Webinar
Contents
Chair
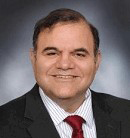
Dr. Kelly Cohen
Speaker(s)
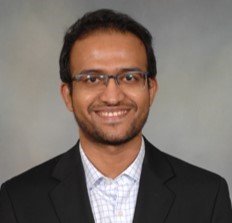
Dr. Anoop Sathyan
Talk Title:
Interpretable AI for Bio-medical Applications
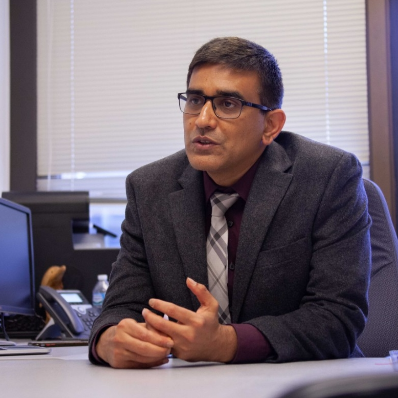
Dr. Manish Kumar
Personal Summary
Manish Kumar received his Bachelor of Technology degree in Mechanical Engineering from Indian Institute of Technology, Kharagpur, India in 1998, and his M.S. and Ph.D. degrees in Mechanical Engineering from Duke University, NC, USA in 2002 and 2004 respectively. After finishing his Ph.D., he served as a postdoctoral researcher in the Department of Mechanical Engineering and Materials Science at Duke University, the US Army Research Office, and General Robotics, Automation, Sensing, and Perception (GRASP) laboratory at the University of Pennsylvania, PA, USA. He started his career as a faculty member at University of Cincinnati (UC) in 2007 in the Department of Mechanical and Materials Engineering where he currently serves as a Professor and the Graduate Program Director. He established Industry 4.0/5.0 Institute which is consortium of industry members engaged in researching and developing advanced technologies for solving key challenges facing the industry. He currently directs Cooperative Distributed Systems (CDS) Laboratory, co-directs Industry 4.0/5.0 Institute, and co-directs UAV MASTER Lab. His research interests include Unmanned Aerial Vehicles, robotics, AI/ML, decision-making and control in complex systems, AI, multi-sensor data fusion, swarm systems, and multiple robot coordination and control. His research has been supported by funding obtained from National Science Foundation, Department of Defense, Ohio Department of Transportation, Ohio Department of Higher Education, Ohio Bureau of Workers Compensation, and several industrial partners. He is a member of the American Society of Mechanical Engineers (ASME). He has served as the Chair of the Robotics Technical Committee of the ASME’s Dynamic Systems and Control Division, and as Associate Editor for the ASME Journal of Dynamic Systems, Measurements and Control and ASME Journal of Autonomous Vehicles and Systems.
Talk Title:
Active manipulation of a tethered drone using explainable AI
Talk Abstract:
Tethered drones are currently finding a wide range of applications such as for aerial surveillance, traffic monitoring, and setting up ad-hoc communication networks. However, many technological gaps are required to be addressed for such systems. Most commercially available tethered drones hover at a certain position; however, the control task becomes challenging when the ground robot or station needs to move. In such a scenario, the drone is required to coordinate its motion with the moving ground vehicle without which the tether can destabilize the drone. Another challenging aspect is when the system is required to operate in GPS denied environments, such as in planetary exploration. In this presentation, to address these issues, we take advantage of passive or force-based control in which the tension in the tether is sensed and used to drive the drone. Fuzzy logic is used to implement the force-based controller as a tool for explainable Artificial Intelligence. The proposed fuzzy logic controller takes tether force and its rate of change as the inputs and provides desired attitudes as the outputs. Via simulations and experiments, we show that the proposed controller allows effective coordination between the drone and the moving ground rover. The rule-based feature of fuzzy logic provides linguistic explainability for its decisions. Simulation and experimental results are provided to validate the novel controller. This presentation additionally discusses an adaptive controller for estimating unknown constant winds on these tethered drone systems using a proportional controller. The simulation results demonstrate the effectiveness of the proposed adaptive control scheme in addressing the effect of wind on a tethered drone.
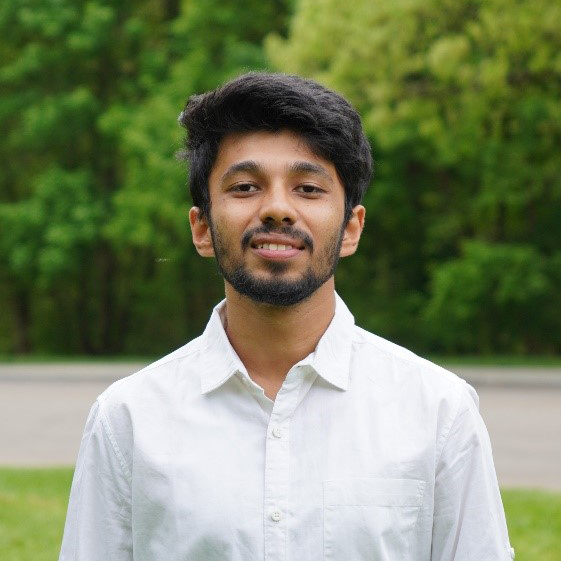
Shurendher Kumar Sampathkumar
Personal Summary:
Shurendher is a master's student pursuing his studies in the Department of Mechanical Engineering at the University of Cincinnati. He earned his bachelor's degree in mechanical engineering from Anna University, India, in 2019. With a keen interest in advancing the field of robotics, Shurendher's research focus revolves around human-robot interaction, robot motion planning, and control of autonomous systems. Currently, he is working on developing a human-aware navigation framework for autonomous mobile robots.
Talk Title:
Human-Aware Navigation Framework for Autonomous Mobile Robots in Public Spaces
Talk Abstract:
As the deployment of Autonomous Mobile Robots (AMRs) transitions from industrial environments to public spaces like airports and shopping malls, ensuring their efficient operation, as well as safe and comfortable coexistence with humans, becomes essential. However, conventional AMR navigation approaches usually focus on collision avoidance and operational efficiency without considering human-related factors, which can result in discomfort and/or unsafe situations for humans. To address these challenges, this work proposes a framework designed to prioritize human safety, comfort, and acceptance during robot interactions in shared environments, called Human-Aware Navigation (HAN). The proposed HAN framework is primarily based on the Enhanced Potential Field (EPF) method, coupled with a Fuzzy Inference System (FIS). While the EPF plays a critical role in resolving common challenges in collision-free navigation, such as local minima and obstacle avoidance near goals, the FIS adaptively determines the EPF’s coefficients by considering human and environmental factors. Additionally, the AMR’s realistic and efficient movements are achieved by accounting for the AMR’s physical constraints using the unicycle kinematic model and an energy-balanced turning approach. The performance of the proposed framework is validated in diverse, human-rich scenarios, and the results demonstrate that AMRs can safely navigate cluttered environments while maintaining human comfort and adhering to operational constraints.
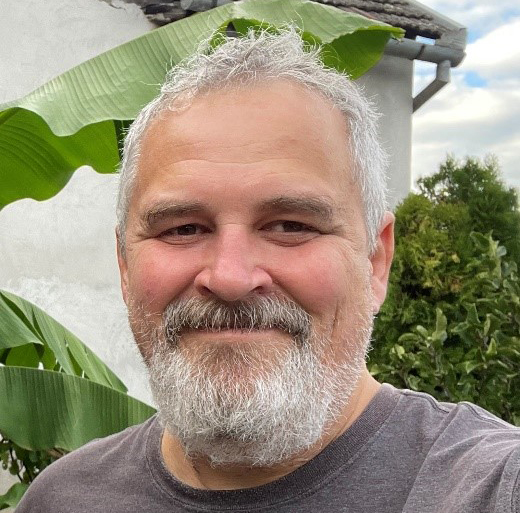
Dr. Barnabas Bede
Personal Summary:
Dr. Barnabas Bede earned his Ph.D. in Mathematics from Babes-Bolyai University of Cluj-Napoca, Romania. His research interests include Machine Learning, Fuzzy Sets and Fuzzy Logic, and Modeling under Uncertainty. He is a Professor of Mathematics at DigiPen Institute of Technology in Redmond, WA, USA, and he serves as Program Director of the Bachelor of Science in Computer Science in Machine Learning. Before that, he held positions at the University of Rio Grande Valley, Texas, the University of Texas at El Paso, the University of Oradea Romania, and Óbuda University in Hungary. He has published more than 100 research publications, including three research monographs.
Talk Title:
Reinforcement learning with Takagi-Sugeno-Kang fuzzy systems
Talk Abstract:
In this talk, we will explore the construction of novel fuzzy-based explainable machine learning algorithms and training of such models using reinforcement learning. Fuzzy systems are widely used in modeling uncertainty and are based on fuzzy rules describing connections between various variables in this setting. We will start by studying the equivalence between a layer of a Neural Network with ReLU activation and a Takagi-Sugeno (TS) fuzzy system with triangular membership function.
We will discuss applications of the interpretable Machine Learning algorithms introduced here to physics engines used in video games. We will also explore the equivalence between neural networks with multiple layers and multiple inputs with ReLU activation, and Takagi-Sugeno systems with similar multi-layer structure. This method allows us to translate a Neural Network into a Fuzzy Systems, potentially extracting fuzzy rules that can make neural networks more interpretable. Future research directions are also explored.
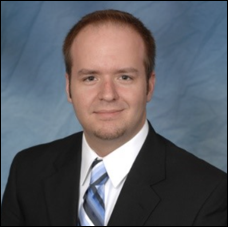
Dr. Timothy Arnett
Personal Summary:
Dr. Arnett is a Senior AI Researcher and Verification Lead at the Thales Avionics office in Cincinnati. He earned his PhD at the University of Cincinnati, where his graduate work focused on efficient machine learning and the formal verification of Fuzzy Systems for aerospace applications. At Thales, he has continued to concentrate on these areas, applying them to the development of the TrUE (Transparent, Understandable, and Ethical) AI toolkit. The TrUE AI toolkit is a Genetic Fuzzy Tree-based machine learning SDK, primarily designed for autonomy, planning, and control in scenarios where explainability, transparency, and formal verification for certification are crucial.
Dr. Arnett is also actively involved in the Fuzzy research community, contributing to both IEEE CIS and NAFIPS. Additionally, he organizes an annual student-focused competition, the Explainable Fuzzy Challenge (XFC), which promotes student development and understanding of key topics related to Fuzzy Logic and Machine Learning.
Talk Title:
Topics revolving the material in the paper titled Formal Verification of Fuzzy-based XAI for Strategic Combat Game.
Talk Abstract:
Explainable AI is a topic at the forefront of the field currently for reasons involving human trust in AI, correctness, auditing, knowledge transfer, and regulation. AI that is developed with Reinforcement Learning (RL) is especially of interest due to the non-transparency of what was learned from the environment. RL AI systems have been shown to be "brittle" with respect to the conditions it can safely operate in, and therefore ways to show correctness regardless of input values are of key interest. One way to show correctness is to verify the system using Formal Methods, known as Formal Verification. These methods are valuable, but costly and difficult to implement, leading most to instead favor other methodologies for verification that may be less rigorous, but more easily implemented. In this work, we show methods for development of an RL AI system for aspects of the strategic combat game Starcraft 2 that is performant, explainable, and formally verifiable. The resulting system performs very well on example scenarios while retaining explainability of its actions to a human operator or designer. In addition, it is shown to adhere to formal safety specifications about its behavior.
Introduction
Relatd Articles:
Active manipulation of a tethered drone using explainable AI
http://dx.doi.org/10.20517/ces.2023.33
Fuzzy inference system-assisted human-aware navigation framework based on enhanced potential field
http://dx.doi.org/10.20517/ces.2023.34
Reinforcement learning with Takagi-Sugeno-Kang fuzzy systems
http://dx.doi.org/10.20517/ces.2023.11
Formal verification of Fuzzy-based XAI for Strategic Combat Game
http://dx.doi.org/10.20517/ces.2022.54
Interpretable AI for bio-medical applications
http://dx.doi.org/10.20517/ces.2022.41
Time (EST, UTC-4) | Speakers | Topics |
10:00-10:10 AM | Dr. Kelly Cohen | Welcome Remarks |
10:10-10:25 AM | Dr Anoop Sathyan | Interpretable AI for Bio-medical Applications |
10:25-10:30 AM | All | Discussion (Q&A) |
10:30-10:45 AM | Dr Manish Kumar | Active manipulation of a tethered drone using explainable AI |
10:45-10:50 AM | All | Discussion (Q&A) |
10:50-11:05 AM | Shurendher Kumar Sampathkumar | Human-Aware Navigation Framework for Autonomous Mobile Robots in Public Spaces |
11:05-11:10 AM | All | Discussion (Q&A) |
11:10-11:25 AM | Dr. Barnabas Bede | Reinforcement learning with Takagi-Sugeno-Kang fuzzy systems |
11:25-11:30 AM | All | Discussion (Q&A) |
11:30-11:45 AM | Dr Tim Arnett | Formal Verification of Fuzzy-based XAI for Strategic Combat Game |
11:45-11:50 AM | All | Discussion (Q&A) |
11:50-12:00 AM | Dr. Kelly Cohen | Summarizing |
Relevant Special Issue(s)
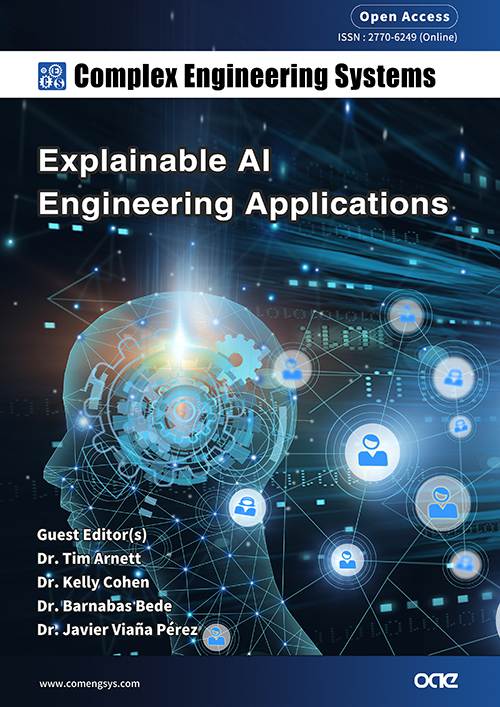
Topic: Explainable AI Engineering Applications
https://www.oaepublish.com/specials/comengsys.1071Moments
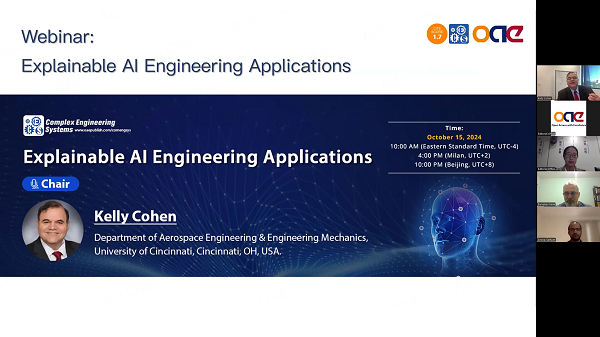
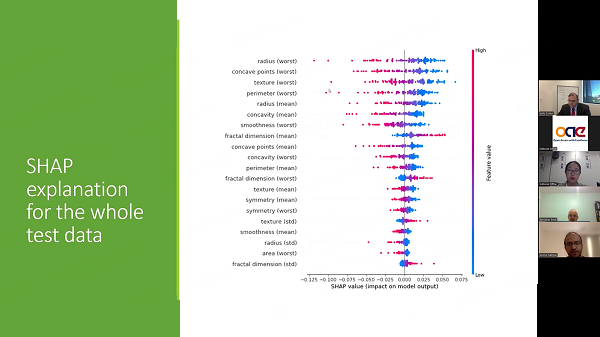
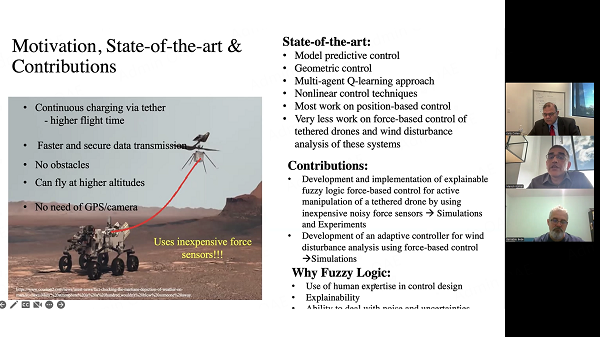
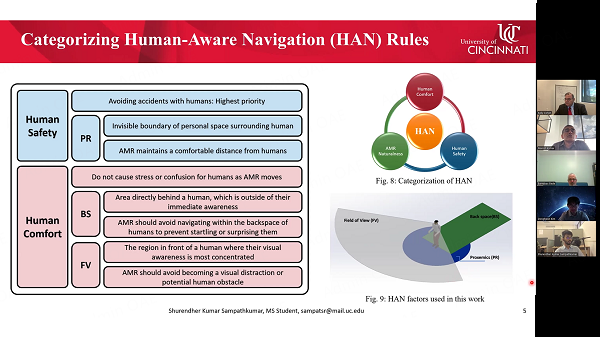
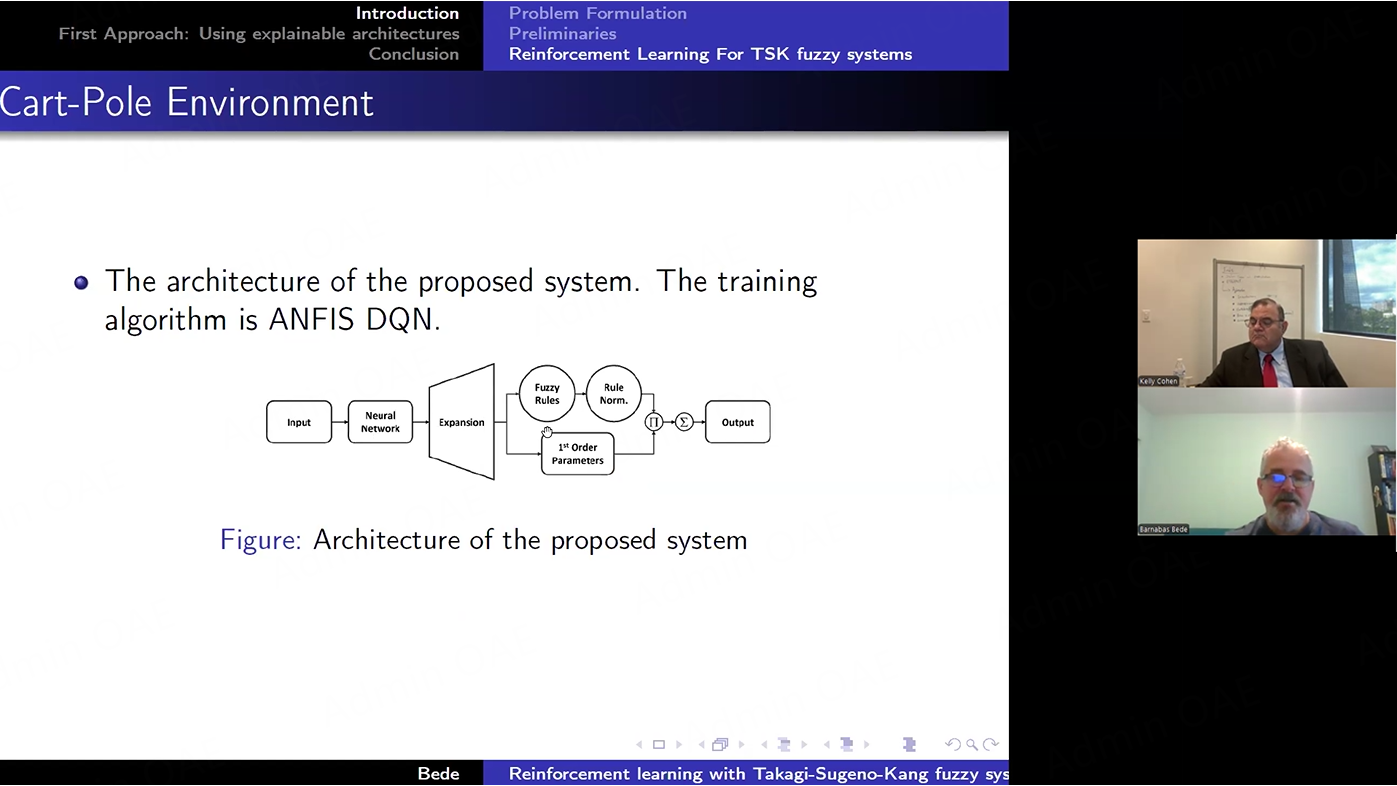
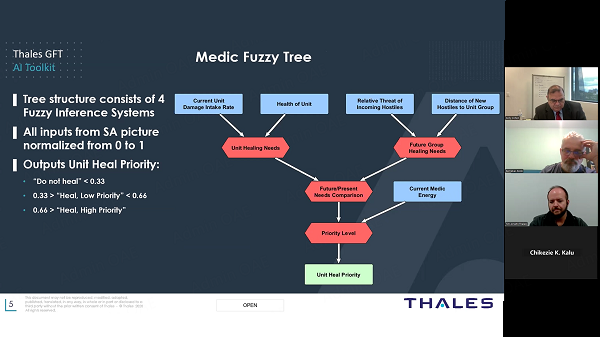
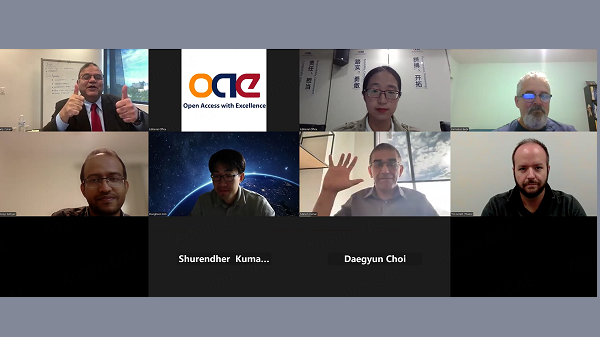
Presentation
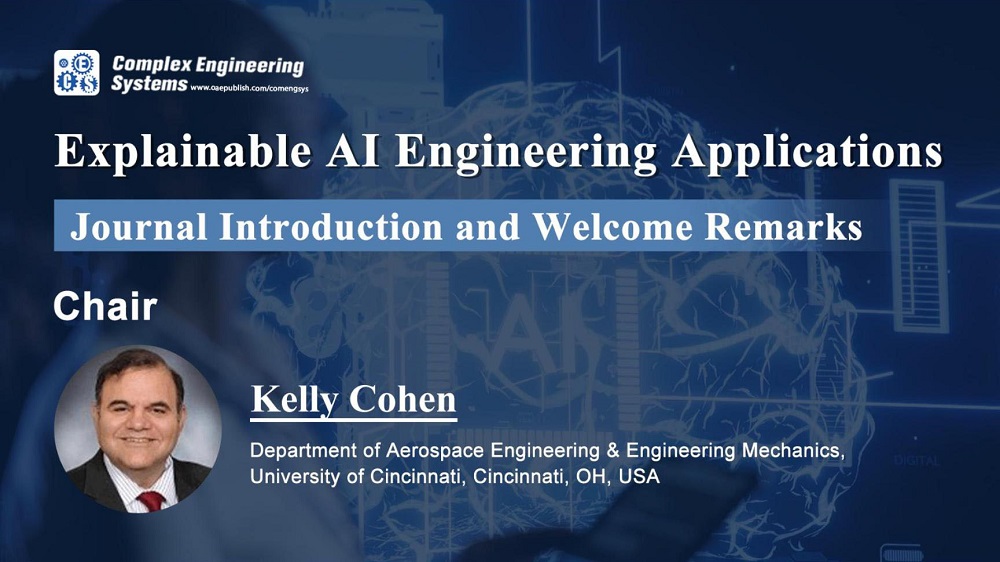
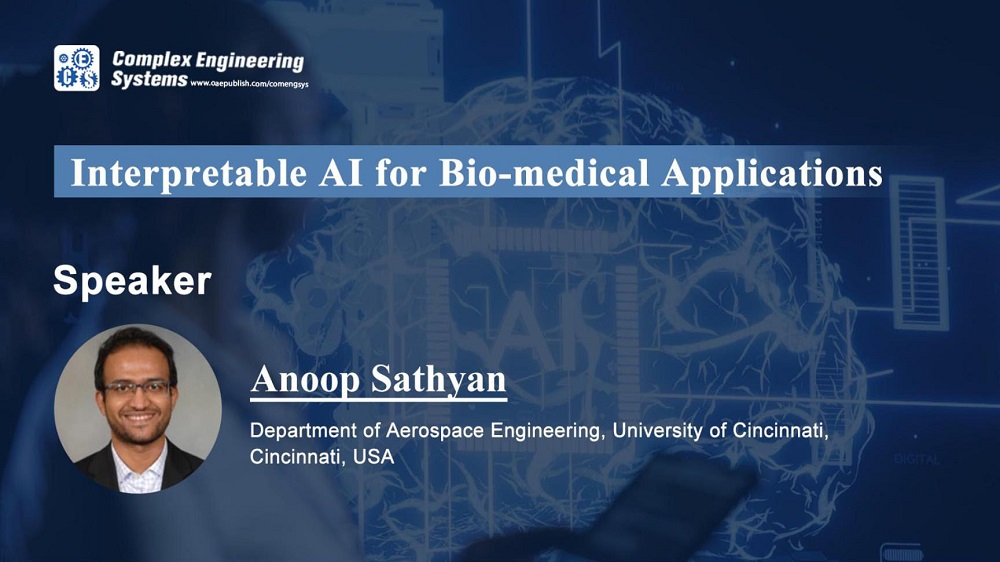
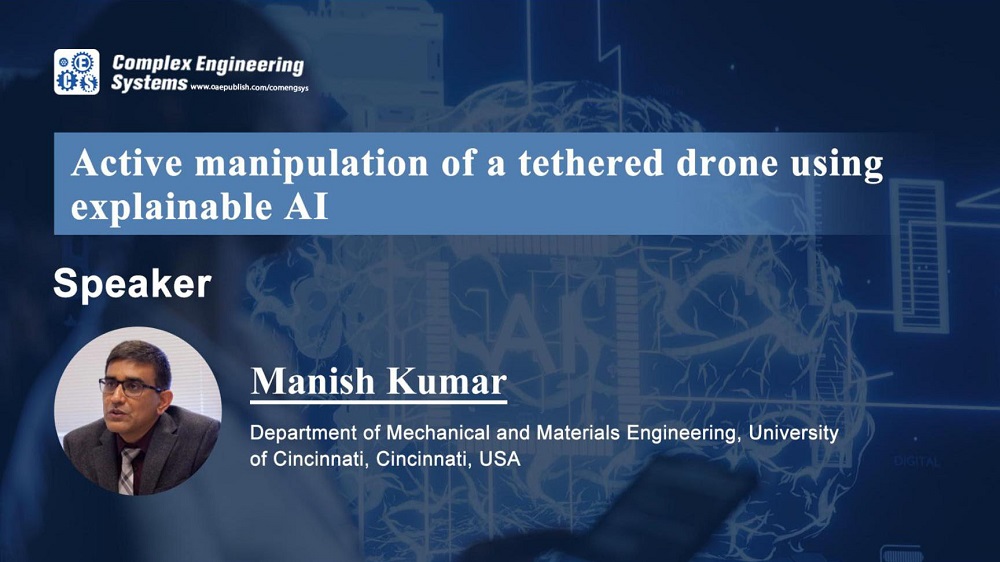
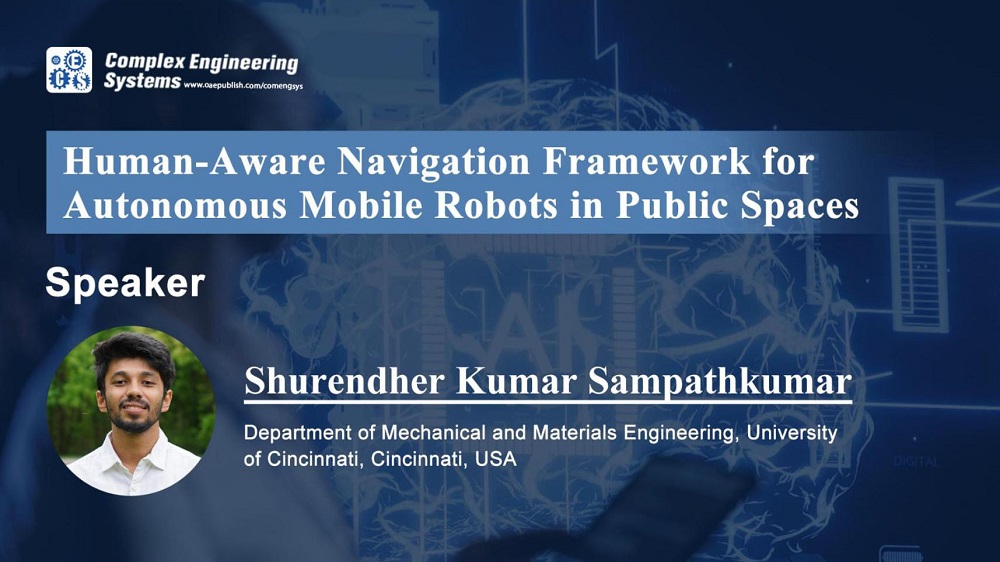
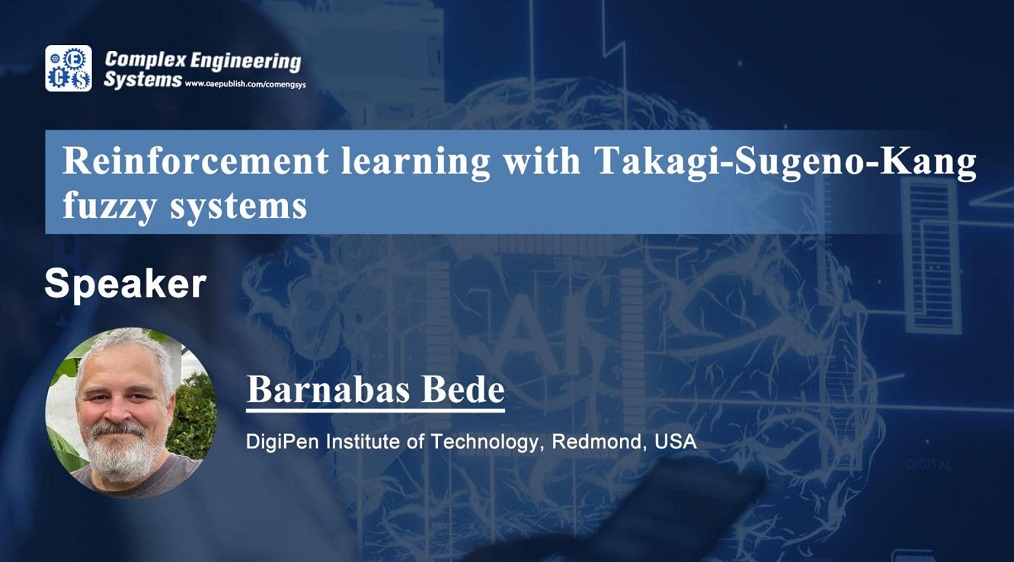
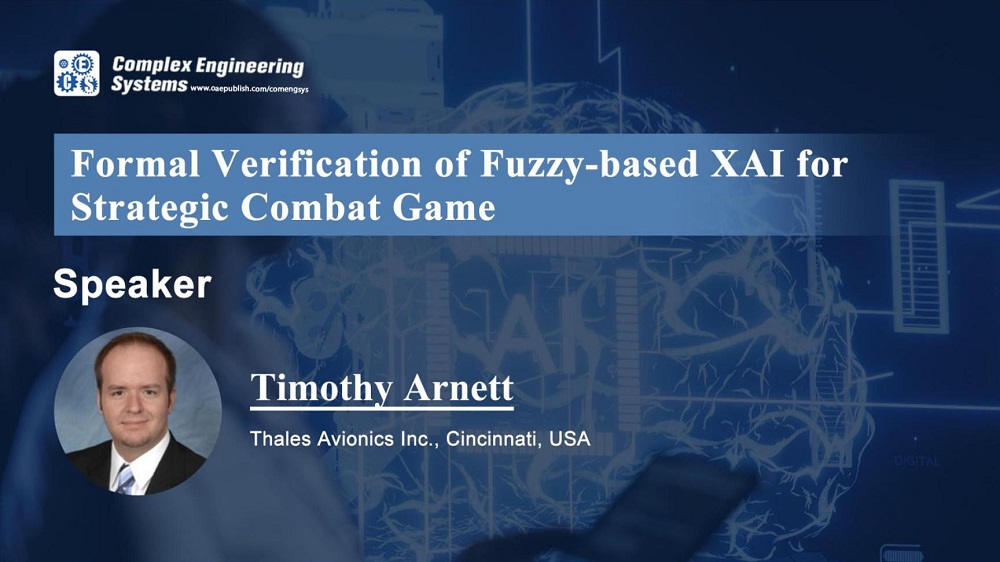
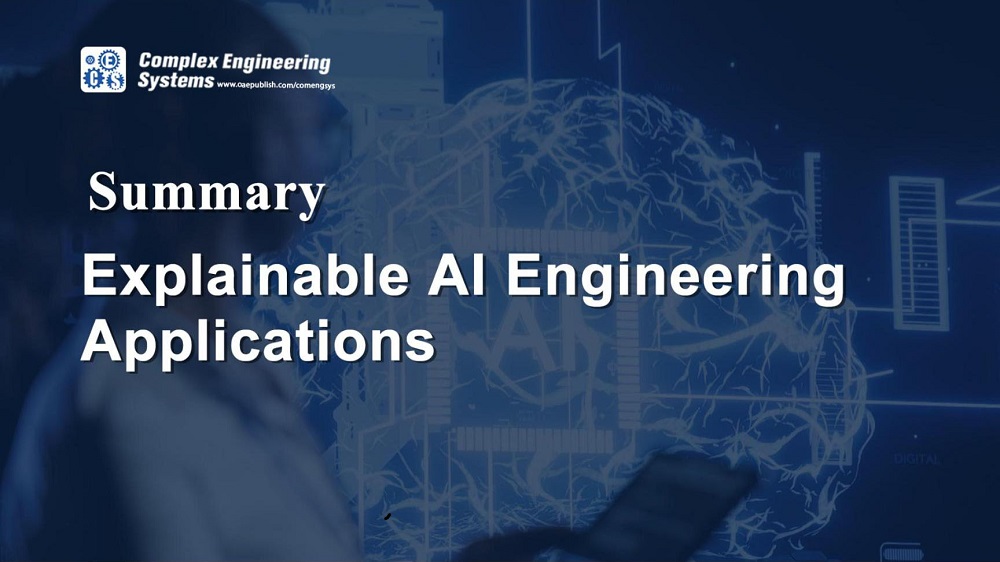