Machine learning enhanced characterization and optimization of photonic cured MAPbI3 for efficient perovskite solar cells
Abstract
Photonic curing (PC) can facilitate high-speed perovskite solar cell (PSC) manufacturing because it uses
Keywords
INTRODUCTION
In recent years, the efficiency of perovskite solar cells (PSCs) has improved remarkably, with power conversion efficiencies (PCEs) reaching up to 26.7%[1]. Of the primary benefits for perovskites are the low cost and outstanding optoelectronic properties of solution-processed films, making PSCs an attractive addition to next-generation photovoltaic technologies[2]. Perovskite active layers often require a thermal annealing (TA) step to convert a deposited precursor film into a fully crystalline film that has high absorbance and large grains, and thus long diffusion length and high mobility[3,4]. However, this process typically takes tens of minutes of annealing at 100 to 150 °C and is a bottleneck for large-scale PSC manufacturing[5]. Previous works on photonic curing (PC), utilizing a xenon flash lamp to deliver intense broadband light to the film, reduced the annealing time of perovskite films to ~20 ms[6-8]. Therefore, this technique can be a candidate to replace TA in the scale-up manufacturing of PSCs.
All previous works on using PC for crystallizing perovskite films vary only by the amount of time over which light illuminates the sample, the pulse length (ms), and the energy the light delivers to the sample in one pulse, the radiant energy (J/cm2)[6,7,9-12]. In this work, we use a more sophisticated pulse that includes micro-pulses (µpulse), which split a single pulse into several smaller sub-pulses with a specified duty cycle. Using these additional features allows us to shape the temperature profile of the thin film, ultimately gaining more control over how the film crystallizes. The addition of these two variables requires optimization of a four-dimensional input space. In the case of problems with only two variables, a typical varying
Various studies have applied machine learning (ML) modeling along with high-throughput material, optical, and electronic characteristics to optimize perovskite materials[13-15], e.g., finding optimal triple-cation perovskite composition using photoluminescence[16] and employing machine vision and optical imaging of perovskite films to predict film quality and estimate short-circuit current density[17]. In this work, we perform BO to optimize PC conditions to crystallize MAPbI3 by measuring their ultraviolet-visible (UV-vis) absorbance spectra, which are used as a proxy for good PSCs. We quantitatively compare the UV-vis absorbance around the bandgap (600-850 nm) for TA and PC MAPbI3 using mathematical similarity metrics. UV-vis absorbance is chosen because it is a fast material characterization method, in addition to providing crucial information about MAPbI3 thin film properties. Beyond light absorption, shifts and changes in the shape of the absorbance curve can indicate grain size and uniformity (including the presence of pinholes, defects, and intermediaries)[18], film thickness[19], and crystallinity[20], which all play key roles in determining the PCEs of MAPbI3 PSCs.
Furthermore, we employ a ML method to improve grain size determination. The usual ASTM E112-13 line intercept method of grain size determination[21] is time-consuming and based on a limited amount of data from the image. By implementing an AI image segmentation model, we use data from the entire image and quickly return average crystal grain sizes that agree with the results for the standard method.
Finally, we fabricate p-i-n PSCs using the optimized PC MAPbI3 condition with a NiOx hole transport layer (HTL) at the bottom. Unexpectedly, our optimized PC PSCs exhibit significantly lower PCEs than TA devices despite their similar UV-vis absorbance. Based on the film temperature simulation using Simpulse®, we hypothesize that this is caused by an elevated MAPbI3 film temperature during PC, resulting in previously known interfacial reaction between NiOx HTL and the MAPbI3 active layer[22,23]. We find that the use of a buffer layer on top of the NiOx alleviates this reaction, significantly improving the PCE for PSCs made with PC MAPbI3.
MATERIALS AND METHODS
Materials
Patterned and unpatterned indium tin oxide (ITO) substrates (10 ohm/sq) were purchased from Kintec. Lead iodide (PbI2) was purchased from TCI America and methylammonium iodide (MAI) was purchased from GreatCell Solar. All other chemicals were purchased from Sigma-Aldrich or Fisher. Chemicals were used as received unless otherwise specified.
Perovskite film preparation for training dataset
Samples for the training dataset were prepared on unpatterned ITO substrates. The MAPbI3 precursor was prepared using established procedures[24,25]. Briefly, equal molar PbI2 and MAI were dissolved in
PSC fabrication
We used patterned ITO substrates to make p-i-n PSCs. The substrates were cleaned sequentially with soapy water, deionized (DI) water, acetone, and isopropanol, followed by a 20-min UV-ozone treatment. The NiOx precursor was prepared according to the following instructions. First, 0.1 M nickel nitrate hexahydrate and acetylacetone in 2-MOE were stirred overnight, and the solution was filtered through a 0.2 µm polytetrafluoroethylene (PTFE) filter immediately before usage. 60 µL of NiO precursor was spin-coated at 3,000 rpm for 30 s onto each sample followed by drying at 60 °C for 3 min. The temperature was increased to 150 °C and held for 5 min before increasing to 250 °C for calcination for a further 30 min. The hot plate was then turned off and the samples were allowed to cool for 20 min.
The samples were then either transferred to a glovebox for MAPbI3 precursor deposition or had a PbI2 or a [2-(3,6-Dimethoxy-9H-carbazol-9-yl)ethyl]phosphonic Acid (MeO-2PACz) buffer layer added. A PbI2 buffer layer was applied by spin coating 0.1 M PbI2 in dimethylformamide (DMF) at 3,000 rpm for 50 s in an N2-purged glovebox before annealing at 100 °C for 15 min[26]. The samples were then taken out of the glovebox and rinsed with an additional 1 mL of DMF to remove unbound residual PbI2 before being dried and returned to the glovebox. A MeO-2PACz buffer layer was made by spin coating a 0.5 mg/mL solution of MeO-2PACz in ethanol at 3,000 rpm for 30 s in ambient air before transferring to an N2 glovebox for TA at 100 °C for 10 min[27].
All samples were then spin-coated with the MAPbI3 precursor as described in the previous sub-section. Subsequently, the electron transport layer (ETL) was deposited by spin coating 20 mg/mL
Materials characterization
Device current density - voltage (J-V) measurements were taken using a 2635A Keithly source meter under an AM 1.5G 100 mW/cm2 illumination from an AAA solar simulator (Abet). Using a 0.0491 cm2 aperture, device forward scans were measured using a voltage sweep of -0.2 to 1.2 V with reverse scans sweeping from 1.2 to -0.2 V at 70 mV/s. The following material characterization techniques were taken on TA/PC MAPbI3 film samples on unpatterned ITO substrates. X-ray diffraction (XRD) patterns for each condition were measured using a Rigaku Mini Flex diffractometer at a scan speed of (3°/min) with Cu Kα radiation (λ = 1.518 Å). Scanning electron microscope (SEM) images of MAPbI3 films were taken using a Zeiss Supra 40 SEM at an acceleration voltage of 5 kV in a 7:3 InlenseDuo:SE2 mode. Atomic force microscopy (AFM) was performed on at least three 5 × 5 μm2 areas using an Asylum Research MFP-3D system. MAPbI3 film thickness was measured using a Keyence optical profilometer (VK-X3100) in a laser confocal mode. Absorbance data for all TA/PC MAPbI3 films was measured using an Ocean Optics USB 4000 spectrometer.
PC on MAPbI3 and temperature simulation
PC of MAPbI3 thin films was performed using a 500 V / 3 A PulseForge Invent system with a single lamp driver. Prior to pulsing a sample, the radiant energy for each PC condition was verified using a National Institute of Standards and Technology (NIST)-traceable bolometer. Samples were pulsed with the appropriate PC condition within 30 s after spin-coating. The pulsing procedure would involve securing the sample face-up onto the PulseForge Invent platform with two magnetic strips. Successful observation of crystallization is indicated by a color change from light brown to dark brown with a shiny appearance, similar to fully converted TA MAPbI3 films, immediately after PC.
Simulations of temperature vs. time for all PC samples were made using the built-in software SimPulse®. Simulated temperatures for each condition were taken on a material stack consisting of (from top down) MAPbI3 (270 nm), ITO (155 nm), and soda-lime glass (1.1 mm). MAPbI3 film thickness was nominally the same for all TA/PC annealing conditions [Table 1]. ITO and glass thickness were verified via specifications provided by the manufacturer. The thermal and optical properties for temperature simulations of ITO and glass were built into the SimPulse® database. All simulated material properties are available in Supplementary Table 2.
A summary of material properties for MAPbI3 thin films produced by TA and the three PC conditions
Annealing condition | Fréchet distance similarity | Normalized (110) MAPbI3 peak intensity | σRMS (nm) | Average grain size (nm) | MAPbI3 film thickness (nm) | Peak interface temperature (°C) |
TA | 1.02E-02 | 1.0 | 14 ± 1 | 161 ± 61 | 270 ± 10 | 100 |
PC 03 (6.80 J/cm2) | 2.84E-02 | 0.67 | 22 ± 1 | 151 ± 52 | 270 ± 7 | 334 |
PC 25 (11.5 J/cm2) | 1.32E-02 | 0.85 | 12 ± 1 | 223 ± 84 | 263 ± 5 | 464 |
PC 04 (12.2 J/cm2) | 6.35E-02 | 0.94 | 15 ± 0 | 313 ± 121 | 280 ± 7 | 509 |
ML method
Initial sampling
Initial PC conditions were chosen using a quasi-random Latin Hypercube Sampling (LHS) for the four PC parameters on the PulseForge Invent tool in “µpulse” mode. The four input parameters (range, increment) correspond to the pulse length (10-50 ms, in steps of 0.1 ms), radiant energy (3.0-13.5 J/cm2, in steps of
Similarity metric calculations
Quantitative comparisons between samples made by PC and TA are evaluated using four similarity metrics: two versions of the Procrustes distance, Fréchet distance, and root mean square distance (RMSD). All similarity metrics were calculated using prebuilt or user-generated MATLAB functions. Procrustes distance seeks to measure the dissimilarity between two curves represented by the same number of points by performing a rotation, translation, and scaling factor to minimize the sum of squares distance[28]. Curves that only differ by rotation, translation, or scale factor will have a Procrustes distance of zero. Procrustes distance was calculated using the built-in MATLAB function “procrustes”[29]. To emphasize the shape of the absorbance curve due to translational shift, which reflects scattering or band gap change, we modified the MATLAB function “procrustes” to deactivate rotation and scaling. This is referred to as the modified Procrustes similarity metric. The discrete Fréchet distance was used to compare two curves with the same number of points by searching for the minimal “maximum” pairwise distance between the two curves[30]. The discrete Fréchet distance, as calculated for this study, is a function that returns the maximum Euclidean distance between two discretely defined curves with the same endpoints[31]. RMSD was used as the final metric to serve as a baseline by simply measuring the average magnitude of the difference between corresponding points and returning the results as a single number. While cosine similarity is a common method for comparing curves, it produced similar values for all curves and was not able to provide useful information. The GP model we developed was designed for maximization, and because our distance metrics sought to minimize, we inverted the values to properly train the model. To invert and scale each metric, we took the absolute value of its logarithm. Additional information about scaling is available in Supplementary Materials. All scripts and functions associated with this study will be available in the GitHub repository (See Data Availability).
Active learning based on BO-GP models
In the interest of not biasing ourselves with a single metric, we trained four models on all four metrics described above. The models were built in MATLAB using the “fitrgp” function with all the associated information about functions and model parameters available in Supplementary Materials. The model was trained using the Matern 5/2 kernel function with automatic relevance determination (ARD) enabled. ARD allowed for independent tuning of characteristic length scales and scale factors for each input dimension. While the GP model can update the kernel hyperparameters as it learns from the dataset[12,32], this method did not work well for our data. When hyperparameters were allowed to be automatically tuned, a severely underfit model resulted. Therefore, we fixed the kernel hyperparameters by analyzing the variation amplitude and spacing of data for the four input variables.
A detailed explanation of how the kernel hyperparameters were chosen for each input variable is available in the Supplementary Materials. Feature importance for the four independently tunable PC variables can be inferred by comparing their characteristic length scales. A smaller length scale indicates that the model is more sensitive to changes in that feature, meaning that small variations in the feature value lead to significant changes in the model’s output. Conversely, a larger length scale suggests that the feature varies more slowly and has a less pronounced impact on the model’s predictions. Supplementary Table 3 shows that the length scale hyperparameters of pulse length and radiant energy are ~2 to 3× shorter than those of the number of µpulses and duty cycle, indicating that pulse length and radiant energy are more important.
The noise variance for each GP model was calculated as the standard deviation for each similarity metric from the LHS PC condition of which most samples were produced. We consider this value to be the uncertainty in making reproducible PC MAPbI3 films. Noise variance values were held constant during active learning iterations as the measurement uncertainty was not expected to change with the addition of new samples to the dataset. The acquisition function for the models was the upper confidence bound (UCB). Following the literature[12,33,34], a UCB exploration hyperparameter of b = 1 was used to maintain a balance between exploration and exploitation when picking the next condition. Each similarity metric model would suggest a new condition to try; thus, in each BO iteration, we have a total of four new conditions, one from each GP model. The search for optimized PC conditions is declared successful when a PC condition produces similarity metric values comparable to the values for two TA MAPbI3 films (~10-10 Procrustes distances, < 2.0 × 10-2 Fréchet distance, and < 2.0 × 10-1 RMSD); i.e., the similarity is within the experimental uncertainty.
Grain size determination
In literature, the ASTM E112-13 line intercept method[21] is the standard for determining average grain size for crystalline samples. However, the ASTM method is a cumbersome process that often requires manually placing several random line segments onto an image and counting the number of grain boundaries that are crossed. The average for a single image can then be calculated after tabulating the total length of the line segments and the total number of boundaries crossed. The drawbacks of this method to analyze multiple images include its time-consuming and tedious nature, the use of only limited data, and possible bias from the researchers in choosing the lines. In this study, we propose an alternative approach whereby a set of images of the same size and magnification can be analyzed in minutes. Using an artificial intelligence (AI) segmentation model derived from Facebook’s open-source Segment Anything Model[35] in a Google Collaborate environment, we can generate masks that correspond to the location of crystalline grains within an SEM image. We can then extract the size of the grains and quickly display the information within our script. The results from the AI segmentation model are compared to those from the ASTM E112-13 method. Our AI segmentation method is much faster in processing multiple SEM images and uses all data in the image.
RESULTS AND DISCUSSION
UV-vis spectra
Supplementary Table 1 displays the input variables of the PC conditions and the four similarity metrics when compared to the TA sample made at the same time. All PC conditions mentioned in the rest of this study will be referenced as PC ## where “##” represents the number of the PC condition as labeled in Supplementary Table 1. The first 20 rows (PC 00 to PC 19) constitute the LHS conditions used as the training dataset for the initial GPR models. Of note from the LHS conditions was PC 07. Condition PC 07 (13.2 J/cm2) was a particularly high radiant energy pulse that ablated the MAPbI3 from the substrate upon exposure and suggested an upper limit to the allowable radiant energy delivered to the film.
The first round of GP-generated conditions consists of PC 20 to PC 23 in Supplementary Table 2 and corresponds to a single new condition picked according to each of the four GP models. The four new conditions did not add any significant information about the dataset with none of the conditions producing a film with a new champion distance among any of the metrics. The models were rerun with the addition of these new data points, and the second round of GP-generated conditions (PC 24 to PC 26) were picked as the next most promising optimization conditions for the models trained on the first three similarity metrics (excluding RMSD due to overfitting). Condition PC 25, suggested by the Fréchet distance model, showed a particularly strong similarity to the TA reference. It was the only condition to achieve Procrustes distance values of 10-10 and one of only two conditions to yield Fréchet and RMS distances < 2.0 × 10-2. UV-vis spectra of MAPbI3 made using PC 03 (6.8 J/cm2), PC 04 (12.2 J/cm2), and PC 25 (11.5 J/cm2) are shown in Figure 1. PC 03, PC 25, and PC 04 are chosen to represent PC MAPbI3 films produced with low, optimal, and high radiant energy conditions. PC 03, a lower-energy condition, shows a clear UV-vis shift, although its Fréchet distance is small [Table 1]. In contrast, PC 04, a higher-energy condition, may appear not too different from the TA reference at first glance but has a poor Fréchet distance.
Figure 1. UV-vis absorbance curves for TA reference and three PC ITO/MAPbI3 samples showing the discrepancy of the curves for different PC conditions. UV-vis: Ultraviolet-visible; PC: Photonic curing; ITO: Indium tin oxide; MAPbI3: Methylammonium lead iodide.
The low similarity metrics of PC 25 suggest that we have found an optimized PC condition that can produce MAPbI3 films with the same UV-vis absorption spectrum as the TA sample. To verify the conversion, we created a final round of conditions (PC 27 to PC 30) that were all picked from the model trained on Fréchet distances. None of the new four conditions produced similarity metrics better than PC 25, and we concluded that the model was properly trained. Figure 2A corresponds to the final heat maps of the expected maximum values of the inverted scaled Fréchet distance in each pair of input parameters of all available PC conditions with the color-coded points corresponding to each round of LHS and GP-generated PC conditions with the best condition, PC 25, marked with a “red star”. The calculation of inverted scaled values of the Fréchet distance is detailed in the Supplementary Materials. Figure 2A clearly shows that the model has converged to an “optimal area” within the input parameter space. This area generally corresponds to a condition with a medium pulse length (> 20 ms), relatively higher radiant energy
Figure 2. (A) Heat maps for the BO-GP model using inverted scaled Fréchet distance as the output metric projected on each pair of input variables; (B) The parity plot comparing the predicted inverted scaled Fréchet distances vs. the experimentally determined inverted scaled Fréchet distance for all PC conditions. BO-GP: Bayesian optimization coupled with Gaussian process regression; PC: Photonic curing.
Material properties for photonic cured MAPbI3
To evaluate the quality of the MAPbI3 produced via PC, PC MAPbI3 films for the three conditions PC 03, PC 25, and PC 04 representing films produced with low, optimal, and high radiant energies were examined. We first performed XRD on all MAPbI3 films and a precursor film [Supplementary Figure 3]. For all annealed samples, tetragonal MAPbI3 perovskite is the dominant crystalline feature with strong intensities displayed for the (110) and (220) major reflections at 14.2° and 28.4°, respectively. The TA sample and the two higher radiant energy PC samples show a small PbI2 (001) peak at ~12.7°. The lower radiant energy condition (PC 03) and the precursor film both share a small peak around 8.2°, consistent with an intermediate MAPbI3 phase for 2-MOE-based, NMP-assisted MAPbI3[24]. Previous works on PC of DMF/dimethyl sulfoxide (DMSO)-based MAPbI3 using radiant energy similar to PC 03 also contained residual DMSO adducts[11]. The peak intensity for the (110) MAPbI3 crystallographic reflection normalized to the TA sample served as the benchmark for the crystallinity of PC samples. Table 1 shows that the crystallinity of PC samples increases with increasing radiant energy.
We measured surface roughness (σRMS) using AFM images shown in Supplementary Figure 4 with the results available in Table 1. The roughness of the PC 25 MAPbI3 was 12 nm, the lowest of the three PC conditions. Condition PC 03 displays the highest surface roughness. We postulate that this is caused by poor crystal grain planarization due to the sample not being exposed to enough heat for long enough during crystallization. Other work has shown that with perovskite thin films, higher annealing temperatures lead to increased grain size, which in turn reduces the surface roughness[36]. The high radiant energy sample, PC 04, also shows a higher surface roughness compared to PC 25 due to a larger number of small PbI2 crystals on the surface of the film, as evident in the SEM images in Supplementary Figure 5. Rough MAPbI3 active layers have been attributed to reduced adhesion and worse coverage of subsequent ETLs/HTLs[37], and an increased number of surface defects[38].
The morphology of MAPbI3 films is compared in Supplementary Figure 5. The SEM images for two higher radiant energy conditions, PC 25 and PC 04 [Supplementary Figure 5C and D], show small light-colored crystals, most likely PbI2, decorating the MAPbI3 grain boundaries, consistent with XRD. Previous work on PC MAPbI3 films made from a DMF/DMSO-based recipe also reported PbI2 crystals when processed with high radiant energy[7]. The SEM images of TA MAPbI3 films in Supplementary Figure 5A show no obvious signs of PbI2 on the surface, even though XRD for TA MAPbI3 indicates its presence in the bulk, suggesting that most PbI2 could be located there. Another possible explanation for the observed discrepancy between XRD and SEM for the TA sample could be a larger beam spot of XRD, which measures a broader area, whereas SEM analyzes smaller regions of the sample. Furthermore, films produced with PC 25 and PC 04 where the PbI2 is present on the surface have a weaker PbI2 (001) reflection compared to the TA reference, suggesting that PbI2 segregation is kinetically limited, as previously reported[7]. The MAPbI3 film produced with condition PC 03 shows a rougher crystal morphology [Supplementary Figure 5B] consistent with the surface roughness results measured by AFM.
SEM grain size analysis using AI segmentation
In this section, we demonstrate the power of using AI segmentation to better quantify the grain size in an SEM image. Starting with an unedited SEM image [Figure 3A], we compare the conventional process using the standard ASTM E112-13 line intercept method [Figure 3B] and the new AI segmentation method [Figure 3C]. As previously mentioned, the manual ASTM method typically takes tens of minutes to hours to quantify the average grain size for multiple images. Using the AI segmentation method, we can quickly run each image through a script that generates masks for each grain in the image [Figure 3C]. The script then tabulates the size of each grain and outputs the average and standard deviation for each image and for the whole set of images. Our method of grain size determination is fast (~3 min for five images). Furthermore, we can compare the amount of data used between the two methods by dividing the sum of the lengths, in pixels, of the eight random lines used in the ASTM method in Figure 3B by the pixels of the entire image used in the AI segmentation method. A simple estimate shows that the AI segmentation method uses ~200 times more pixels in calculating the average grain size. This gives us confidence that the grain size and distribution we obtain using the AI segmentation method reflect the morphology shown in the images more truthfully.
Figure 3. (A) An unprocessed TA MAPbI3 SEM image; (B) depicts the ASTM E112-13 line method for grain size determination; (C) shows masks for each grain generated by the AI segmentation model in the image; (D) The average grain diameters calculated using the AI segmentation model for the four types of MAPbI3. The scale bar in (A)-(C) is 100 nm. TA: Thermal annealed; MAPbI3: Methylammonium lead iodide; SEM: Scanning electron microscope; AI: Artificial intelligence.
As shown by the red circles in Figure 3C, the current segmentation model has some issues including double counting masks and poor grain edge detection. We validated and confirmed the accuracy of the model by first comparing the average grain diameters from the ASTM 112-13 method and our AI segmentation method. For example, in Figure 3, the grain size for this TA MAPbI3 is 146 ± 6 nm from the ASTM E112-13 method and 152 ± 65 nm from the AI segmentation method, comparable within the standard deviation range. Note that the larger standard deviation for the AI segmentation method accurately reflects the size distribution when all grains in the image are used for analysis. Statistical values comparing the grain sizes calculated from multiple images using the ASTM E112-13 and AI segmentation methods for each annealing condition are available in Supplementary Table 4.
We also compared the model’s output with an “ideally” segmented SEM image, which was obtained via an additional manual process to remove all overlapping masks. By removing overlapping masks and recalculating the grain size, the average diameter only differs by ± 5% compared to the automatic AI segmentation method. Currently, our model works without any image pre-processing and minimal post-processing, which only removes extreme outliers such as small PbI2 crystals. Further improvement on the AI segmentation method for grain size determination with pre- and post-processing features is the subject of future work.
The grain size distributions determined using the AI segmentation method for samples made using different annealing conditions are shown in Figure 3D and detailed in Table 1. By setting a lower threshold for masks, most PbI2 crystals in PC 25 and PC 04 are filtered out and not confused with MAPbI3 grains. Consistent with the crystallinity data from XRD and the results of a previous study on PC MAPbI3[7], the average grain size for photonic cured samples increases as the radiant energy delivered to the sample increases. Generally, MAPbI3 with larger crystal grain sizes corresponds to better PSC device performance as larger crystal grains reduce the likelihood of recombination events and trap states at the grain boundary, resulting in improved charge carrier lifetime and mobility[39-42].
PSCs using optimally photonic cured MAPbI3
Next, we compare PSC performance for p-i-n type devices made using TA and PC MAPbI3 films. NiOx is chosen as the base HTL. All PC PSCs used the optimized PC condition PC 25. In addition to the annealing method, the MAPbI3/NiOx interface is chemically modified. PSC J-V characteristics for all devices are shown in Figure 4 and Supplementary Table 5. The champion device J-V curves are shown in Figure 5.
Figure 4. Box plots for the statistical analysis of J-V characteristics for all PSC devices produced with different combinations of HTLs and annealing conditions. All PSCs contain NiOx as the base-HTL. Plot labels contain the MAPbI3 annealing type, and any addition of HTL buffer layer. MeO-2PACz is abbreviated as SAM in this plot’s labels. PSC: Perovskite solar cell; HTLs: Hole transport layers;
Figure 5. Champion forward and reverse J-V scans for devices produced with different HTL combinations and different MAPbI3 annealing conditions. MeO-2PACz is abbreviated as SAM in this plot’s labels. HTL: Hole transport layer; MAPbI3: Methylammonium lead iodide; SAM: Self-assembled monolayer.
Using NiOx as the HTL, MAPbI3 PSCs made by TA show PCEs of 10.3% ± 1.0%, while devices made by PC 25 show significantly worse J-V characteristics with an average PCE of 1.19% ± 0.75%. The PC PSCs primarily suffer in short-circuit current density (Jsc) with a still significant reduction to both open-circuit voltage (Voc) and fill factor, as seen in Figure 4 between black and red. These results are very unexpected as the Fréchet distance calculated from the UV-vis absorption spectra taken on these devices [Supplementary Figure 6] is small, agreeing with the results of PC MAPbI3 on ITO shown in Figure 1 and Table 1. Thus, the reduced J-V characteristics are not caused by the PC MAPbI3 itself. Since our device structure is p-i-n, MAPbI3 is deposited on the HTL, which undergoes the same processing conditions as the MAPbI3. Consequently, there could be an unanticipated problem at the PC MAPbI3/NiOx interface.
Previous work showed that MAPbI3 and NiOx begin to react at temperatures as low as 120 °C with significant damage occurring as temperatures approach 180 °C under steady state heating[23]. The reaction causes the decomposition of MAPbI3, severely degrading overall PSC J-V characteristics, consistent with what we see in PSCs with PC MAPbI3 on NiOx HTL[22]. As displayed in Table 1, the peak interface temperature for condition PC 25 simulated by SimPulse® reaches 464 °C, well above the threshold for MAPbI3/NiOx interaction. SimPulse® simulated temperature vs. time curve for PC 25
Next, we investigated a more recently studied HTL/buffer layer, MeO-2PACz. Depositing MeO-2PACz on top of the NiOx HTL and processing MAPbI3 using PC 25 produce PSC devices with PCEs slightly better than the TA MAPbI3/NiOx reference [Figures 4 and 5, Supplementary Table 5]. Photonic cured PSCs with NiOx HTL + MeO-2PACz show a Jsc of 16.5 mA/cm2, closing in on the 17.2 mA/cm2 we report for the TA MAPbI3/NiOx in Supplementary Table 5. The Voc and fill factor for the PC 25 sample are boosted slightly compared to the TA device. To verify that PC 25 is the optimal PC condition for MAPbI3 processing, we compared PSCs fabricated on NiOx HTL + MeO-2PACz using PC 03, PC 25, and PC 04. The J-V parameters [Supplementary Figure 8] show that PSC fabricated on NiOx + MeO-2PACz using PC 25 performed better than those made using other PC conditions. The results for TA devices fabricated on NiOx HTL + MeO-2PACz are also included.
CONCLUSIONS
In this work, we demonstrate that UV-vis absorbance can function as an effective metric for training a
DECLARATIONS
Acknowledgments
We thank J. V. Le for developing the script for grain size analysis and M. Valdez for her help and expertise in scanning electron microscopy.
Authors’ contributions
Developed the project idea: Allen CR, Lee M, Hsu JWP
Performed most experimental work including ML modeling, UV-vis testing/measurements, grain size analysis, and PSC fabrication: Allen CR
Performed most advanced material characterization work including AFM, XRD, and SEM, and assisted with PSC fabrication: Bhandari B
Oversaw experimental work and ML modeling: Xu W, Lee M, Hsu JWP
Wrote the first draft of the manuscript: Allen CR, Xu W
Revised and finalized the manuscript: Allen CR, Bhandari B, Xu W, Lee M, Hsu JWP
Availability of data and materials
Data and codes for this paper are available on GitHub (https://github.com/UTD-Hsu-Lab/MAPI-JMI).
Financial support and sponsorship
This work is supported by the National Science Foundation CMMI-2109554. Bhandari B acknowledges the support of the U.S. Department of Energy’s Office of Energy Efficiency and Renewable Energy under the Solar Energy Technologies Office Award Number DE-EE0009518. Hsu JWP acknowledges the support of the Texas Instruments Distinguished Chair in Nanoelectronics.
Conflicts of interest
All authors declared that there are no conflicts of interest.
Ethical approval and consent to participate
Not applicable.
Consent for publication
Not applicable.
Copyright
© The Author(s) 2024.
Supplementary Materials
REFERENCES
1. NREL. Best research-cell efficiency chart. Available from: https://www.nrel.gov/pv/cell-efficiency.html. [Last accessed on 30 Dec 2024].
2. Zhang C, Park N. Materials and methods for cost-effective fabrication of perovskite photovoltaic devices. Commun Mater 2024;5:636.
3. Abbasi S, Wang X, Tipparak P, et al. Proper annealing process for a cost effective and superhydrophobic ambient-atmosphere fabricated perovskite solar cell. Mat Sci Semicon Proc 2023;155:107241.
4. Penpong K, Seriwatanachai C, Naikaew A, et al. Robust perovskite formation via vacuum thermal annealing for indoor perovskite solar cells. Sci Rep 2023;13:10933.
5. Huddy JE, Ye Y, Scheideler WJ. Eliminating the perovskite solar cell manufacturing bottleneck via high-speed flexography. Adv Mater Technol 2022;7:2101282.
6. Lavery BW, Kumari S, Konermann H, Draper GL, Spurgeon J, Druffel T. Intense pulsed light sintering of CH3NH3PbI3 solar cells. ACS Appl Mater Interfaces 2016;8:8419-26.
7. Xu W, Daunis TB, Piper RT, Hsu JW. Effects of photonic curing processing conditions on MAPbI3 film properties and solar cell performance. ACS Appl Energy Mater 2020;3:8636-45.
8. Ghahremani AH, Pishgar S, Bahadur J, Druffel T. Intense pulse light annealing of perovskite photovoltaics using gradient flashes. ACS Appl Energy Mater 2020;3:11641-54.
9. Serafini P, Boix PP, Barea EM, Edvinson T, Sánchez S, Mora-Seró I. Photonic processing of MAPbI3 films by flash annealing and rapid growth for high-performance perovskite solar cells. Solar RRL 2022;6:2200641.
10. Ankireddy K, Ghahremani AH, Martin B, Gupta G, Druffel T. Rapid thermal annealing of CH3NH3PbI3 perovskite thin films by intense pulsed light with aid of diiodomethane additive. J Mater Chem A 2018;6:9378-83.
11. Xu W, Bonner JC, Piper RT, Hsu JWP. Effects of residual DMSO adduct on photonically cured MAPbI3 solar cells. J Phys Chem C 2023;127:14933-9.
12. Xu W, Liu Z, Piper RT, Hsu JW. Bayesian optimization of photonic curing process for flexible perovskite photovoltaic devices. Sol Energ Mat Sol C 2023;249:112055.
13. Yılmaz B, Yıldırım R. Critical review of machine learning applications in perovskite solar research. Nano Energy 2021;80:105546.
14. Song Q, Bai Y, Chen Q. The spring of processing chemistry in perovskite solar cells-bayesian optimization. J Phys Chem Lett 2022;13:10741-50.
15. Srivastava M, Howard JM, Gong T, Rebello Sousa Dias M, Leite MS. Machine learning roadmap for perovskite photovoltaics. J Phys Chem Lett 2021;12:7866-77.
16. Higgins K, Valleti SM, Ziatdinov M, Kalinin SV, Ahmadi M. Chemical robotics enabled exploration of stability in multicomponent lead halide perovskites via machine learning. ACS Energy Lett 2020;5:3426-36.
17. Taherimakhsousi N, Fievez M, Macleod BP, et al. A machine vision tool for facilitating the optimization of large-area perovskite photovoltaics. npj Comput Mater 2021;7:657.
18. Kumar V, Pandey A, Vishvakarma A, Kumar A, Kumar L, Pal Singh B. Growth of MAPbI3 perovskite films on MWCNT-modified TiO2 thin films for solar cell applications. Inorg Chem Commun 2024;163:112360.
20. Qaid SMH, Ghaithan HM, Al-Asbahi BA, Aldwayyan AS. Solvent effects on the structural and optical properties of MAPbI3 perovskite thin film for photovoltaic active layer. Coatings 2022;12:549.
22. Dunlap-Shohl WA, Li T, Mitzi DB. Interfacial effects during rapid lamination within MAPbI3 thin films and solar cells. ACS Appl Energy Mater 2019;2:5083-93.
23. Thampy S, Zhang B, Hong K, Cho K, Hsu JWP. Altered stability and degradation pathway of CH3NH3PbI3 in contact with metal oxide. ACS Energy Lett 2020;5:1147-52.
24. Lee SH, Hong S, Kim HJ. Selection of a suitable solvent additive for 2-methoxyethanol-based antisolvent-free perovskite film fabrication. ACS Appl Mater Interfaces 2022;14:39132-40.
25. Bhandari B, Bonner JC, Piper RT, Hsu JWP. Effects of transparent conducting electrodes and hole transport layers on the performance of MAPbI3 solar cells fabricated on PET substrates. Flex Print Electron 2024;9:035002.
26. Mohanraj J, Samanta B, Almora O, et al. NiOx passivation in perovskite solar cells: from surface reactivity to device performance. ACS Appl Mater Interfaces 2024;16:42835-50.
27. Phung N, Verheijen M, Todinova A, et al. Enhanced self-assembled monolayer surface coverage by ALD NiO in p-i-n perovskite solar cells. ACS Appl Mater Interfaces 2022;14:2166-76.
29.
30. Eiter T, Mannila H. Computing discrete fréchet distance. 1994. Available from: https://www.researchgate.net/profile/Thomas-Eiter-2/publication/228723178_Computing_Discrete_Frechet_Distance/links/5714d93908aebda86c0d1a7b/Computing-Discrete-Frechet-Distance.pdf. [Last accessed on 30 Dec 2024].
31. Danziger Z. Discrete frechet distance. MATLAB Central File Exchange. 2024. Available from: https://www.mathworks.com/matlabcentral/fileexchange/31922-discrete-frechet-distance. [Last accessed on 30 Dec 2024].
32. Gongora AE, Xu B, Perry W, et al. A Bayesian experimental autonomous researcher for mechanical design. Sci Adv 2020;6:eaaz1708.
33. Rohr B, Stein HS, Guevarra D, et al. Benchmarking the acceleration of materials discovery by sequential learning. Chem Sci 2020;11:2696-706.
34. Liu Z, Rolston N, Flick AC, et al. Machine learning with knowledge constraints for process optimization of open-air perovskite solar cell manufacturing. Joule 2022;6:834-49.
35. Kirillov A, Mintun E, Ravi N, et al. Segment anything. arXiv 2023; arXiv:2304.02643. Available from:
36. Wu G, Cai M, Cao Y, et al. Enlarging grain sizes for efficient perovskite solar cells by methylamine chloride assisted recrystallization. J Energy Chem 2022;65:55-61.
37. Jin H, Farrar MD, Ball JM, et al. Alumina nanoparticle interfacial buffer layer for low-bandgap lead-tin perovskite solar cells. Adv Funct Mater 2023;33:2303012.
38. Cui P, Fu P, Wei D, et al. Reduced surface defects of organometallic perovskite by thermal annealing for highly efficient perovskite solar cells. RSC Adv 2015;5:75622-9.
39. Wang T, Lian G, Huang L, et al. MAPbI3 quasi-single-crystal films composed of large-sized grains with deep boundary fusion for sensitive vis-NIR photodetectors. ACS Appl Mater Interfaces 2020;12:38314-24.
40. Giesbrecht N, Schlipf J, Grill I, et al. Single-crystal-like optoelectronic-properties of MAPbI3 perovskite polycrystalline thin films. J Mater Chem A 2018;6:4822-8.
41. Kim HD, Ohkita H, Benten H, Ito S. Photovoltaic performance of perovskite solar cells with different grain sizes. Adv Mater 2016;28:917-22.
42. deQuilettes DW, Vorpahl SM, Stranks SD, et al. Solar cells. Impact of microstructure on local carrier lifetime in perovskite solar cells. Science 2015;348:683-6.
Cite This Article
How to Cite
Allen, C. R.; Bhandari, B.; Xu, W.; Lee, M.; Hsu, J. W. P. Machine learning enhanced characterization and optimization of photonic cured MAPbI3 for efficient perovskite solar cells. J. Mater. Inf. 2024, 4, 35. http://dx.doi.org/10.20517/jmi.2024.72
Download Citation
Export Citation File:
Type of Import
Tips on Downloading Citation
Citation Manager File Format
Type of Import
Direct Import: When the Direct Import option is selected (the default state), a dialogue box will give you the option to Save or Open the downloaded citation data. Choosing Open will either launch your citation manager or give you a choice of applications with which to use the metadata. The Save option saves the file locally for later use.
Indirect Import: When the Indirect Import option is selected, the metadata is displayed and may be copied and pasted as needed.
About This Article
Special Issue
Copyright
Data & Comments
Data
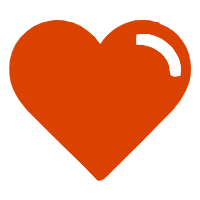
Comments
Comments must be written in English. Spam, offensive content, impersonation, and private information will not be permitted. If any comment is reported and identified as inappropriate content by OAE staff, the comment will be removed without notice. If you have any queries or need any help, please contact us at support@oaepublish.com.