Webinar
Contents
Host
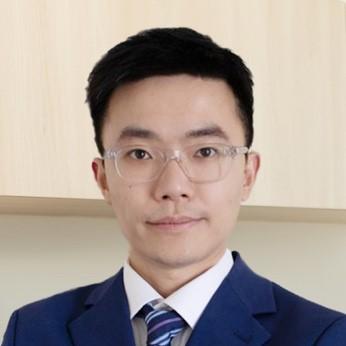
Dr. Pengfei Ou
Department of Chemistry, National University of Singapore, Singapore.
Speaker(s)
Prof. Hao Li
Advanced Institute for Materials Research (WPI-AIMR), Tohoku University, Japan.
Topic: Latest Advances in the “Materials Turing Scheme”: AI + Theory-Driven Framework for Efficient Functional Materials Design
Topic: Latest Advances in the “Materials Turing Scheme”: AI + Theory-Driven Framework for Efficient Functional Materials Design
Abstract:
This talk will present the latest progress of the “Materials Turing Scheme” led by the Hao Li Lab at WPI-AIMR, focusing on AI-driven frameworks for materials design. We will explore the development of a digital platform that integrates large-scale data mining, predictive modeling, computational methodologies, and automated proof-of-concept experiments.
Key highlights include:
i) Our Digital Catalysis Platform 3.0 (DigCat 3.0)—the first comprehensive heterogeneous electrocatalysis database—built through large-scale experimental data mining and large language model training.
ii) Strategies to reduce complexity in materials design, particularly for catalysis, battery, and hydrogen storage, by applying advanced materials theory.
iii) The development of novel computational methods (including cloud-based platforms, models, algorithms, and software packages) to accelerate materials simulation.
This talk will showcase the predictive capabilities of theory in fields such as electrochemical and thermal catalysis, solid-state battery electrolytes, and hydrogen storage materials. Finally, we will discuss our ongoing efforts to establish a global network for automatic synthesis, guided by our AI-powered platform.
Bio:
Prof. Hao Li, Associate Professor at the Advanced Institute for Materials Research (WPI-AIMR), Tohoku University, Japan, and head of the Digital Catalysis & Battery Lab (DigCat & DigBat). He is also a collaborating researcher at the Australian Research Council Centre of Excellence for Green Electrochemical CO2 Transformation (GETCO2). He received his Ph.D. from the Department of Chemistry and the Oden Institute for Computational Engineering and Sciences at the University of Texas at Austin and completed postdoctoral work at the Department of Physics, Technical University of Denmark.
His research focuses on AI-driven materials science, catalyst and materials theory development, theoretical computational methods and machine learning algorithms, and the design of novel catalysts. He has received numerous international awards and honors, including the "Young Scholar in Surface Science" recognition (2022 ACS Annual Meeting) and the "Best Fundamental Research of the Year" award by the American Institute of Chemical Engineers (AIChE). He was also named a Highly Cited Researcher for 2021–2024.
He has published over 230 papers in leading journals such as Nature Catalysis, Nature Communications, Nature Sustainability, JACS, Angewandte Chemie International Edition, Advanced Materials, PNAS, Chemical Science, and ACS Catalysis, with more than 10,000 citations to date. He has been invited to deliver over 90 talks at renowned universities and top-tier conferences worldwide.
This talk will present the latest progress of the “Materials Turing Scheme” led by the Hao Li Lab at WPI-AIMR, focusing on AI-driven frameworks for materials design. We will explore the development of a digital platform that integrates large-scale data mining, predictive modeling, computational methodologies, and automated proof-of-concept experiments.
Key highlights include:
i) Our Digital Catalysis Platform 3.0 (DigCat 3.0)—the first comprehensive heterogeneous electrocatalysis database—built through large-scale experimental data mining and large language model training.
ii) Strategies to reduce complexity in materials design, particularly for catalysis, battery, and hydrogen storage, by applying advanced materials theory.
iii) The development of novel computational methods (including cloud-based platforms, models, algorithms, and software packages) to accelerate materials simulation.
This talk will showcase the predictive capabilities of theory in fields such as electrochemical and thermal catalysis, solid-state battery electrolytes, and hydrogen storage materials. Finally, we will discuss our ongoing efforts to establish a global network for automatic synthesis, guided by our AI-powered platform.
Bio:
Prof. Hao Li, Associate Professor at the Advanced Institute for Materials Research (WPI-AIMR), Tohoku University, Japan, and head of the Digital Catalysis & Battery Lab (DigCat & DigBat). He is also a collaborating researcher at the Australian Research Council Centre of Excellence for Green Electrochemical CO2 Transformation (GETCO2). He received his Ph.D. from the Department of Chemistry and the Oden Institute for Computational Engineering and Sciences at the University of Texas at Austin and completed postdoctoral work at the Department of Physics, Technical University of Denmark.
His research focuses on AI-driven materials science, catalyst and materials theory development, theoretical computational methods and machine learning algorithms, and the design of novel catalysts. He has received numerous international awards and honors, including the "Young Scholar in Surface Science" recognition (2022 ACS Annual Meeting) and the "Best Fundamental Research of the Year" award by the American Institute of Chemical Engineers (AIChE). He was also named a Highly Cited Researcher for 2021–2024.
He has published over 230 papers in leading journals such as Nature Catalysis, Nature Communications, Nature Sustainability, JACS, Angewandte Chemie International Edition, Advanced Materials, PNAS, Chemical Science, and ACS Catalysis, with more than 10,000 citations to date. He has been invited to deliver over 90 talks at renowned universities and top-tier conferences worldwide.
Dr. Pengfei Ou
Department of Chemistry, National University of Singapore, Singapore.
Topic: Machine Learning-driven Electrocatalysts Design for a Sustainable Future
Topic: Machine Learning-driven Electrocatalysts Design for a Sustainable Future
Abstract:
Catalyst design is essential for advancing renewable energy storage and utilization. Due to the diverse elemental and structural nature of catalyst active sites, the chemical space is vast, and comprehensive datasets remain limited. Key scientific questions persist regarding the optimal modelling of catalyst activity and efficient screening methods across extensive chemical space. In this talk, I will discuss the concepts of data-driven and machine learning (ML) enabled approaches for catalyst design and discovery using reactivity descriptors and scaling relations. Specifically, I will introduce the ML workflow developed based on the density functional theory (DFT) calculations to predict activity and stability and understand the underlying mechanism for the basal plane activation, which originates from the electronic effect, in two-dimensional transition metal dichalcogenides (2D TMDC) alloys. Moving to high-entropy alloys (HEA), I will showcase how we introduced adsorption site similarity into a graph neural network and achieved a 2× acceleration in ML training, reducing the workload of generating the dataset using computational-intensive DFT calculations. This approach was validated with a successful experimental identification of HEA electrocatalyst for the oxygen reduction reaction, outperforming the traditional Pt/C.
Bio:
Dr. Pengfei Ou joined the Department of Chemistry at the National University of Singapore (NUS) as a Presidential Young Professor in August 2024. He received his Ph.D. from McGill University in 2020 (advisor: Professor Jun Song). He was a postdoctoral fellow at the University of Toronto from 2020 to 2022 and a Research Associate at Northwestern University from 2022 to 2024, under the guidance of Professor Edward H. Sargent. Dr. Ou’s research is focused on computational materials science, computational catalysis, and machine learning. He has published papers in prestigious journals such as Nat. Energy, Nat. Catal., Nat. Commun., J. Am. Chem. Soc., Sci. Adv., and Proc. Natl. Acad. Sci, as first or corresponding author. To date, he has authored over 80 papers with >4,300 citations and an H-index of 37. He has received honors such as the Climate Positive Energy Postdoctoral Fellowship from the University of Toronto and the Chinese Government Award for Outstanding Self-financed Students Abroad.
Catalyst design is essential for advancing renewable energy storage and utilization. Due to the diverse elemental and structural nature of catalyst active sites, the chemical space is vast, and comprehensive datasets remain limited. Key scientific questions persist regarding the optimal modelling of catalyst activity and efficient screening methods across extensive chemical space. In this talk, I will discuss the concepts of data-driven and machine learning (ML) enabled approaches for catalyst design and discovery using reactivity descriptors and scaling relations. Specifically, I will introduce the ML workflow developed based on the density functional theory (DFT) calculations to predict activity and stability and understand the underlying mechanism for the basal plane activation, which originates from the electronic effect, in two-dimensional transition metal dichalcogenides (2D TMDC) alloys. Moving to high-entropy alloys (HEA), I will showcase how we introduced adsorption site similarity into a graph neural network and achieved a 2× acceleration in ML training, reducing the workload of generating the dataset using computational-intensive DFT calculations. This approach was validated with a successful experimental identification of HEA electrocatalyst for the oxygen reduction reaction, outperforming the traditional Pt/C.
Bio:
Dr. Pengfei Ou joined the Department of Chemistry at the National University of Singapore (NUS) as a Presidential Young Professor in August 2024. He received his Ph.D. from McGill University in 2020 (advisor: Professor Jun Song). He was a postdoctoral fellow at the University of Toronto from 2020 to 2022 and a Research Associate at Northwestern University from 2022 to 2024, under the guidance of Professor Edward H. Sargent. Dr. Ou’s research is focused on computational materials science, computational catalysis, and machine learning. He has published papers in prestigious journals such as Nat. Energy, Nat. Catal., Nat. Commun., J. Am. Chem. Soc., Sci. Adv., and Proc. Natl. Acad. Sci, as first or corresponding author. To date, he has authored over 80 papers with >4,300 citations and an H-index of 37. He has received honors such as the Climate Positive Energy Postdoctoral Fellowship from the University of Toronto and the Chinese Government Award for Outstanding Self-financed Students Abroad.