Application and use of artificial intelligence in colorectal cancer surgery: where are we?
Abstract
AI is revolutionizing the landscape of colorectal cancer (CRC) surgery, permeating diverse facets ranging from intraoperative guidance to predictive modeling of postoperative outcomes. This scoping review aims to comprehensively delineate the breadth of artificial intelligence (AI) applications in CRC surgery. A search of PubMed, Embase, and Ebsco databases up to December 2023 was conducted, with registration in the international prospective register of systematic reviews (PROSPERO) (CRD42024502107). Sixty-two studies meeting stringent inclusion criteria were scrutinized, encompassing AI utilization in CRC surgery or the development of AI-driven tools for colorectal surgical practice. Five principal domains of AI application emerged: (i) Intraoperative guidance, leveraging real-time navigation, indocyanine green (ICG) angiography, and hyperspectral imaging (HSI) to enhance surgical precision; (ii) Image segmentation, facilitating phase recognition, tools recognition, and anatomical identification to optimize surgical visualization; (iii) Training and performance assessment, enabling objective evaluation and enhancement of surgical skills through AI-driven simulations and feedback mechanisms; (iv) Prediction of surgical complications, encompassing prognostication of anastomotic leakage (AL) or stricture, stoma requirements, and prediction of low anterior resection syndrome (LARS) and short-term postoperative complications; (v) Utilization of electronic health records (EHRs), harnessing AI algorithms to streamline data analysis and inform decision-making processes. This review underscores the paradigm-shifting impact of AI in CRC surgery, transcending conventional boundaries and catalyzing advancements across diverse surgical domains. Although many applications are still experimental, as AI continues to evolve, it promises to transform surgical practice, optimize outcomes, and revolutionize patient care. Embracing AI technologies is imperative for colorectal surgeons to remain at the vanguard of surgical innovation and deliver superior outcomes for CRC patients.
Keywords
INTRODUCTION
In recent years, the global landscape of artificial intelligence (AI) has witnessed rapid growth[1]. Driven by breakthroughs in sequencing technologies and computational methods, AI has emerged as a powerful tool for improving precision and accuracy in various fields. In the medical field, AI applications have proliferated, revolutionizing diagnostic imaging analysis, pathology interpretation, disease prognosis prediction, complication prevention, and competency assessment[2]. In addition, AI has been used to improve the quality of medical care.
Machine learning (ML), an important subfield of AI, encompasses a variety of data-driven techniques. These algorithms use historical data to gain knowledge and make predictions. Over time, they self-improve, increasing the accuracy of their predictions as they encounter more information[3]. ML can be broadly categorized into three groups. In supervised learning, algorithms trained on labeled data sets map input to output, enabling predictions to be made on unseen data. Common examples include logistic regression (LR), support vector machines (SVMs), and neural networks. In unsupervised learning, algorithms operate on unlabeled data and discover patterns and relationships without explicit guidance. Techniques such as k-means clustering, hierarchical clustering, and principal component analysis (PCA) fall into this category. Finally, in reinforcement learning, agents learn to make decisions by interacting with an environment and receiving rewards or punishments based on their actions. The goal is to optimize the cumulative rewards over time. Notable reinforcement learning algorithms include Q-learning, deep Q-networks (DQN), and policy gradient methods[4].
Deep learning (DL), a subset of ML, focuses on training artificial neural networks (ANNs) without explicit programming. Inspired by the structure of the human brain, DL mimics the neural connections that facilitate experiential learning[5]. In ANNs, interconnected layers of nodes (neurons) form the backbone of DL. Information flows through each layer, enabling hierarchical representations of data. In terms of Depth and Abstraction, DL’s depth enables automatic feature extraction at different levels of abstraction. It mirrors how humans learn from complex data. Finally, in Backpropagation, DL models adjust weights and biases during training based on predicted versus actual results. Accuracy gradually improves, enabling precise predictions.
ML and DL methodologies have been used for some time, but there is a significant lack of confidence and familiarity in healthcare. Among the numerous possible applications, ML and DL techniques can be applied to large clinical datasets for the development of robust models. The learning methods offer tremendous potential to enhance medical research and clinical care. There are many areas that may benefit from the application of ML techniques in the medical field, such as diagnosis, management, and outcome prediction[6,7].
Colorectal cancer (CRC) is the third most commonly diagnosed cancer worldwide and the second leading cause of cancer death[8]. AI-guided care can play a pivotal role in clinical practice to improve strategies for screening, diagnosis, and treatment of patients with CRC[9]. These include increasing the effectiveness of screening, and improving the accuracy of diagnostic tools like colonoscopies by reducing rates of missed adenomas[10]. It also improves the performance of radiologic diagnosis[11]. Recently, there has been an exponential increase in publications regarding the use of AI in the surgical treatment of CRC. The aim of the current study was to review the currently available literature on the applications of AI in the surgical treatment of CRC patients, including all ML and DL methods that offer the colorectal surgeon a tool to improve clinical practice.
METHODS
This study was conducted in accordance with the preferred reporting items for systematic reviews and meta-analysis (PRISMA) statement[12]. The scoping review was conducted using the preferred reporting items for systematic reviews and meta-analyses extension for scoping reviews (PRISMA-ScR) guidelines for reporting[13]. The study protocol was registered on the international prospective register of systematic reviews (PROSPERO, registration number CRD42024502107) on 25 January 2024.
Inclusion/exclusion criteria
Inclusion criteria were established using the Patient, Concept, Context (PCC) criteria following the Joanna Briggs Institute methodology for scoping reviews[14]. All papers that used AI as a tool to analyze data specific to CRC surgery were included. Articles that used AI as a direct application to design the approach or perform surgery were included. The following study designs were considered: randomized controlled trials, controlled clinical trials, observational studies (retrospective and prospective), cohort studies, population-based studies, cross-sectional studies, and case-control studies.
Exclusion criteria were nonsurgical articles, reviews, books and book chapters, conference proceedings, and editorials. Articles related to the diagnostic pathway such as screening, endoscopy, and other nonsurgical areas were excluded. Articles in languages other than English were excluded.
Search strategy, study selection and data collection
A review of the published literature until 15 December 2023 was performed in the following databases: PubMed, Embase, and Ebsco. The keywords (including synonyms or equivalent terms) used included “artificial intelligence”, “machine learning”, “neural network”, “deep learning”, “computer vision”, “natural language” and “colorectal cancer”, “rectal cancer”, “colon cancer”, “CRC” in combination with Boolean operators (AND, OR).
Articles were screened according to the previously described inclusion criteria, and two reviewers independently screened the literature according to the predefined strategy described above. Covidence (Covidence systematic review software, Veritas Health Innovation, Melbourne, Australia) was used to remove duplicates. Two reviewers (F.C. and S.F.) independently screened the titles and abstracts and cross-checked the results of the studies. Disagreements were resolved by a third reviewer (G.S.). Each reviewer extracted the following data variables: title and reference details (first author, journal, year). During the review process, both reviewers independently recorded data in separate databases. To mitigate selection bias, a comparison was conducted at the end. Additionally, manuscripts related to AI applications in colorectal surgery were categorized into five distinct groups based on their primary focus. [i.e., Intraoperative guidance, Image segmentation, Training and performance assessment, Surgical complications prediction, Electronic Health Record (EHR)].
An overview of the study’s methodology is provided in Figure 1.
RESULTS
The search identified 5,787 studies, of which 1,264 were automatically removed from Covidence (Covidence systematic review software, Veritas Health Innovation, Melbourne, Australia) as they were automatically identified as duplicates. Eighty-nine articles were selected for full-text evaluation, and 27 were excluded based on the above exclusion criteria. Finally, 62 studies were included.
The application of AI to the surgical treatment of CRC or the analysis of surgery and its outcomes revealed five primary themes.
Intraoperative guidance
AI-based image recognition has rapidly advanced, nearing human-level capabilities, particularly in minimally invasive surgery (MIS), encompassing both laparoscopic and robotic approaches, to aid surgeons in intraoperative decision making and navigation. In several areas, the use of image-guided surgery has demonstrated significant benefits. For example, in liver surgery during hepatectomy for liver tumors, guidance systems can be used to allow the surgeon to see the tumor and its relationship to major intra-parenchymal vascular structures in real time. Similarly, in spine surgery, real-time image-guided procedures have been shown to provide significant benefits in terms of safety and outcomes.
Seven studies were identified, focusing on real-time navigation, indocyanine green (ICG) angiography, and hyperspectral imaging (HSI) [Table 1] during colorectal surgeries.
Intraoperative guidance
Group | Authors | Year | Model | Objectives |
Real-time navigation | Kitaguchi et al.[15] | 2023 | ANN | Ureter and nerves identification |
Real-time navigation | Kojima et al.[16] | 2023 | CNN | Nerves identification |
Real-time navigation | Ryu et al.[17] | 2023 | DL | Nerves identification |
ICG angiography | Park et al.[18] | 2020 | ANN | Microcirculation assessment |
HSI | Okamoto et al.[19] | 2022 | CNN | Fat tissue discrimination |
HSI | Beaulieu et al.[20] | 2018 | SVM | Pathologic tissue identification |
HSI | Manni et al.[21] | 2020 | CNN | Pathologic tissue identification |
HSI | Jansen-Winkeln et al.[22] | 2021 | ANN | Pathologic tissue identification |
HSI | Collins et al.[23] | 2022 | CNN | Pathologic tissue identification |
HSI | Baltussen et al.[24] | 2019 | SVM | Pathologic tissue identification |
HSI | Tkachenko et al.[25] | 2023 | CNN | Pathologic tissue identification |
Real-time navigation
Several models employing DL algorithms demonstrated high detection rates (ranging from 66% to 100%) for identifying nerve structures during sigmoid and anterior rectal resections[15-17] Kitaguchi et al. and Kojima et al. additionally observed that the models outpaced surgeons in nerve identification in over 50% of cases[15,16].
ICG-angiography
The application of AI in fluorescence laparoscopic systems for intraoperative angiography using ICG enables the prediction of hypoperfusion-related complications, such as anastomotic leakage (AL)[18]. Park
HSI
HSI offers contrast-free optical imaging, enabling quantitative assessment of physiological tissue parameters and visualization of anatomical structures. In colorectal surgery, HSI provides valuable insights into tissue composition, oxygenation levels, and metabolic activity, aiding in precise interventions and complication mitigation.
The included papers utilize operative and specimen images taken outside of the surgical piece, and thus, the application of these models is usable intraoperatively.
Okamoto et al. proposed a convolutional neural network (CNN)-based model to differentiate colic and mesocolic tissues from retroperitoneal tissues in pigs, achieving high sensitivity (86.0% ± 16.0%) and F1 score (0.90 ± 0.11) for colon-mesocolon discrimination[19]. Moreover, several studies utilized ML algorithms for HSI image analysis, achieving > 90% sensitivity and > 80% specificity in discriminating pathological from healthy tissue in colon specimens[20,21], showcasing the potential for real-time hyperspectral technology integration during colorectal surgery. The use of the HSI can also be aimed at evaluating the resected surgical specimen after extraction, in order to check whether the resection margin can be adequate, as has been effectively demonstrated in several publications. Jansen-Winkeln et al. developed a multi-layer perceptron (MLP) model to differentiate CRC from healthy mucosa and adenomas with an accuracy of 94%[22]. Several models have been developed with the same objective, all with excellent results in discriminating between healthy and malignant colon tissue[23-25].
Image segmentation
Semantic segmentation, a groundbreaking image recognition methodology grounded in pixel-level classification, stands as a transformative force in the realm of surgical applications. Its implications span the entire spectrum of clinical processes, including diagnosis, intervention planning, and computer-assisted surgery. However, despite its immense potential, the application of semantic segmentation for organ delineation using intraoperative video presents unique challenges, primarily stemming from the demands of manual annotation and the intricacies of deploying sophisticated automatic segmentation algorithms.
In the surgical field, semantic segmentation becomes a guiding light for surgeons. By facilitating the real-time identification and delineation of organs and structures, it assists in preoperative planning and intraoperative decision making. This not only enhances surgical precision but also contributes to the overall safety and efficacy of procedures.
Several studies have been identified that have used image segmentation with different objectives: segmentation of entire surgeries with the aim of classifying different stages, or segmentation of specific steps with the aim of creating an algorithm for recognizing anatomical structures (e.g., blood vessels) and dissection planes [Table 2].
Image segmentation
Group | Authors | Year | Model | Objectives |
Phases recognition | Jalal et al.[26] | 2018 | CNN; HMM | Sigmoid resection segmentation |
Phases recognition | Kitaguchi et al.[27] | 2020 | CNN | Sigmoid resection segmentation |
Phases recognition | Kitaguchi et al.[28] | 2022 | CNN | TaTME segmentation |
Phases recognition | Kitaguchi et al.[29] | 2021 | CNN | TaTME segmentation |
Phases recognition | Kolbinger et al.[30] | 2023 | CNN | Robotic rectal resection segmentation |
Phases and tools recognition | Kitaguchi et al.[31] | 2020 | CNN | Colorectal resection segmentation |
Tools recognition | Kitaguchi et al.[32] | 2022 | CNN | Instrument identification |
Tools recognition | Maier-Hein et al.[33] | 2021 | CNN | Instrument identification |
Anatomy identification | Ryu et al.[34] | 2023 | DL | Vessels recognition |
Anatomy identification | Igaki et al.[35] | 2022 | DL | Mesorectal plain identification |
Anatomy identification | Kitaguchi et al.[36] | 2022 | CNN | Vessels recognition |
Phases recognition
Automated recognition of surgical steps through image segmentation serves multiple purposes, aiding in accurately identifying and localizing different anatomical structures within the surgical field, enhancing understanding of the surgical site, and improving decision making throughout various stages of the intervention. Several algorithms have been developed for automatic stage detection in colorectal surgery. Jalal et al. and Kitaguchi et al. developed CNN-based DL models for automatic recognition of laparoscopic sigmoid resection phases, achieving overall accuracy rates of 91.9% and 89.4% for extracorporeal action and irrigation recognition, respectively[26,27]. Moreover, Kitaguchi et al. devised CNN-based models for video segmentation of transanal total mesorectal excision (TaTME), achieving overall accuracies of 93.2% for main surgical steps and 76.7% for sub-steps[28,29]. Kolbinger et al. trained ML models to recognize phases of robotic rectal resection, with their best-performing model achieving a dice similarity coefficient of 0.82 and an accuracy of 0.84[30].
Tools recognition
Automatic recognition of surgical instruments offers several benefits, including standardization of surgical technique, facilitating instrument management in operating rooms, and enabling automatic storage. Kitaguchi et al. developed CNN-based models for automatically recognizing surgical instruments commonly used in laparoscopic colorectal surgery, achieving mean average precision rates exceeding 90%[31,32]. In 2021, Maier-Hein et al. published the Heidelberg Colorectal (HeiCo) dataset based on images from 30 videos of colorectal surgery for laparoscopic instrument recognition[33].
Anatomy identification
Ryu et al. constructed a DL model to automatically recognize major blood vessels during right hemicolectomy with central vascular ligation, achieving DSC scores of 0.78 for the superior mesenteric vein, 0.55 for the ileocolic artery, and 0.54 for the ileocolic vein[34]. Igaki et al. developed a DL model to identify the correct plane for mesorectal dissection during laparoscopic total mesorectal excision (TME), achieving a DSC of 0.84[35]. Additionally, Kitaguchi et al. developed a DL model to identify the inferior mesenteric artery during sigmoid resection, achieving a mean DSC of 0.798 and demonstrating potential for real-time surgical use[36].
Training and performance assessment
The segmentation of surgical videos can also be used for educational purposes and evaluation purposes. After the surgery, segmented images can be used for postoperative assessment, allowing surgeons to review the outcomes and identify any issues that may require further attention or intervention. The automatic indexing of documented procedures can be useful for educational purposes, analysis of complications, consultations, patient briefs, and treatment planning.
Image segmentation aids in the creation of realistic 3D models and simulations for training purposes. Surgeons and surgical trainees can practice and enhance their skills in a simulated environment, improving their understanding of the different stages of surgical procedures [Table 3].
Training and performance
Group | Authors | Year | Model | Objectives |
Training and performance | Ryu et al.[37] | 2023 | DL | Real-time training for TME |
Training and performance | Kitaguchi et al.[39] | 2023 | CNN | Purse-string suture in TaTME |
Training and performance | Igaki et al.[40] | 2023 | CNN | Assess surgical skill |
Training and performance | Kitaguchi et al.[41] | 2021 | CNN | Assess surgical skill |
Training and performance | Kolbinger et al.[42] | 2023 | CNN | Assess surgical skill |
Training and performance | Sasaki et al.[43] | 2023 | LR | Tissue handling evaluation |
Ryu et al. successfully applied the DL-Eureka model developed by Kumazu et al. for real-time surgical training, demonstrating the safe performance of TME with nerve identification and preservation[37,38]. Kitaguchi et al. developed an automatic skill assessment system for purse-string suture in TaTME using a DL-based approach[39]. Moreover, Igaki et al., Kitaguchi et al., and Kolbinger et al. devised DL models based on intraoperative videos of laparoscopic colorectal surgery for automatic surgical skill assessment[40-42]. Igaki’s model[40] exhibited favorable performance compared to the endoscopic surgical skill qualification system (ESSQS), achieving sensitivity and specificity exceeding 80% and 90%, respectively. Sasaki et al. developed a model for laparoscopic tissue handling evaluation using intraoperative videos of laparoscopic colorectal surgery[43]. They trained a ML model to classify pixel RGB values into blood and non-blood, achieving an overall accuracy of 85.7%.
Surgical complications prediction
A total of 34 articles employing AI to predict surgical complications following colorectal surgery were identified.
Anastomotic complications
AL stands as one of the most common and serious complications following CRC surgery, with reported incidences ranging from 3% to 21%[44]. AL significantly impacts patient outcomes, often necessitating prolonged hospital stays, re-operation, and increased mortality rates. Despite efforts to identify risk factors for AL[45], predicting its occurrence remains challenging. Various models, including random forest classifiers[46], regression models[47,48], and ANN-based models[49], have been developed to predict AL risk factors and occurrence. Risk factors identified include tumor distance from the anal verge, T4 stage tumors, male sex, preoperative stenosis, preoperative anemia, massive blood loss, diabetes, hypertension, neoadjuvant radiotherapy, and surgeon volume. Models developed by Adams et al., Shao et al., Baker et al., and Sammour et al. demonstrated efficacy in predicting AL occurrence[50-53].
Non-malignant anastomotic stenosis following rectal cancer surgery presents a significant concern, with incidences ranging from 2% to 19%[54,55]. Su et al. developed ML models, with random forest exhibiting superior discriminatory and predictive efficacy for predicting anastomotic stenosis[56]. Prophylactic ileostomy, operative time, and AL were found to significantly influence the model [Table 4].
Anastomotic complication prediction
Complication prediction | Authors | Year | Model | Risk factors |
AL | Wen et al.[46] | 2021 | RF | Distance from AV, male sex, preoperative stenosis, anemia, blood loss, diabetes, Neoadj CRT, surgeon volume |
AL | Shen et al.[47] | 2023 | LR | Hypertension, cT4 stage, intraoperative blood loss > 100 mL, operating time > 160 min, and tumor location |
AL | Arezzo et al.[48] | 2019 | LR | Male sex, short-course neoadjuvant RT, T4 tumor, blood transfusion, and tumor distance |
AL | Mazaki et al.[49] | 2021 | ANN | pT4, double-row circular stapler |
AL | Adams et al.[50] | 2014 | ANN | Main predictors were CRP on POD 4-5, PLT count on POD 1-5, preoperative hemoglobin |
AL | Shao et al.[51] | 2022 | RF | Transverse diameter of abdominal cavity (TD), anterior to posterior diameter of abdominal cavity (APD) and visceral fat area (VFA) |
AL | Baker et al.[52] | 2022 | ML | Clostridium difficile infection |
AL | Sammour et al.[53] | 2017 | DT | AL risk calculators evaluation |
Non-malignant anastomotic stenosis | Su et al.[56] | 2023 | RF | Prophylactic ileostomy, operative time, AL |
Ostomy prediction after rectal surgery
Temporary or permanent ostomy creation following TME for rectal cancer remains a vital consideration to prevent AL. Shao et al. employed SVM models to predict the need for a temporary ileostomy, while Liu
Ostomy prediction after rectal surgery
Stoma prediction | Authors | Year | Model | Risk factors |
Temporary ileostomy | Shao et al.[57] | 2023 | SVM | Operative time, location of the tumor, preoperative albumin levels, the incidence of diabetes and the electrolyte disorders |
Definitive ostomy | Liu et al.[58] | 2023 | XGBoost | Tumor distance from dentate line, advanced age, previous chemoradiotherapy, rectal stricture, diabetes, hypertension |
Definitive ostomy | Kuo et al.[59] | 2023 | DT, LightGBM | Distance of the lesion from the anal verge, clinical N stage, age, sex, ASA score, and preoperative albumin and CEA levels |
Low anterior resection syndrome
Up to 40% of patients may experience low anterior resection syndrome (LARS) following rectal surgery, adversely impacting quality of life[60]. LR models[61,62] and random forest models[63] have been utilized to predict LARS risk factors, including length of distal rectum, AL, neoadjuvant therapy, presence of diverting stoma, and type of surgery [Table 6].
LARS prediction
Complication prediction | Authors | Year | Model | Risk factors |
LARS risk | Qin et al.[61] | 2023 | RF, LR, SVM, DT | Length of distal rectum, AL, proximal colon of neorectum (sigmoid/descending), and pathologic nodal stage |
LARS risk | Huang et al.[62] | 2023 | LR, SVM, DT, RF, ANN | Distance from the anal verge, presence of diverting stoma, exsufflation, and type of surgery |
LARS risk | Wang et al.[63] | 2023 | RF | Anastomotic height, neoadjuvant therapy, presence of stoma, BMI |
Early complications after colorectal surgery
Studies investigating AI models for predicting early complications after colorectal surgery encompass a range of algorithms, including LR, neural networks, and random forest classifiers[64-70]. These models, developed by various researchers, utilize clinical risk factors to comprehensively predict postoperative complication rates. Identified risk factors include surgical site infection[71,72], length of stay, readmissions, mortality[73], textbook outcome[74], and conversion from MIS to open surgery[75]. These findings underscore the potential of AI models in predicting surgical complications following colorectal surgery, aiding clinicians in risk assessment and patient management [Table 7].
Early complications prediction
Complication prediction | Authors | Year | Model | Risk factors |
Early complications | Wang et al.[64] | 2023 | RF | Inflammation-related prognostic index, prognostic nutrition index, tumor location, T stage |
Early complications | Lin et al.[65] | 2022 | LR | ASA ≥ 3, ECOG-PS ≥ 2, open surgery, emergency surgery and tumor perforation |
Early complications | Wei et al.[66] | 2023 | XGBoost | Distance from the anus, age at diagnosis, surgery time, comorbidities |
Early complications | Merath et al.[67] | 2020 | DT | Best performance in prediction of stroke, wound dehiscence, cardiac arrest, progressive renal failure |
Early complications | Francis et al.[68] | 2015 | ANN | Non-mobilization on postoperative day 1, development of ileus, and continuation of IV fluids beyond postoperative day 1 |
Early complications | Manilich et al.[69] | 2013 | RF | Readmission rates, rates of transfusions, SSI: BMI, operative time, identity of the surgeon |
Myocardial infarction risk | Liu et al.[70] | 2023 | XGBoost | Advanced age, preoperative and intraoperative tachycardia, BMI ≥ 25 kg/m2, history of smoking, NLR ≥ 3, CRP ≥ 10 mg/L, intraoperative blood transfusion, intraoperative SPO2 < 90%, operative time ≥ 270 min, and intraoperative bleeding ≥ 100 mL |
SSI | Chen et al.[71] | 2023 | ANN | SSI present at the time of surgery, operative time, oral antibiotic bowel preparation, and surgical approach |
SSI | Ohno et al.[72] | 2022 | ANN | Length of hospital stay, blood loss, lymphocyte to monocyte ratio, and insulin use |
LOS, readmission, mortality | Masum et al.[73] | 2022 | SVM, Bi-LSTM | LOS: age, ASA, operative time. Readmission: age, laparoscopic procedure, stoma performed. Mortality: age, ASA, BMI |
Textbook outcome | Ashraf Ganjouei et al.[74] | 2024 | XGBoost | Surgical approach, patient age, preoperative hematocrit, preoperative oral antibiotic bowel preparation |
Conversion risk | Guidolin et al.[75] | 2023 | LR, RF | Age, BMI, sex, diabetes, ASA class, wound class, ascites, T stage, weight loss, pneumonia |
EHR
AI has revolutionized the interpretation and utilization of vast amounts of data, particularly within EHRs. EHRs serve as a digital repository of a patient’s medical history, encompassing clinical analyses, imaging results, surgical records, laboratory tests, and pathology reports. They aim to enhance patient care quality and safety by providing a comprehensive, accessible, and accurate record for healthcare professionals. EHRs facilitate improved communication, coordination among healthcare providers, informed clinical decision making, and streamlined healthcare processes. Moreover, they offer extensive opportunities for research and clinical practice improvement through automated processes. The automation of EHR data analysis is crucial in clinical settings to distill complex data into actionable insights, thus enabling new avenues for research and development. Several AI models have been developed to analyze EHRs in order to identify and predict the occurrence of complications in patients undergoing colorectal surgery [Table 8]: Ruan et al. utilized a Gated Recurrent Unit with Decay based DL architectures and atemporal LR models to predict wound and organ space infections, superficial infections, and bleeding post-surgery[76]. Weller et al. achieved an AUROC of 0.86 for bleeding complications on POD2 using various ML models, including random forest[77]. Chen et al. designed a GBM model to predict postoperative bleeding risk, identifying risk factors such as anemia, hemophilia, surgery length, heart failure, and kidney disease[78]. Soguero-Ruiz et al. developed a Bag-of-Words and SVM-based feature selection model capable of detecting AL occurrence[79]. Jo et al. identified an eXtreme Gradient Boosting; (XGBoost) model to predict prolonged length of stay after surgery using EHR data, with main risk factors including surgeon, cooperation, albumin levels, specific surgeries, urinary symptoms, marital status, N stage, and urine white blood cell count[80]. Furthermore, Strömblad et al. developed a ML model to predict surgery duration, leading to improved accuracy in predicting case duration and reduced patient waiting time for colorectal surgery[81]. These advancements highlight the transformative potential of AI-driven EHR analysis in enhancing patient care outcomes and optimizing healthcare delivery in CRC surgery.
EHR
Group | Authors | Year | Model | Objectives |
EHR | Ruan et al.[76] | 2022 | RNN | Early diagnosis of complications |
EHR | Weller et al.[77] | 2018 | RF | Early diagnosis of complications |
EHR | Chen et al.[78] | 2018 | GBM | Early diagnosis of bleeding |
EHR | Soguero-Ruiz et al.[79] | 2016 | SVM | AL detection in EHR |
EHR | Jo et al.[80] | 2021 | XGBoost | LOS |
EHR | Strömblad et al.[81] | 2021 | ML | optimizing operating room time |
DISCUSSION
The application of AI in colorectal surgery represents a burgeoning field with significant potential to augment various aspects of surgical practice. This comprehensive review highlights five major areas where AI is making substantial contributions, including intraoperative guidance, image segmentation, training and performance assessment, prediction of surgical complications, and HER analysis. AI-driven image recognition technologies are advancing rapidly, offering surgeons real-time navigation aids during colorectal procedures[81].
These innovations, such as ICG angiography and HSI, provide invaluable insights into tissue perfusion and anatomical structures, aiding in surgical decision making and enhancing patient safety[22,23].
Moreover, DL algorithms have demonstrated remarkable proficiency in identifying nerve structures and anatomical landmarks, surpassing human capabilities in certain instances[19,20].
Despite these advancements, challenges remain in integrating these technologies seamlessly into the surgical workflow and ensuring their reliability in diverse clinical scenarios. Semantic segmentation techniques, facilitated by CNNs, offer precise organ delineation and surgical phase recognition, thereby improving preoperative planning and intraoperative navigation[27,28]. However, the complexity of deploying automatic segmentation algorithms in real-world surgical settings poses significant challenges, including the need for extensive training data and robust validation protocols[30]. Additionally, ensuring the accuracy and reliability of these algorithms across different patient populations and surgical variations requires further investigation.
AI-driven models for surgical skill assessment and performance evaluation offer promising avenues for enhancing surgical training and proficiency[41]. By analyzing surgical videos and extracting relevant metrics, these models provide objective feedback to surgeons and trainees, facilitating targeted skill development and continuous improvement[40,43]. Nevertheless, the generalizability of these models to diverse surgical contexts and the incorporation of subjective elements, such as intraoperative decision making, present notable challenges[37]. Moreover, the reliance on retrospective data and the lack of standardized evaluation criteria limits the applicability of these models in real-time surgical settings.
Predictive models leveraging AI algorithms enable early identification of surgical complications, thereby informing preoperative planning and optimizing patient outcomes[57]. However, the effectiveness of these models hinges on the availability of comprehensive and high-quality data, as well as the accurate identification of relevant risk factors[44]. Moreover, the interpretability of these models and the integration of probabilistic predictions into clinical decision making frameworks require careful consideration and validation in prospective clinical studies.
AI-driven analysis of EHR data holds great potential for enhancing clinical decision making, improving patient outcomes, and advancing research endeavors[76-81]. By automating data analysis and extracting actionable insights from large-scale EHR repositories, AI algorithms enable clinicians to identify patterns, predict outcomes, and personalize treatment strategies[77,79]. Nonetheless, challenges related to data privacy, interoperability, and algorithmic bias necessitate robust governance frameworks and interdisciplinary collaboration to mitigate risks and maximize the benefits of AI in healthcare.
The application of AI models in colorectal surgery has a number of advantages but also current limitations. On the one hand, AI enhances intraoperative guidance, significantly improving decision making and navigation during minimally invasive procedures. Techniques such as real-time navigation and ICG angiography have demonstrated high detection rates of critical anatomical structures, which can lead to safer procedures and better outcomes. HSI further enhances the surgical experience by providing detailed information about tissue characteristics. In addition, AI supports semantic segmentation, enabling accurate identification of anatomical structures and surgical steps, which can improve surgical training and performance evaluation[82].
In the landscape of AI applications in colorectal surgery, several limitations warrant acknowledgment.
Firstly, the inclusion criteria may have introduced selection bias, potentially overlooking relevant studies or emerging technologies. Additionally, the reliance on published literature may have overlooked unpublished studies or ongoing research initiatives, leading to incomplete coverage of the field. Furthermore, the heterogeneity of study designs, patient populations, and outcome measures across the included studies may limit the generalizability of the findings. Finally, the dynamic nature of AI technologies and the rapid evolution of surgical practices necessitate continuous updates and revisions to reflect the latest advancements and insights in the field.
Despite the encouraging outcomes reported in preliminary investigations, significant knowledge gaps remain that need to be addressed. Challenges persist in the deployment of AI models, necessitating rigorous validation and oversight to ensure accuracy, as inaccuracies in image recognition can result in severe complications. Moreover, the incorporation of AI into clinical workflows may require additional training for surgical teams, which could complicate established practices. Ethical concerns surrounding data privacy and the implications of using AI in patient care further complicate matters. Lastly, while AI has the potential to enhance efficiency, its implementation demands considerable investment in technology and infrastructure, which may not be attainable for all healthcare environments. Balancing these advantages and challenges is essential for the successful integration of AI into colorectal surgery. Many preliminary studies have been conducted in the realm of AI and CRC; however, the studies included in this review exhibit significant variability in sample size and presentation methods. This variability impedes meaningful comparisons across studies, particularly in models designed to predict postoperative complications. Consequently, this review is limited to a descriptive summary of the studied methods without a comprehensive evaluation of their actual clinical applicability in practice.
Although, as mentioned above, these models are largely experimental and their clinical applicability is currently undefined, they certainly open the door to the future of surgery, not only in the colorectal field, but with a broader thought, in all branches of surgery. The integration of AI models into minimally invasive and, in particular, robotic surgery will make it possible to perform surgeries with increasing safety, precision and better results. The application of complication prediction models will improve patient selection and treatment management, reduce the occurrence of adverse events, and optimize resources, including economic ones. The training of trainee surgeons is already supported by 3D simulators and AI-modeled video material; in the future, augmented reality will enable further advancements in this training. In the more distant future, automatic real-time recognition of anatomical structures, instruments, and surgical steps will lay the groundwork for a future of autonomous actions in surgery.
However, we remain focused on how AI will be implemented and applied in all clinical settings, with the hope that it will enable an overall improvement in surgical practice in the field of CRC.
CONCLUSION
While AI holds immense promise in transforming colorectal surgery, addressing the aforementioned limitations and navigating the complex interplay between technological innovation, clinical practice, and patient care are essential for realizing its full potential. Collaborative efforts between clinicians, researchers, policymakers, and industry stakeholders are paramount to harnessing the benefits of AI while safeguarding patient safety and advancing the quality of surgical care.
DECLARATIONS
Authors’ contributions
Conception and design of the study: Celotto F, Ferrari S
Acquisition, analysis, and interpretation of data: Celotto F, Ferrari S, Capelli G, Scarpa M
Manuscript draft preparation: Celotto F, Capelli G, Scarpa M, Pucciarelli S, Spolverato G
Manuscript review and editing: Celotto F, Ferrari S, Capelli G, Scarpa M, Pucciarelli S, Spolverato G
Availability of data and materials
Not applicable.
Financial support and sponsorship
None.
Conflicts of interest
Spolverato G is the Associate Editor of the journal Artificial Intelligence Surgery, while the other authors have declared that they have no conflicts of interest.
Ethical approval and consent to participate
Not applicable.
Consent for publication
Not applicable.
Copyright
© The Author(s) 2024.
REFERENCES
1. Zhao M, Tang Y, Kim H, Hasegawa K. Machine learning with K-means dimensional reduction for predicting survival outcomes in patients with breast cancer. Cancer Inform 2018;17:1176935118810215.
2. Elemento O, Leslie C, Lundin J, Tourassi G. Artificial intelligence in cancer research, diagnosis and therapy. Nat Rev Cancer 2021;21:747-52.
3. Jordan MI, Mitchell TM. Machine learning: trends, perspectives, and prospects. Science 2015;349:255-60.
4. Xu Y, Liu X, Cao X, et al. Artificial intelligence: a powerful paradigm for scientific research. Innovation 2021;2:100179.
5. Egger J, Gsaxner C, Pepe A, et al. Medical deep learning - a systematic meta-review. Comput Methods Programs Biomed 2022;221:106874.
6. Handelman GS, Kok HK, Chandra RV, Razavi AH, Lee MJ, Asadi H. eDoctor: machine learning and the future of medicine. J Intern Med 2018;284:603-19.
7. Maier-Hein L, Eisenmann M, Sarikaya D, et al. Surgical data science - from concepts toward clinical translation. Med Image Anal 2022;76:102306.
8. Sung H, Ferlay J, Siegel RL, et al. Global cancer statistics 2020: GLOBOCAN estimates of incidence and mortality worldwide for 36 cancers in 185 countries. CA Cancer J Clin 2021;71:209-49.
9. Maier-Hein L, Vedula SS, Speidel S, et al. Surgical data science for next-generation interventions. Nat Biomed Eng 2017;1:691-6.
11. Inchingolo R, Maino C, Cannella R, et al. Radiomics in colorectal cancer patients. World J Gastroenterol 2023;29:2888-904.
12. Liberati A, Altman DG, Tetzlaff J, et al. The PRISMA statement for reporting systematic reviews and meta-analyses of studies that evaluate health care interventions: explanation and elaboration. J Clin Epidemiol 2009;62:e1-34.
13. Tricco AC, Lillie E, Zarin W, et al. PRISMA extension for scoping reviews (PRISMA-ScR): checklist and explanation. Ann Intern Med 2018;169:467-73.
14. Pollock D, Peters MDJ, Khalil H, et al. Recommendations for the extraction, analysis, and presentation of results in scoping reviews. JBI Evid Synth 2023;21:520-32.
15. Kitaguchi D, Harai Y, Kosugi N, et al. Artificial intelligence for the recognition of key anatomical structures in laparoscopic colorectal surgery. Br J Surg 2023;110:1355-8.
16. Kojima S, Kitaguchi D, Igaki T, et al. Deep-learning-based semantic segmentation of autonomic nerves from laparoscopic images of colorectal surgery: an experimental pilot study. Int J Surg 2023;109:813-20.
17. Ryu S, Goto K, Kitagawa T, et al. Real-time artificial intelligence navigation-assisted anatomical recognition in laparoscopic colorectal surgery. J Gastrointest Surg 2023;27:3080-2.
18. Park SH, Park HM, Baek KR, Ahn HM, Lee IY, Son GM. Artificial intelligence based real-time microcirculation analysis system for laparoscopic colorectal surgery. World J Gastroenterol 2020;26:6945-62.
19. Okamoto N, Rodríguez-Luna MR, Bencteux V, et al. Computer-assisted differentiation between colon-mesocolon and retroperitoneum using hyperspectral imaging (HSI) technology. Diagnostics 2022;12:2225.
20. Beaulieu RJ, Goldstein SD, Singh J, Safar B, Banerjee A, Ahuja N. Automated diagnosis of colon cancer using hyperspectral sensing. Int J Med Robot 2018;14:e1897.
21. Manni F, Fonolla R, der Sommen FV, et al. Hyperspectral imaging for colon cancer classification in surgical specimens: towards optical biopsy during image-guided surgery. Annu Int Conf IEEE Eng Med Biol Soc 2020;2020:1169-73.
22. Jansen-Winkeln B, Barberio M, Chalopin C, et al. Feedforward artificial neural network-based colorectal cancer detection using hyperspectral imaging: a step towards automatic optical biopsy. Cancers 2021;13:967.
23. Collins T, Bencteux V, Benedicenti S, et al. Automatic optical biopsy for colorectal cancer using hyperspectral imaging and artificial neural networks. Surg Endosc 2022;36:8549-59.
24. Baltussen EJM, Kok END, Brouwer de Koning SG, et al. Hyperspectral imaging for tissue classification, a way toward smart laparoscopic colorectal surgery. J Biomed Opt 2019;24:1-9.
25. Tkachenko M, Chalopin C, Jansen-Winkeln B, Neumuth T, Gockel I, Maktabi M. Impact of pre- and post-processing steps for supervised classification of colorectal cancer in hyperspectral images. Cancers 2023;15:2157.
26. Jalal NA, Abdulnaki Alshirbaji T, Möller K. Evaluating convolutional neural network and hidden markov model for recognising surgical phases in sigmoid resection. Curr Dir Biomed Eng 2018;4:415-8.
27. Kitaguchi D, Takeshita N, Matsuzaki H, et al. Real-time automatic surgical phase recognition in laparoscopic sigmoidectomy using the convolutional neural network-based deep learning approach. Surg Endosc 2020;34:4924-31.
28. Kitaguchi D, Takeshita N, Matsuzaki H, et al. Deep learning-based automatic surgical step recognition in intraoperative videos for transanal total mesorectal excision. Surg Endosc 2022;36:1143-51.
29. Kitaguchi D, Takeshita N, Matsuzaki H, et al. Computer-assisted real-time automatic prostate segmentation during TaTME: a single-center feasibility study. Surg Endosc 2021;35:2493-9.
30. Kolbinger FR, Bodenstedt S, Carstens M, et al. Artificial intelligence for context-aware surgical guidance in complex robot-assisted oncological procedures: an exploratory feasibility study. Eur J Surg Oncol 2023:106996.
31. Kitaguchi D, Takeshita N, Matsuzaki H, et al. Automated laparoscopic colorectal surgery workflow recognition using artificial intelligence: experimental research. Int J Surg 2020;79:88-94.
32. Kitaguchi D, Lee Y, Hayashi K, et al. Development and validation of a model for laparoscopic colorectal surgical instrument recognition using convolutional neural network-based instance segmentation and videos of laparoscopic procedures. JAMA Netw Open 2022;5:e2226265.
33. Maier-Hein L, Wagner M, Ross T, et al. Heidelberg colorectal data set for surgical data science in the sensor operating room. Sci Data 2021;8:101.
34. Ryu K, Kitaguchi D, Nakajima K, et al. Deep learning-based vessel automatic recognition for laparoscopic right hemicolectomy. Surg Endosc 2024;38:171-8.
35. Igaki T, Kitaguchi D, Kojima S, et al. Artificial intelligence-based total mesorectal excision plane navigation in laparoscopic colorectal surgery. Dis Colon Rectum 2022;65:e329-33.
36. Kitaguchi D, Takeshita N, Matsuzaki H, et al. Real-time vascular anatomical image navigation for laparoscopic surgery: experimental study. Surg Endosc 2022;36:6105-12.
37. Ryu S, Goto K, Imaizumi Y, Nakabayashi Y. Laparoscopic colorectal surgery with anatomical recognition with artificial intelligence assistance for nerves and dissection layers. Ann Surg Oncol 2024;31:1690-1.
38. Kumazu Y, Kobayashi N, Kitamura N, et al. Automated segmentation by deep learning of loose connective tissue fibers to define safe dissection planes in robot-assisted gastrectomy. Sci Rep 2021;11:21198.
39. Kitaguchi D, Teramura K, Matsuzaki H, Hasegawa H, Takeshita N, Ito M. Automatic purse-string suture skill assessment in transanal total mesorectal excision using deep learning-based video analysis. BJS Open 2023;7:zrac176.
40. Igaki T, Kitaguchi D, Matsuzaki H, et al. Automatic surgical skill assessment system based on concordance of standardized surgical field development using artificial intelligence. JAMA Surg 2023;158:e231131.
41. Kitaguchi D, Takeshita N, Matsuzaki H, Igaki T, Hasegawa H, Ito M. Development and validation of a 3-dimensional convolutional neural network for automatic surgical skill assessment based on spatiotemporal video analysis. JAMA Netw Open 2021;4:e2120786.
42. Kolbinger FR, Rinner FM, Jenke AC, et al. Anatomy segmentation in laparoscopic surgery: comparison of machine learning and human expertise - an experimental study. Int J Surg 2023;109:2962-74.
43. Sasaki S, Kitaguchi D, Takenaka S, et al. Machine learning-based automatic evaluation of tissue handling skills in laparoscopic colorectal surgery: a retrospective experimental study. Ann Surg 2023;278:e250-5.
44. Chiarello MM, Fransvea P, Cariati M, Adams NJ, Bianchi V, Brisinda G. Anastomotic leakage in colorectal cancer surgery. Surg Oncol 2022;40:101708.
45. Rencuzogullari A, Benlice C, Valente M, Abbas MA, Remzi FH, Gorgun E. Predictors of anastomotic leak in elderly patients after colectomy: nomogram-based assessment from the american college of surgeons national surgical quality program procedure-targeted cohort. Dis Colon Rectum 2017;60:527-36.
46. Wen R, Zheng K, Zhang Q, et al. Machine learning-based random forest predicts anastomotic leakage after anterior resection for rectal cancer. J Gastrointest Oncol 2021;12:921-32.
47. Shen Y, Huang LB, Lu A, Yang T, Chen HN, Wang Z. Prediction of symptomatic anastomotic leak after rectal cancer surgery: a machine learning approach. J Surg Oncol 2024;129:264-72.
48. Arezzo A, Migliore M, Chiaro P, et al; REAL Score Collaborators. The REAL (REctal Anastomotic Leak) score for prediction of anastomotic leak after rectal cancer surgery. Tech Coloproctol 2019;23:649-63.
49. Mazaki J, Katsumata K, Ohno Y, et al. A novel predictive model for anastomotic leakage in colorectal cancer using auto-artificial intelligence. Anticancer Res 2021;41:5821-5.
50. Adams K, Papagrigoriadis S. Creation of an effective colorectal anastomotic leak early detection tool using an artificial neural network. Int J Colorectal Dis 2014;29:437-43.
51. Shao SL, Li YK, Qin JC, Liu L. Comprehensive abdominal composition evaluation of rectal cancer patients with anastomotic leakage compared with body mass index-matched controls. World J Gastrointest Surg 2022;14:1250-9.
52. Baker SE, Monlezun DJ, Ambroze WL Jr, Margolin DA. Anastomotic leak is increased with Clostridium difficile infection after colectomy: machine learning-augmented propensity score modified analysis of 46 735 patients. Am Surg 2022;88:74-82.
53. Sammour T, Cohen L, Karunatillake AI, et al. Validation of an online risk calculator for the prediction of anastomotic leak after colon cancer surgery and preliminary exploration of artificial intelligence-based analytics. Tech Coloproctol 2017;21:869-77.
54. Lee SY, Kim CH, Kim YJ, Kim HR. Anastomotic stricture after ultralow anterior resection or intersphincteric resection for very low-lying rectal cancer. Surg Endosc 2018;32:660-6.
55. Sartori A, De Luca M, Fiscon V, Frego M, Portale G; CANSAS study working group. Retrospective multicenter study of post-operative stenosis after stapled colorectal anastomosis.
56. Su Y, Li Y, Chen W, Yang W, Qin J, Liu L. Automated machine learning-based model for predicting benign anastomotic strictures in patients with rectal cancer who have received anterior resection. Eur J Surg Oncol 2023;49:107113.
57. Shao S, Zhao Y, Lu Q, Liu L, Mu L, Qin J. Artificial intelligence assists surgeons’ decision-making of temporary ileostomy in patients with rectal cancer who have received anterior resection. Eur J Surg Oncol 2023;49:433-9.
58. Liu Y, Zhao S, Du W, et al. Applying interpretable machine learning algorithms to predict risk factors for permanent stoma in patients after TME. Front Surg 2023;10:1125875.
59. Kuo CY, Kuo LJ, Lin YK. Artificial intelligence based system for predicting permanent stoma after sphincter saving operations. Sci Rep 2023;13:16039.
60. Keane C, Fearnhead NS, Bordeianou LG, et al; LARS International Collaborative Group. International consensus definition of low anterior resection syndrome. Dis Colon Rectum 2020;63:274-84.
61. Qin Q, Huang B, Wu A, et al; Chinese Radiation Intestinal Injury Research Group. Development and validation of a post-radiotherapy prediction model for bowel dysfunction after rectal cancer resection. Gastroenterology 2023;165:1430-42.e14.
62. Huang MJ, Ye L, Yu KX, et al. Development of prediction model of low anterior resection syndrome for colorectal cancer patients after surgery based on machine-learning technique. Cancer Med 2023;12:1501-19.
63. Wang Z, Shao SL, Liu L, Lu QY, Mu L, Qin JC. Machine learning model for prediction of low anterior resection syndrome following laparoscopic anterior resection of rectal cancer: a multicenter study. World J Gastroenterol 2023;29:2979-91.
64. Wang K, Tang Y, Zhang F, Guo X, Gao L. Combined application of inflammation-related biomarkers to predict postoperative complications of rectal cancer patients: a retrospective study by machine learning analysis. Langenbecks Arch Surg 2023;408:400.
65. Lin V, Tsouchnika A, Allakhverdiiev E, et al. Training prediction models for individual risk assessment of postoperative complications after surgery for colorectal cancer. Tech Coloproctol 2022;26:665-75.
66. Wei R, Guan X, Liu E, et al. Development of a machine learning algorithm to predict complications of total laparoscopic anterior resection and natural orifice specimen extraction surgery in rectal cancer. Eur J Surg Oncol 2023;49:1258-68.
67. Merath K, Hyer JM, Mehta R, et al. Use of machine learning for prediction of patient risk of postoperative complications after liver, pancreatic, and colorectal surgery. J Gastrointest Surg 2020;24:1843-51.
68. Francis NK, Luther A, Salib E, et al. The use of artificial neural networks to predict delayed discharge and readmission in enhanced recovery following laparoscopic colorectal cancer surgery. Tech Coloproctol 2015;19:419-28.
69. Manilich E, Vogel JD, Kiran RP, Church JM, Seyidova-Khoshknabi D, Remzi FH. Key factors associated with postoperative complications in patients undergoing colorectal surgery. Dis Colon Rectum 2013;56:64-71.
70. Liu Y, Song C, Tian Z, Shen W. Identification of high-risk patients for postoperative myocardial injury after CME using machine learning: a 10-year multicenter retrospective study. Int J Gen Med 2023;16:1251-64.
71. Chen KA, Joisa CU, Stem JM, Guillem JG, Gomez SM, Kapadia MR. Improved prediction of surgical-site infection after colorectal surgery using machine learning. Dis Colon Rectum 2023;66:458-66.
72. Ohno Y, Mazaki J, Udo R, et al. Preliminary evaluation of a novel artificial intelligence-based prediction model for surgical site infection in colon cancer. Cancer Diagn Progn 2022;2:691-6.
73. Masum S, Hopgood A, Stefan S, Flashman K, Khan J. Data analytics and artificial intelligence in predicting length of stay, readmission, and mortality: a population-based study of surgical management of colorectal cancer. Discov Oncol 2022;13:11.
74. Ashraf Ganjouei A, Romero-Hernandez F, Conroy PC, et al. A novel machine learning approach to predict textbook outcome in colectomy. Dis Colon Rectum 2024;67:322-32.
75. Guidolin K, Ng D, Zorigtbaatar A, Chadi S, Quereshy F. A machine learning model to predict the need for conversion of operative approach in patients undergoing colectomy for neoplasm. Cancer Rep 2024;7:e1917.
76. Ruan X, Fu S, Storlie CB, Mathis KL, Larson DW, Liu H. Real-time risk prediction of colorectal surgery-related post-surgical complications using GRU-D model. J Biomed Inform 2022;135:104202.
77. Weller GB, Lovely J, Larson DW, Earnshaw BA, Huebner M. Leveraging electronic health records for predictive modeling of post-surgical complications. Stat Methods Med Res 2018;27:3271-85.
78. Chen D, Afzal N, Sohn S, et al. Postoperative bleeding risk prediction for patients undergoing colorectal surgery. Surgery 2018;164:1209-16.
79. Soguero-Ruiz C, Hindberg K, Rojo-Alvarez JL, et al. Support vector feature selection for early detection of anastomosis leakage from bag-of-words in electronic health records. IEEE J Biomed Health Inform 2016;20:1404-15.
80. Jo YY, Han J, Park HW, et al. Prediction of prolonged length of hospital stay after cancer surgery using machine learning on electronic health records: retrospective cross-sectional study. JMIR Med Inform 2021;9:e23147.
81. Strömblad CT, Baxter-King RG, Meisami A, et al. Effect of a predictive model on planned surgical duration accuracy, patient wait time, and use of presurgical resources: a randomized clinical trial. JAMA Surg 2021;156:315-21.
82. He K, Zhang X, Ren S, Sun J. Deep residual learning for image recognition. ArXiv. [Preprint.] Dec 10, 2015 [accessed on 2024 Oct 30]. Available from: https://doi.org/10.48550/arXiv.1512.03385.
Cite This Article
How to Cite
Celotto, F.; Capelli, G.; Ferrari, S.; Scarpa, M.; Pucciarelli, S.; Spolverato, G. Application and use of artificial intelligence in colorectal cancer surgery: where are we?. Art. Int. Surg. 2024, 4, 348-63. http://dx.doi.org/10.20517/ais.2024.26
Download Citation
Export Citation File:
Type of Import
Tips on Downloading Citation
Citation Manager File Format
Type of Import
Direct Import: When the Direct Import option is selected (the default state), a dialogue box will give you the option to Save or Open the downloaded citation data. Choosing Open will either launch your citation manager or give you a choice of applications with which to use the metadata. The Save option saves the file locally for later use.
Indirect Import: When the Indirect Import option is selected, the metadata is displayed and may be copied and pasted as needed.
About This Article
Copyright
Data & Comments
Data
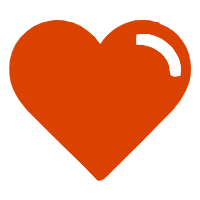
Comments
Comments must be written in English. Spam, offensive content, impersonation, and private information will not be permitted. If any comment is reported and identified as inappropriate content by OAE staff, the comment will be removed without notice. If you have any queries or need any help, please contact us at support@oaepublish.com.