: Fuleihan AA, Menta AK, Azad TD, Theodore N
Writing and editing: Jiang K, Weber-Levine C, Davidar AD, Hersh AM
Availability of data and materials
Not applicable.
Financial support and sponsorship
None.
Conflicts of interest
Theodore N receives royalties from and owns stock in Globus Medical. He is a consultant for Globus Medical and has served on the scientific advisory board/other office for Globus Medical. While the other authors have declared that they have no conflicts of interest.
Ethical approval and consent to participate
Not applicable.
Consent for publication
Not applicable.
Copyright
© The Author(s) 2024.
Supplementary Materials
REFERENCES
1. Varghese C, Harrison EM, O’Grady G, Topol EJ. Artificial intelligence in surgery. Nat Med 2024;30:1257-68.
2. Holzinger A, Langs G, Denk H, Zatloukal K, Müller H. Causability and explainability of artificial intelligence in medicine. Wiley Interdiscip Rev Data Min Knowl Discov 2019;9:e1312.
3. Sahni NR, Stein G, Zemmel R, Cutler D. The potential impact of artificial intelligence on healthcare spending. In: The economics of artificial intelligence. University of Chicago Press; 2023. Available from: https://www.degruyter.com/document/doi/10.7208/chicago/9780226833125-004/pdf?licenseType=restricted. [Last accessed on 10 Oct 2024].
4. Burns JE, Yao J, Summers RM. Vertebral body compression fractures and bone density: automated detection and classification on CT images. Radiology 2017;284:788-97.
5. Al Arif SMMR, Knapp K, Slabaugh G. Fully automatic cervical vertebrae segmentation framework for X-ray images. Comput Methods Programs Biomed 2018;157:95-111.
6. Doerr SA, Weber-Levine C, Hersh AM, et al. Automated prediction of the thoracolumbar injury classification and severity score from CT using a novel deep learning algorithm. Neurosurg Focus 2022;52:E5.
7. Maier-Hein L, Vedula SS, Speidel S, et al. Surgical data science for next-generation interventions. Nat Biomed Eng 2017;1:691-6.
8. Kim JS, Merrill RK, Arvind V, et al. Examining the ability of artificial neural networks machine learning models to accurately predict complications following posterior lumbar spine fusion. Spine 2018;43:853-60.
9. Karhade AV, Bongers MER, Groot OQ, et al. Development of machine learning and natural language processing algorithms for preoperative prediction and automated identification of intraoperative vascular injury in anterior lumbar spine surgery. Spine J 2021;21:1635-42.
10. Wijnberge M, Geerts BF, Hol L, et al. Effect of a machine learning-derived early warning system for intraoperative hypotension vs standard care on depth and duration of intraoperative hypotension during elective noncardiac surgery: the HYPE randomized clinical trial. JAMA 2020;323:1052-60.
11. Kalidasan V, Yang X, Xiong Z, et al. Wirelessly operated bioelectronic sutures for the monitoring of deep surgical wounds. Nat Biomed Eng 2021;5:1217-27.
12. Bihorac A, Ozrazgat-Baslanti T, Ebadi A, et al. MySurgeryRisk: development and validation of a machine-learning risk algorithm for major complications and death after surgery. Ann Surg 2019;269:652-62.
13. Hu Z, Simon GJ, Arsoniadis EG, Wang Y, Kwaan MR, Melton GB. Automated detection of postoperative surgical site infections using supervised methods with electronic health record data. Stud Health Technol Inform 2015;216:706-10.
14. Pellisé F, Serra-Burriel M, Smith JS, et al; International Spine Study Group, European Spine Study Group. Development and validation of risk stratification models for adult spinal deformity surgery. J Neurosurg Spine 2019;31:587-99.
15. El Moheb M, Gebran A, Maurer LR, et al. Artificial intelligence versus surgeon gestalt in predicting risk of emergency general surgery. J Trauma Acute Care Surg 2023;95:565-72.
16. Ames CP, Smith JS, Pellisé F, et al; European Spine Study Group, International Spine Study Group. Artificial intelligence based hierarchical clustering of patient types and intervention categories in adult spinal deformity surgery: towards a new classification scheme that predicts quality and value. Spine 2019;44:915-26.
17. Choy W, Azad TD, Scheer JK, Safaee MM, Ames CP. Biomarkers in adult spinal deformity surgery. Semin Spine Surg 2023;35:101058.
18. Topol EJ. As artificial intelligence goes multimodal, medical applications multiply. Science 2023;381:adk6139.
19. Goedmakers CMW, Lak AM, Duey AH, et al. Deep learning for adjacent segment disease at preoperative MRI for cervical radiculopathy. Radiology 2021;301:664-71.
20. Mirza FN, Tang OY, Connolly ID, et al. Using ChatGPT to facilitate truly informed medical consent. NEJM AI 2024;1:AIcs2300145.
21. Ali R, Connolly ID, Tang OY, et al. Bridging the literacy gap for surgical consents: an AI-human expert collaborative approach. NPJ Digit Med 2024;7:63.
22. Solanki SL, Pandrowala S, Nayak A, Bhandare M, Ambulkar RP, Shrikhande SV. Artificial intelligence in perioperative management of major gastrointestinal surgeries. World J Gastroenterol 2021;27:2758-70.
24. Boczar D, Sisti A, Oliver JD, et al. Artificial intelligent virtual assistant for plastic surgery patient’s frequently asked questions: a pilot study. Ann Plast Surg 2020;84:e16-21.
25. Ni L, Lu C, Liu N, Liu J. MANDY: towards a smart primary care chatbot application. Commun Comput Inform Sci 2017;780:38-52.
26. Ino T, Samukawa M, Ishida T, et al. Validity of AI-based gait analysis for simultaneous measurement of bilateral lower limb kinematics using a single video camera. Sensors 2023;23:9799.
27. Keller AV, Torres-Espin A, Peterson TA, et al. Unsupervised machine learning on motion capture data uncovers movement strategies in low back pain. Front Bioeng Biotechnol 2022;10:868684.
28. Rasouli JJ, Shao J, Neifert S, et al. Artificial intelligence and robotics in spine surgery. Global Spine J 2021;11:556-64.
29. Mascagni P, Alapatt D, Sestini L, et al. Computer vision in surgery: from potential to clinical value. NPJ Digit Med 2022;5:163.
30. Goodman ED, Patel KK, Zhang Y, et al. Analyzing surgical technique in diverse open surgical videos with multitask machine learning. JAMA Surg 2024;159:185-92.
31. Lavanchy JL, Zindel J, Kirtac K, et al. Automation of surgical skill assessment using a three-stage machine learning algorithm. Sci Rep 2021;11:5197.
32. Levin M, McKechnie T, Khalid S, Grantcharov TP, Goldenberg M. Automated methods of technical skill assessment in surgery: a systematic review. J Surg Educ 2019;76:1629-39.
33. Lam K, Chen J, Wang Z, et al. Machine learning for technical skill assessment in surgery: a systematic review. NPJ Digit Med 2022;5:24.
34. Abdelhady AM, Davis CR. Plastic surgery and artificial intelligence: how ChatGPT improved operation note accuracy, time, and education. Mayo Clin Proc 2023;1:299-308.
35. Zaidat B, Tang J, Arvind V, et al. Can a novel natural language processing model and artificial intelligence automatically generate billing codes from spine surgical operative notes? Global Spine J 2024;14:2022-30.
36. Alsareii SA, Raza M, Alamri AM, et al. Machine learning and internet of things enabled monitoring of post-surgery patients: a pilot study. Sensors 2022;22:1420.
37. Lee HJ, Jin SM, Kim SJ, et al. Development and validation of an artificial intelligence-based motion analysis system for upper extremity rehabilitation exercises in patients with spinal cord injury: a randomized controlled trial. Healthcare 2023;12:7.
38. Karhade AV, Ogink PT, Thio QCBS, et al. Development of machine learning algorithms for prediction of prolonged opioid prescription after surgery for lumbar disc herniation. Spine J 2019;19:1764-71.
39. Wells CI, Xu W, Penfold JA, et al. Wearable devices to monitor recovery after abdominal surgery: scoping review. BJS Open 2022;6:zrac031.
40. Dawes AJ, Lin AY, Varghese C, Russell MM, Lin AY. Mobile health technology for remote home monitoring after surgery: a meta-analysis. Br J Surg 2021;108:1304-14.
41. McGirt MJ, Sivaganesan A, Asher AL, Devin CJ. Prediction model for outcome after low-back surgery: individualized likelihood of complication, hospital readmission, return to work, and 12-month improvement in functional disability. Neurosurg Focus 2015;39:E13.
42. Vedula SS, Hager GD. Surgical data science: the new knowledge domain. Innov Surg Sci 2017;2:109-21.
43. Zuluaga L, Rich JM, Gupta R, et al. AI-powered real-time annotations during urologic surgery: the future of training and quality metrics. Urol Oncol 2024;42:57-66.
44. Azad TD, Warman A, Tracz JA, Hughes LP, Judy BF, Witham TF. Augmented reality in spine surgery - past, present, and future. Spine J 2024;24:1-13.
45. Gillespie BM, Harbeck E, Kang E, Steel C, Fairweather N, Chaboyer W. Correlates of non-technical skills in surgery: a prospective study. BMJ Open 2017;7:e014480.
46. Agha RA, Fowler AJ, Sevdalis N. The role of non-technical skills in surgery. Ann Med Surg 2015;4:422-7.
Cite This Article
How to Cite
Fuleihan, A. A.; Menta, A. K.; Azad, T. D.; Jiang, K.; Weber-Levine, C.; Davidar, A. D.; Hersh, A. M.; Theodore, N. Navigating artificial intelligence in spine surgery: implementation and optimization across the care continuum. Art. Int. Surg. 2024, 4, 288-95. http://dx.doi.org/10.20517/ais.2024.39
Download Citation
Export Citation File:
Type of Import
Tips on Downloading Citation
Citation Manager File Format
Type of Import
Direct Import: When the Direct Import option is selected (the default state), a dialogue box will give you the option to Save or Open the downloaded citation data. Choosing Open will either launch your citation manager or give you a choice of applications with which to use the metadata. The Save option saves the file locally for later use.
Indirect Import: When the Indirect Import option is selected, the metadata is displayed and may be copied and pasted as needed.
About This Article
Special Issue
Copyright
Data & Comments
Data
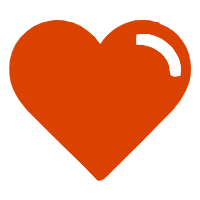
Comments
Comments must be written in English. Spam, offensive content, impersonation, and private information will not be permitted. If any comment is reported and identified as inappropriate content by OAE staff, the comment will be removed without notice. If you have any queries or need any help, please contact us at support@oaepublish.com.