A novel SNP-based approach for non-invasive prenatal paternity testing using multiplex PCR targeted capture sequencing
Abstract
Objective: To enhance the safety, simplicity, and efficacy of non-invasive prenatal paternity testing, we developed a method based on multiplex PCR targeted capture sequencing technology utilizing single nucleotide polymorphisms (SNPs) as genetic markers.
Method: We screened 627 SNPs from public databases and literature based on specific criteria and population genetic data from 100 unrelated individuals. A total of 15 peripheral blood samples were collected from pregnant women and the suspected father. Paternal alleles were detected and analyzed in the plasma cell-free DNA (cfDNA) of pregnant women, fetal SNP genotypes were obtained, and the combined paternity index (CPI) was calculated for paternity testing.
Results: Biological fathers were accurately determined in all cases, with CPI values ranging from 1.05 × 1014 to
Conclusion: This novel approach demonstrates significant improvements by reducing the number of SNPs, streamlining the research procedure, and lowering costs, yielding substantial advancements in non-invasive prenatal paternity testing.
Keywords
INTRODUCTION
Prenatal paternity testing is a crucial component of forensic genetics, which involves determining the genetic relationship between a fetus and a suspected father before birth. In recent years, there has been a significant increase in women’s legal literacy and self-protection awareness, leading to a higher demand for prenatal paternity tests in forensic cases. These cases often involve sensitive situations such as sexual assault, alimony disputes, and inheritance claims. In particular, pregnant women in sexual assault cases frequently seek to confirm paternity early to make informed decisions about continuing the pregnancy. Conventional prenatal paternity testing methods, such as amniocentesis or chorionic villus sampling[1], are invasive, typically performed after 12 weeks of pregnancy, and can pose physical and emotional risks to the mother[2].
The discovery of cell-free fetal DNA (cffDNA) in maternal blood in 1997 marked a breakthrough in prenatal testing[3]. The cffDNA, released from placental cells following apoptosis[4], can be detected as early as two weeks into pregnancy and remains stable in the blood from the sixth week onwards. This allows for earlier and safer non-invasive prenatal paternity testing (NIPPT). Additionally, cffDNA disappears from the mother’s blood within hours after birth, ensuring that results are not influenced by previous pregnancies[5]. These attributes make cffDNA an ideal material for NIPPT, and various methods utilizing cfDNA have since been developed and widely adopted in clinical and forensic settings[6-10].
Short tandem repeat (STR) genotyping is a primary tool for forensic human identification and paternity cases[11]. Initial attempts to apply STR genotyping to cffDNA were unsuccessful, as demonstrated by
Despite its advantages, the biallelic nature of SNPs requires a large number of loci to achieve sufficient discriminatory power, leading to higher costs and complexities[16]. New genetic markers have been proposed to address these limitations. For instance, Moriot et al. suggested using deletion/insertion polymorphisms linked to STR (DIP-STR) in 2019[17], while Ou et al. explored a hybrid system of 60 microhaplotypes (MHs) in 2020[18]. Both methods showed promise but faced challenges in data processing and lacked authoritative guidance, limiting their widespread adoption[19,20].
Given the ongoing need for a reliable NIPPT method, we combined multiplex PCR targeted capture sequencing with an SNP-based approach to develop a novel panel containing 627 SNPs.
In comparison with the research conducted by Chang et al., while maintaining the accuracy of the system, we have minimized the number of required SNPs by setting screening criteria, thereby simplifying the process of data analysis and reducing costs[15]. In addition, the sensitivity of the new system has also been improved. We can obtain accurate results when the proportion of fetal components in the plasma of pregnant women is above 2%. We conducted a genetic survey of 100 unrelated individuals and statistically analyzed the forensic parameters to evaluate the system’s efficacy and forensic value. Additionally, we tested the system on 15 groups of mother, suspected father, and maternal plasma samples to assess its potential for paternity testing. Our results indicate that this method reduces costs, improves detection efficiency, and holds promise for broad application in forensic cases.
METHODS AND MATERIALS
Sample collection
We collected peripheral blood samples from 15 pregnant women and their husbands (or potential biological fathers). All participants were in good health, and relevant information such as maternal age and gestational age was recorded. The study included only singleton pregnancies, with gestational ages at blood sampling ranging from 5 to 15 weeks. Additionally, we collected peripheral blood samples from 100 unrelated individuals (70 males and 30 females). After birth, hospital professionals also collected fetal buccal swabs for validation studies.
Maternal blood samples (approximately 10 mL) were collected using MiniMax cfDNA blood collection tubes (Apostle, USA), while other blood samples (approximately 5 mL) were collected using vacuum blood collection tubes (BD Biosciences, USA). Buccal samples were collected using flocked swabs (BD Biosciences, USA). All participants were of Han Chinese origin, and samples were collected anonymously after obtaining informed consent. The study followed ethical guidelines and the Declaration of Helsinki, with approval from the Ethics Committee of the Academy of Forensic Sciences of China (No. 2019-W8).
DNA extraction
Maternal plasma was isolated from the peripheral blood using a two-step centrifugation process[21]. cfDNA was then extracted from maternal plasma using the MagicPure Cell-Free DNA Kit II (TransGen Biotech, China) and concentrated to 10 μL using an Eppendorf Concentrator Plus (Eppendorf, Germany). Genomic DNA (gDNA) from the buffy coat and peripheral blood samples of parents and unrelated individuals was extracted using the QIAamp DNA Blood Mini Kit (Qiagen, Germany), while gDNA from fetal buccal swabs was extracted using the QIAamp DNA Investigator Kit (Qiagen, Germany). The concentrations of extracted gDNA and cfDNA were measured using the NanoDrop Lite Spectrophotometer (Thermo Fisher Scientific, USA) and the Qubit dsDNA HS Assay Kit with the Qubit 3.0 fluorometer (Thermo Fisher Scientific, USA), respectively, following the manufacturer’s protocols.
Pedigree confirmation by CE-based STR genotyping
To confirm paternity for the 15 sets of trios, traditional STR-based paternity tests were conducted using fetal gDNA from buccal swabs. Samples were amplified with the SifaSTR 23 plex DNA kit (GoldenEye, China) and analyzed using capillary electrophoresis (CE) on the ABI 3500 genetic analyzer (Applied Biosystems, USA). Genotyping data analysis was performed with GeneMapper ID software v.5 (Applied Biosystems, USA). Parentage testing was conducted by calculating the Combined Paternity Index (CPI)[22].
Selection of SNPs
We selected biallelic SNPs from NCBI public databases and literature, focusing on intronic regions with a minor allele frequency (MAF) ≥ 0.3. We excluded SNPs linked to diseases, located within or near functional genomic elements, and less than 5 Mb apart. This yielded 883 candidate SNPs. Multiplex PCR primers were designed using Primer Premier 5.0 software and validated for specificity with Primer-BLAST. In addition, different amplification fragments in multiplex PCR amplification will compete with each other, resulting in an unbalanced amplification of target fragments. Therefore, the amplification efficiencies of all primers for target fragments should be as close as possible under the same conditions. The following design principles should be adhered to: (1) The length of primers should be between 18 and 30 bp; (2) The annealing temperatures should be as close as possible; (3) The GC content should be between 40% and 60%; (4) Nucleotide complementarity at the 3’end should be avoided, and there should be no more than three consecutive bases; and (5) The formation of hairpin structures or primer dimers among primers should be avoided. Each pair of primers was first independently optimized for its reaction conditions. Subsequently, the primers were pooled and mixed in sequence before further optimization. Due to the large number of SNP loci included in the NGS-SNP multiplex detection system constructed in this study, the phenomenon of dimer formation was inevitable, and thus, some SNP loci could not be successfully amplified. Eventually, the primer combination that could amplify the maximum number of SNP loci was selected to construct the system. Finally, these SNPs were tested on 100 unrelated individuals to exclude those SNPs with low genotyping success rates, poor polymorphism, or linkage disequilibrium.
Library preparation and massively parallel sequencing
Using a custom-designed panel (IGMU229V1, iGeneTech, China), DNA libraries were prepared through two rounds of multiplex PCR and bead purification, as shown in Supplementary Table 1. After the sample was homogenized, the first round of multiplex PCR reaction was carried out, and the product was purified with magnetic beads. Subsequently, the adapter sequence was added for the second round of PCR reaction, and the final product was obtained after purification with magnetic beads again. Library concentrations were measured with a Qubit 3.0 Fluorometer using the Qubit dsDNA HS Assay Kit, with acceptable concentrations exceeding 1.0 ng/μL. Library lengths, typically 300-450 bp, were assessed using the Agilent 2100 Bioanalyzer system (Agilent, USA). Sequencing was performed on the NovaSeq 6000 (Illumina) after quantitative and quality assessments.
SNP calling
To ensure data accuracy, raw sequencing reads were filtered to remove adapter sequences, low-quality bases, and short sequences. The procedure of reads filtering involves (1) eliminating sequences with an average base quality value below 20 using an 8 bp sliding window; (2) eliminating adapter sequences at the end of the reads; (3) directly removing the base if the quality value of the first or last base is below 20; and (4) discarding sequences with length less than 40 bp (for paired-end reads) after the processing mentioned above. The cleaned reads were aligned to the human genome reference Hg19 (GRCh37)[23,24], and SNP genotyping was performed using Samtools (version 1.9) and GATK (version 3.8.0)[25].
Data analysis
We analyzed non-maternal allele sequencing reads (NGASRs) in each sample to assess maternal composition and sequencing errors[15], thereby enhancing SNP genotyping accuracy. NGASRs are sequencing reads from alleles that are not maternally inherited. They offer valuable information on other genetic contributors in various genetic analysis contexts, such as DNA microchimerism and population genetics studies. Data analysis was exclusively performed on homozygous SNPs in the maternal gDNA. In addition to 15 maternal plasma samples, six gDNA samples from unrelated individuals were included as the non-pregnancy control group. The control group included three male samples (MC-1, MC-2, MC-3), one female sample with pregnancy experience (FC-1), and two female samples without pregnancy experience (FC-2, FC-3).
Paternity testing primarily focused on autosomal SNPs, with X-SNP and Y-SNP results serving as Supplementary Materials. When an SNP is homozygous in the mother’s gDNA but heterozygous in cfDNA, the non-maternal allele is considered to originate from the father. Conversely, when both the mother and the fetus had the same homozygous genotype, the non-maternal allele in the maternal plasma cfDNA was considered background noise generated during sequencing. The proportion of cffDNA was calculated using the sequencing depth of the fetus-specific alleles relative to the sequencing depth of the maternal-fetal shared alleles in the sequencing data. The formula for calculating the fetal fraction (FF) in cfDNA is:
where dfather represents the sequencing depth of the fetus-specific allele inherited from the father, and dmother denotes the sequencing depth of the allele shared between the fetus and the mother. The FF is also the average of the ratios of 2dfather to total sequencing depth for each SNP.
The SNPs that are homozygous in the maternal gDNA and heterozygous in the cfDNA are selected as effective SNPs for calculating the paternity index (PI) and determining kinship. The PI is an indicator that assesses the strength of genetic evidence in paternity testing[26]. It refers to the likelihood ratio comparing the probability that the alleged father is the biological father to the probability that a random male is the biological father. The combined paternity index (CPI) is the product of PIs from multiple non-linked SNPs. The PI was calculated as:
Mixture studies
We manually constructed a series of mixtures using gDNA from a mother and her fetus with minor components of 0.5%, 1%, 2%, 3%, 5%, 7%, 10%, 20%, 30%, and 50% to simulate cfDNA samples in the actual case. Sequencing was performed using the multiplex detection system developed in this study. The number of effective SNPs obtained from each proportion of simulated samples was subsequently determined to ascertain the minimum fetal fraction threshold required for reliable results.
RESULTS
Construction and systematic evaluation of the multiplex system
For this study, SNPs with sequencing depths below 30× were excluded, resulting in a final system comprising 589 autosomal SNPs (A-SNPs), 12 X-chromosome SNPs (X-SNPs), and 26 Y-chromosome SNPs (Y-SNPs). The primer information of 627 SNPs can be found in Supplementary Table 2. All included SNPs exhibited biallelic polymorphism. Specifically, 490 SNPs exhibited transitions, and 137 SNPs showed transversions. The minor allele frequency (MAF) ranged from 0.0052 to 0.5000. Sequencing data from 100 unrelated individuals revealed that 213 SNPs had an MAF greater than 0.4, 382 SNPs greater than 0.3, and 504 SNPs greater than 0.2.
The distribution of the 627 SNPs across each chromosome is depicted in Figure 1, with chromosome 2 containing the highest number of SNPs (52) and chromosome 22 the fewest (9). Detailed allele frequencies (AF) and forensic population genetic parameters for the 589 A-SNPs among the 100 unrelated individuals are provided in Supplementary Table 3.
Genotyping of fetal SNPs
We performed sequencing on cfDNA and gDNA from 15 pregnant women and 6 control individuals. The control group included three males, two females with pregnancy experience, and one female without previous pregnancy experience. To eliminate the influence of maternal DNA on fetal allele detection, we focused on homozygous SNPs in gDNA. The number of homozygous SNPs in each sample’s gDNA ranged from 327 to 371.
We then calculated the number and proportion of SNPs displaying non-maternal allele sequencing reads (NGASRs) in the plasma. Figure 2 illustrates these results. In the cfDNA from pregnant women, an average of 88.63% of homozygous SNPs in gDNA showed NGASRs. In contrast, 74.99% of SNPs in cfDNA from the control group exhibited NGASRs. The proportion of NGASRs in cfDNA was significantly higher than in the control group. Among the controls, 97.73% to 99.70% of SNPs had an NGASR fraction (number of NGASRs/number of total reads) below 1%, with the average NGASR fraction varying from 0.03% to 0.52%. This consistency across non-pregnant females, males, and females with and without pregnancy experience suggests that NGASR in controls is mainly due to sequencing or mapping errors.
Figure 2. Proportion of SNPs with varying fractions of non-maternal allele-specific reads (NGASRs) in cfDNA from pregnant women and negative controls among total homozygotes. Different colors in the graph represent different ranges of NGASR fractions.
The NGASR fraction pattern in plasma from pregnant women differed significantly from the control group. In pregnant women’s cfDNA, the average NGASR fraction for SNPs ranged from 1.05% to 2.22%, with only 46.84% to 66.2% of SNPs showing an NGASR fraction below 1.0%. In these cases, NGASR likely results from sequencing errors. SNPs with NGASR fractions exceeding 2.0% accounted for 9.18% to 38.64% of the total homozygous SNPs, representing fetal-specific alleles from the father.
To reduce false positives for SNPs in fetal heterozygotes and minimize misjudgment due to sequencing errors, we considered non-maternal alleles as paternal when the allele fraction in plasma exceeded 2%. An effective SNP is defined as one where the mother is homozygous, and the fetus is heterozygous. In this study, we identified 58-152 effective SNPs in each cfDNA sample. Comparing these SNPs with fetal gDNA sequencing results, we found three inconsistencies among 1,646 effective SNPs in 15 cfDNA samples, resulting in an error rate of 0.18%, ranging from 0% to 1.05%. The fetal gDNA sequencing showed the number of mis-detected SNPs ranging from 0 to 17 in 15 cfDNA samples, with a misdetection rate of 2.83%.
Paternity test with CPI
Paternity testing was performed on 15 alleged family cases using effective SNPs from cfDNA compared with paternal gDNA SNP genotypes. This comparison aimed to verify paternal alleles and calculate the CPI value to determine kinship. The logarithm of the CPI [Log10(CPI)] was computed to simplify data analysis and interpretation.
In two cases, mismatches between cfDNA and paternal gDNA were observed: case 2 had 40 mismatched SNPs, and case 6 had 15 mismatched SNPs. The log10 CPI values for these cases were -89.97 and -27.39, respectively, conclusively excluding paternity. In the remaining cases, the cfDNA matched the paternal gDNA, with log10 CPI values ranging from 14.02 to 34.31, confirming paternity. These results are summarized in Table 1.
Summary of sequencing data and results for 15 trios samples
Case | Fetus gender | Maternal age, year | Gestational age (week) | Number of undetected SNPs | Success rate of SNP detection | Number of effective SNPsa | FF | Number of mismatchesb | CPI (NIPPT) (log10)c | Decisions (NIPPT)d | CPI (STR) (log10) | Decisions (STR) |
1 | Female | 27 | 13 | 0 | 100.00% | 131 | 8.37% | 0 | 32.19 | √ | 9.89 | √ |
2 | Male | 21 | 8 | 5 | 99.15% | 95 | 6.21% | 40 | -89.97 | × | -32.67 | × |
3 | Male | 27 | 15 | 2 | 99.66% | 121 | 10.16% | 0 | 30.15 | √ | 8.64 | √ |
4 | Male | 28 | 10 | 4 | 99.32% | 80 | 7.61% | 0 | 18.90 | √ | 8.43 | √ |
5 | Female | 30 | 8 | 0 | 100.00% | 112 | 6.73% | 0 | 21.80 | √ | 9.22 | √ |
6 | Male | 19 | 6 | 0 | 100.00% | 72 | 4.20% | 15 | -27.39 | × | -31.19 | × |
7 | Male | 26 | 13 | 3 | 99.49% | 125 | 7.71% | 0 | 28.75 | √ | 9.52 | √ |
8 | Male | 23 | 8 | 2 | 99.66% | 130 | 3.93% | 0 | 29.40 | √ | 7.76 | √ |
9 | Male | 34 | 13 | 0 | 100.00% | 152 | 7.93% | 0 | 34.31 | √ | 8.57 | √ |
10 | Male | 21 | 6 | 0 | 100.00% | 83 | 4.36% | 0 | 14.02 | √ | 6.14 | √ |
11 | Male | 31 | 13 | 0 | 100.00% | 116 | 10.55% | 0 | 26.32 | √ | 9.56 | √ |
12 | Male | 29 | 5 | 0 | 100.00% | 58 | 4.10% | 0 | 15.32 | √ | 7.02 | √ |
13 | Male | 32 | 10 | 0 | 100.00% | 140 | 7.70% | 0 | 31.52 | √ | 10.04 | √ |
14 | Female | 28 | 12 | 4 | 99.32% | 131 | 9.47% | 0 | 29.57 | √ | 8.63 | √ |
15 | Male | 33 | 11 | 2 | 99.66% | 100 | 7.44% | 0 | 22.87 | √ | 7.94 | √ |
To validate the accuracy of these findings, we used the PCR-CE method, the gold standard for kinship analysis, on postpartum child samples. The NIPPT results were consistent with PCR-CE findings. Further validation involved testing each of the 70 unrelated males as the alleged father in place of the biological father in the 15 family cases. For unrelated individuals, log10 CPI values were all less than -27.98, whereas the log10 CPI values for biological fathers were above 14.02 [Table 2].
CPI for unrelated males as fetal biological fathers in 15 family cases
Case | The number of effective SNPs | Number of mismatches | CPI (log10) | ||
Average | Range | Average | Range | ||
1 | 131 | 47.20 | 29-62 | -110.82 | -58.53~-152.12 |
2 | 95 | 34.44 | 27-46 | -85.44 | -63.55~-116.93 |
3 | 121 | 41.90 | 30-52 | -96.59 | -62.00~-125.89 |
4 | 80 | 29.06 | 20-38 | -67.64 | -43.60~-93.39 |
5 | 112 | 34.07 | 25-43 | -79.13 | -54.76~-103.34 |
6 | 72 | 24.66 | 13-35 | -59.17 | -27.98~-89.46 |
7 | 125 | 41.80 | 33-53 | -95.31 | -68.98~-127.78 |
8 | 130 | 43.24 | 34-57 | -98.35 | -70.56~-138.05 |
9 | 152 | 53.86 | 39-64 | -121 | -87.03~-153.61 |
10 | 83 | 26.09 | 17-37 | -62.66 | -38.16~-91.53 |
11 | 116 | 40.66 | 26-50 | -96.82 | -56.55~-124.63 |
12 | 58 | 21.47 | 14-29 | -52.34 | -31.88~-73.27 |
13 | 140 | 47.06 | 26-62 | -111.25 | -51.64~-153.01 |
14 | 131 | 46.20 | 32-58 | -109.5 | -71.62~-144.20 |
15 | 100 | 36.80 | 25-51 | -88.04 | -55.74~-126.51 |
We defined mismatched SNPs as those where the paternal contribution did not provide non-maternal fetal alleles. The 70 unrelated male individuals had an average of 21.47 to 53.86 mismatch SNPs, with an average mismatch rate of 30.42% to 37.02%. In contrast, no mismatch SNPs were observed in the biological fathers of the fetuses. The results demonstrate significant differences between the alleged fathers and unrelated males in all samples, indicating the high accuracy of this approach for paternity testing [Figure 3].
Figure 3. Log10 CPI values for alleged fathers and unrelated males in 15 family cases. The red circles represent the alleged fathers corresponding to cfDNA, while the blue dots represent unrelated males. The box plots display each group's median, 25th percentile, 75th percentile, upper boundary, and lower boundary, providing a comprehensive description of the overall data distribution.
Sensitivity
A series of mixed samples with minor components of 0.5%, 1%, 2%, 3%, 5%, 7%, 10%, 20%, 30%, and 50% were artificially constructed to simulate cfDNA samples in actual cases to further assess the sensitivity of the method in detecting a low proportion of FF. We established a detection threshold of 30× for the paternal allele, and 130 effective SNPs were detected in the simulated trio sample through sequencing.
As the proportion of the minor components increased from 0.5% to 50%, the number of effective SNPs rose from 7 to 129 [Figure 4]. When the minor component was below 5%, there was a significant correlation between the number of effective SNPs and the proportion of minor components. At a 4% minor component level, over 99% of effective SNPs could be detected. Reducing the minor component to 2% still allowed for the detection of 98 effective SNPs, yielding a CPI value of 2.89 × 1013, which is sufficient to determine paternity.
Figure 4. Detection of different proportions of minor components. The X-axis represents the fetal fraction, the left Y-axis (blue line) indicates the number of effective SNPs, and the right Y-axis (red line) denotes the log10 CPI value. The graph demonstrates how the number of effective SNPs and log10 CPI value change with varying fetal fractions.
However, when the minor component was reduced to 1%, only 33 SNPs could be identified, resulting in a CPI value of 73.2, which is insufficient to establish paternity. Thus, our method demonstrates high sensitivity and can accurately determine paternity with minor components as low as 2%, but below this threshold, the detection capability significantly diminishes.
Detection and analysis of Y-SNP in maternal plasma
The detection of Y-SNP alleles in maternal plasma provides crucial information about paternal-inherited alleles since Y-SNPs are absent in the maternal genome[27,28]. If Y-SNP reads are found in cfDNA with an average read depth greater than 30×, these reads are considered to originate from the fetus rather than due to sequencing errors.
In our study of 15 cfDNA samples, three had average Y-SNP reads below 30×, indicating the presence of female fetuses. The remaining 12 samples had average Y-SNP reads above 30×, suggesting male fetuses. Figure 5 presents the Y-SNP detection results for the groups of male fetuses, female fetuses, and the non-pregnant control group.
Figure 5. Comparison of Y-SNP reads in maternal plasma across different groups: the non-pregnant control group (red points), the female fetal group (green points), and the male fetal group (blue points). Each data point corresponds to a single Y-SNP read. The average Y-SNP reads for each group are depicted as a red line. The predefined threshold of 30× is represented by a dotted line. There was a statistically significant difference (P < 0.001) in Y-SNP reads of the male fetal group and the other groups, as indicated by *** and determined by an independent-sample t-test.
The average Y-SNP reads in plasma from the three pregnant women carrying female fetuses were
DISCUSSION
Short tandem repeats (STRs) are the predominant genetic markers used in forensic individual identification and paternity testing. However, the short length of degraded DNA fragments often leads to allele or locus dropout, and differentiating between minor contributors and STR stutters in mixtures is challenging. These limitations greatly restrict the use of STRs in NIPPT, which relies on cfDNA as the primary sample. SNP, another commonly used genetic marker, is more appropriate for cfDNA. Although SNPs are typically biallelic and exhibit less genetic polymorphism than STRs, they can effectively analyze highly fragmented and unbalanced mixtures. Previous studies have demonstrated that 50 SNPs can differentiate unrelated individuals as effectively as 12 STRs. However, the low concentration of target alleles means that their reads can easily be confused with background noise, necessitating the analysis of additional SNPs to improve identification accuracy.
In this study, we selected identification-informative SNPs (IISNPs) spanning all chromosomes with high heterozygosity and stable mutation rates, reducing the number of SNPs from thousands to hundreds. SNPs located on the X and Y chromosomes are often excluded in previous research to simplify the analysis, as their unique analytical methods differ from those used for autosomes. Despite their small number, these SNPs enhance the overall system efficacy and can serve as supplementary markers, providing valuable information for NIPPT. Y-SNPs, in particular, can infer the ethnicity and geographical origin of the samples due to their paternal inheritance characteristics, geographical variations, and ethnic specificity.
This study successfully determined the paternity of 15 family cases using the constructed SNP multiplex system, with results validated with PCR-CE. In these cases, the CPI values exceeded 1.05 × 1014, significantly surpassing the criteria for kinship determination (CPI > 10,000), demonstrating this method’s reliability. The specificity of the method was further confirmed by testing 70 unrelated males as alleged fathers in each of the 15 cases.
Effective SNPs were identified by distinguishing paternal alleles in cfDNA from maternal plasma and calculating the CPI to determine paternity. Setting appropriate sequencing thresholds for data interpretation is crucial for identifying as many paternal alleles as possible and achieving accurate fetal genotyping. A high threshold can prevent sequencing errors but may also exclude effective SNPs, while a low threshold can yield many effective SNPs but misclassify sequencing noise as paternal alleles. We set the sequencing threshold at 2% by analyzing the proportion of NGASRs in homozygous SNPs from six non-pregnant samples and 15 cfDNA from pregnant women. Additionally, ensuring a sequencing depth greater than 30× minimizes errors and maximizes the detection of effective SNPs.
In cases 6, 10, and 12, the number of SNPs decreased as the NGASR ratio declined. This discrepancy may be due to the lower proportion of fetal components in cfDNA from cases 6, 10, and 12. Maternal components influence these samples more significantly, resulting in numerous SNP identification failures. Low fetal fraction (FF) in cfDNA can lead to false-negative results and affect the detection rate of effective SNPs[29]. The results from mixtures with different ratios of minor components demonstrate that when the FF is less than 4%, maternal DNA significantly inhibits fetal components, and the number of effective SNPs increases as the fetal percentage grows. When the FF exceeds 4%, the maternal influence diminishes, allowing for accurate genotyping of paternal-derived alleles. Thus, using cfDNA with an FF exceeding 4% is recommended in practical applications. In practical applications, it has been observed that NIPPT during the early stage of pregnancy is prone to failure[8]. This is attributable to the fact that during the early pregnancy period, the proportion of cffDNA in the total DNA within the maternal plasma is extremely small. This results in a lack of effective amounts available for accurate detection[4]. Meanwhile, the abundant presence of maternal DNA significantly interferes with the detection of cffDNA, making it arduous to accurately distinguish between maternal DNA and fetal DNA. To ensure the accuracy of the test results, several measures can be taken. Firstly, the gestational week for blood collection can be appropriately adjusted, and advanced blood collection equipment, along with highly efficient nucleic acid enrichment techniques, can be employed. Secondly, the detection techniques can be enhanced by applying methods with higher sensitivity. Thirdly, the data analysis algorithms should be improved. Specifically, targeted algorithms need to be developed and supplemented with the assistance of machine learning and other means for auxiliary analysis. Meanwhile, the data quality control should be strengthened.
Identifying factors influencing FF can help improve prenatal paternity testing procedures and determine the most suitable detection timeframe. FF in maternal plasma may be influenced by gestational week and maternal age[30]. Our study showed no significant correlation between maternal age and FF, although the FF was higher in women under 25 compared to those over 25. However, the sample size was small, and the findings should be considered preliminary. Previous studies suggest that the proportion of fetal components in maternal cfDNA increases with gestational week, reaching its peak before delivery[31]. Our results corroborate these findings, showing significant differences in FF across different gestational ages and a positive correlation between FF and gestational weeks.
In NIPPT, safeguarding the rights of both embryos and parents is essential, especially in countries where abortion is permitted for non-medical reasons[32,33]. Early miscarriages have a lower impact on women’s health, and pregnancies are typically terminated at eight weeks or earlier. In our study, paternity was accurately determined as early as five weeks after conception, aligning with the initial emergence of cffDNA in maternal blood. Forensic applications encountering low fetal component samples should confirm results through repeated experiments or increase gestational age before testing to reduce failure risks. Moreover, data obtained from women who have previously given birth but are not currently pregnant support the finding that past pregnancies do not impact the genetic makeup of DNA in female plasma. In this study, we tested the plasma of women who had given birth in the past but were not currently pregnant and did not detect cell-free fetal DNA. This aligns with studies showing that cffDNA is rapidly cleared postpartum[4]. In women who have experienced miscarriage, the cffDNA in plasma also gets rapidly cleared after the termination of pregnancy. If the gestational age is relatively advanced, paternity testing can be conducted by examining fetal tissues. In the case of termination during the early stage of pregnancy, an attempt can be made to collect vaginal secretions. We will further validate the feasibility of this approach in our future research endeavors.
To demonstrate the advantages of the method in this study, we have summarized the studies on NIPPT in recent years in Supplementary Table 4. Upon comparison with the research conducted by Chang et al., it was observed that the number of SNPs involved in our new system is relatively small, which directly reduces sequencing costs and simplifies the data processing procedure[15]. While Tam et al. also employed a relatively small number of SNPs and demonstrated remarkable performance in terms of detection accuracy, their experimental procedure is notably complex[34]. Specifically, it is necessary to attach the Unique Molecular Identifier (UMI) sequences one by one to each DNA molecule to be sequenced in the early stage of sample processing. Moreover, specific probes need to be utilized to conduct the enrichment operation on the target genomic regions. In contrast, the method adopted in this study merely requires the extraction of cffDNA from the plasma of pregnant women following conventional procedures. Consequently, the operational process has been significantly simplified, making it more conducive to being promoted in practical applications. It is worth noting that the new system of this study also demonstrates an extremely excellent performance in terms of sensitivity. When the proportion of fetal components in the plasma of pregnant women reaches 2% or above, accurate genotyping can be achieved. This undoubtedly indicates that we are capable of successfully detecting fetal DNA at an even earlier stage of pregnancy, thereby providing more favorable conditions for the relevant research and applications.
Our research holds substantial potential for application in forensic practice. In criminal cases, specifically those involving sexual assaults, the determination of the paternity relationship can be achieved by analyzing the fetal DNA present in the peripheral blood of pregnant women and subsequently conducting a comparative analysis with the genes of suspected offenders. This process furnishes crucial evidence for the investigation, prosecution, and sentencing of such cases. In cases of trafficking in children where pregnant women are among the victims, this approach can also be utilized to elucidate the interpersonal relationships. In civil cases pertaining to the distribution of property involving a fetus, our novel methodology can be employed to precisely define the paternity relationship between the fetus and its relatives. It ensures the fairness and rationality of property distribution and contributes to the avoidance of subsequent disputes. In disputes regarding child support, the paternity of the fetus is customarily subject to query by the male party. It is capable of ascertaining whether the male should assume the obligation of providing child support, thereby safeguarding the legitimate rights and interests of pregnant women and the fetus.
Our study aims to standardize NIPPT in forensic practice, but it has limitations. The study involved a limited number of pregnant women, and future research should include more samples from early pregnancies with lower FF. Incomplete sample information prevented us from assessing the impact of maternal BMI and health status on FF. Additionally, the MAF of selected SNPs was validated only in a cohort of 100 unrelated Chinese individuals, and their applicability to other ethnic groups needs verification. Future large-scale studies with different gestational weeks are necessary to evaluate the accuracy and feasibility of this method in practical applications. In addition, this research did not analyze the impact of closely related males within the same patrilineal line on the accuracy of paternity testing. In practical applications, researchers are required to further determine the genetic relationship between the suspected biological father’s brothers or other male relatives and the fetus. We will include the male relatives of the biological father of the fetus as the research samples in our subsequent studies, with the aim of further refining this method.
CONCLUSION
In summary, we have developed a NIPPT method based on NGS technology. The multiplex detection system constructed in this study includes 627 SNPs and maintains over 99% effectiveness in detecting SNPs when the minor contributor in mixtures is as low as 4%. Our research demonstrated that cfDNA from pregnant women as early as five weeks can accurately determine kinship. The FF is influenced by gestational age and other factors, resulting in significant individual differences. Therefore, detection failures can still occur even at five weeks of gestation. In forensic practice, it may be necessary to extend the gestational age, use more IISNPs, or enrich cffDNA based on actual circumstances. Despite its limitations, our study has shown the practicality of this NIPPT method and its potential utility as an auxiliary tool for detecting trace DNA and analyzing mixtures. Future research will involve more pregnant women at different gestational stages to gather more detailed information on factors affecting testing efficiency. We aim to establish more effective analysis methods and enhance the accuracy and reliability of NIPPT in forensic applications.
DECLARATIONS
Acknowledgments
The authors thank all those who have contributed to the successful completion of this project. The appreciation extends to the participants of this study for their willingness to contribute to the advancement of knowledge in forensic science.
Authors’ contributions
Wrote and revised the manuscript: Qu Y, Zhang R, Zhang S
Conceptual design: Zhang S, Li C, Liang W
Performed experiments: Chen A, Qu Y, Qing L, Ma X
Performed analyses and quality control: Qu Y, Qing L, Ma X, Wang H
All authors reviewed and approved the manuscript.
Availability of data and materials
All data supporting the findings are available within the manuscript and the Supplementary Materials.
Financial support and sponsorship
This work was supported by a grant from the National Natural Science Foundation of China (82072123).
Conflicts of interest
Zhang S is a Junior Editorial Board member of Journal of Translational Genetics and Genomics. She was not involved in any steps of editorial processing, specifically reviewers’ selection, manuscript handling, and decision making. while the other authors declared that there are no conflicts of interest.
Ethical approval and consent to participate
This study was approved by the Ethics Committee of the Academy of Forensic Sciences, China
Consent for publication
Written informed consent for publication was obtained from all participants.
Copyright
© The Author(s) 2024.
Supplementary Materials
REFERENCES
1. Van den Veyver IB, Beaudet AL. Comparative genomic hybridization and prenatal diagnosis. Curr Opin Obstet Gynecol 2006;18:185-91.
3. Lo YMD, Corbetta N, Chamberlain PF, et al. Presence of fetal DNA in maternal plasma and serum. Lancet 1997;350:485-7.
5. Alberry M, Maddocks D, Jones M, et al. Free fetal DNA in maternal plasma in anembryonic pregnancies: confirmation that the origin is the trophoblast. Prenat Diagn 2007;27:415-8.
6. Rong Y, Gao J, Jiang X, Zheng F. Multiplex PCR for 17 Y-chromosome specific short tandem repeats (STR) to enhance the reliability of fetal sex determination in maternal plasma. Int J Mol Sci 2012;13:5972-81.
7. Zhang S, Han S, Zhang M, Wang Y. Non-invasive prenatal paternity testing using cell-free fetal DNA from maternal plasma: DNA isolation and genetic marker studies. Leg Med 2018;32:98-103.
8. Christiansen SL, Jakobsen B, Børsting C, et al. Non-invasive prenatal paternity testing using a standard forensic genetic massively parallel sequencing assay for amplification of human identification SNPs. Int J Legal Med 2019;133:1361-8.
9. Hu J, Yan K, Jin P, Yang Y, Sun Y, Dong M. Prenatal diagnosis of trisomy 8 mosaicism, initially identified by cffDNA screening. Mol Cytogenet 2022;15:39.
10. Juvet LK, Ormstad SS, Stoinska-Schneider A, et al. Non-invasive prenatal test (NIPT) for identification of trisomy 21, 18 and 13: report from the Norwegian Institute of Public Health No. 2016-18. 2016.
11. Butler JM. Genetics and genomics of core short tandem repeat loci used in human identity testing. J Forensic Sci 2006;51:253-65.
12. Wagner J, Dzijan S, Marjanović D, Lauc G. Non-invasive prenatal paternity testing from maternal blood. Int J Legal Med 2009;123:75-9.
13. Zhang R, Tan Y, Wang L, et al. Set of 15 SNP-SNP markers for detection of unbalanced degraded DNA mixtures and noninvasive prenatal paternity testing. Front Genet 2021;12:800598.
14. Shen X, Li R, Li H, et al. Noninvasive prenatal paternity testing with a combination of well-established SNP and STR markers using massively parallel sequencing. Genes 2021;12:454.
15. Chang L, Yu H, Miao X, Zhang J, Li S. Development and comprehensive evaluation of a noninvasive prenatal paternity testing method through a scaled trial. Forensic Sci Int Genet 2019;43:102158.
16. Gill P. An assessment of the utility of single nucleotide polymorphisms (SNPs) for forensic purposes. Int J Legal Med 2001;114:204-10.
17. Moriot A, Hall D. Analysis of fetal DNA in maternal plasma with markers designed for forensic DNA mixture resolution. Genet Med 2019;21:613-21.
18. Ou X, Qu N. Noninvasive prenatal paternity testing by target sequencing microhaps. Forensic Sci Int Genet 2020;48:102338.
19. Giannico R, Forlani L, Andrioletti V, et al. NIPAT as non-invasive prenatal paternity testing using a panel of 861 SNVs. Genes 2023;14:312.
20. Chen P, Deng C, Li Z, et al. A microhaplotypes panel for massively parallel sequencing analysis of DNA mixtures. Forensic Sci Int Genet 2019;40:140-9.
21. Chiu RWK, Poon LLM, Lau TK, Leung TN, Wong EMC, Lo YMD. Effects of blood-processing protocols on fetal and total DNA quantification in maternal plasma. Clin Chem 2001;47:1607-13.
22. Zheng H, Tao R, Zhang J, et al. Development and validation of a novel SiFaSTRTM 23-plex system. Electrophoresis 2019;40:2644-54.
23. McKenna A, Hanna M, Banks E, et al. The genome analysis Toolkit: a MapReduce framework for analyzing next-generation DNA sequencing data. Genome Res 2010;20:1297-303.
24. Li H, Durbin R. Fast and accurate short read alignment with Burrows-Wheeler transform. Bioinformatics 2009;25:1754-60.
25. Li H, Handsaker B, Wysoker A, et al. The sequence alignment/map format and SAMtools. Bioinformatics 2009;25:2078-9.
26. Baur MP, Elston RC, Gürtler H, et al. No fallacies in the formulation of the paternity index. Am J Hum Genet 1986;39:528-36.
27. Lo YMD, Patel P, Baigent CN, et al. Prenatal sex determination from maternal peripheral blood using the polymerase chain reaction. Hum Genet 1993;90:483-8.
28. Butler JM. Recent developments in Y-short tandem repeat and Y-single nucleotide polymorphism analysis. Forensic Sci Rev 2003;15:91-111.
29. Xu H, Wang S, Ma LL, et al. Informative priors on fetal fraction increase power of the noninvasive prenatal screen. Genet Med 2018;20:817-24.
30. Zaki-Dizaji M, Shafiee A, Kohandel Gargari O, Fathi H, Heidary Z. Maternal and fetal factors affecting cell-free fetal DNA (cffDNA) fraction: a systematic review. J Reprod Infertil 2023;24:219-31.
31. Birch L, English CA, O'Donoghue K, Barigye O, Fisk NM, Keer JT. Accurate and robust quantification of circulating fetal and total DNA in maternal plasma from 5 to 41 weeks of gestation. Clin Chem 2005;51:312-20.
32. Moray N, Pink KE, Borry P, Larmuseau MHD. Paternity testing under the cloak of recreational genetics. Eur J Hum Genet 2017;25:768-70.
33. Toya W. Ethical, legal and social issues in Japan on the determination of blood relationship via DNA testing. Asian Bioeth Rev 2017;9:19-32.
Cite This Article

How to Cite
Qu, Y.; Zhang, R.; Qing, L.; Ma, X.; Chen, A.; Liang, W.; Wang, H.; Li, C.; Zhang, S. A novel SNP-based approach for non-invasive prenatal paternity testing using multiplex PCR targeted capture sequencing. J. Transl. Genet. Genom. 2024, 8, 378-93. http://dx.doi.org/10.20517/jtgg.2024.46
Download Citation
Export Citation File:
Type of Import
Tips on Downloading Citation
Citation Manager File Format
Type of Import
Direct Import: When the Direct Import option is selected (the default state), a dialogue box will give you the option to Save or Open the downloaded citation data. Choosing Open will either launch your citation manager or give you a choice of applications with which to use the metadata. The Save option saves the file locally for later use.
Indirect Import: When the Indirect Import option is selected, the metadata is displayed and may be copied and pasted as needed.
About This Article
Copyright
Data & Comments
Data
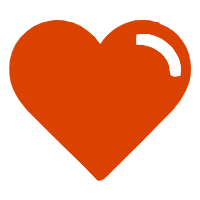
Comments
Comments must be written in English. Spam, offensive content, impersonation, and private information will not be permitted. If any comment is reported and identified as inappropriate content by OAE staff, the comment will be removed without notice. If you have any queries or need any help, please contact us at support@oaepublish.com.