N-heterocyclic carbene coordinated single atom catalysts on C2N for enhanced nitrogen reduction
Abstract
Single-atom catalysts (SACs) with N-heterocyclic carbene (NHC) coordination provide an effective strategy for enhancing nitrogen reduction reaction (NRR) performance by modulating the electronic properties of the metal active sites. In this work, we designed a novel NHC-coordinated SAC by embedding transition metals (TM) into a two-dimensional C2N-based nanomaterial (TM@C2N-NCM) and evaluated the NRR catalytic performance using a combination of density functional theory and machine learning. A multi-step screening identified eight
Keywords
INTRODUCTION
Ammonia (NH3) is indispensable in modern industry, primarily as an essential component in global fertilizer production and as a key precursor in the synthesis of numerous chemicals[1]. Moreover, NH3 has gained increased attention as a promising carbon-free energy carrier due to its high hydrogen content
Single-atom catalysts (SACs), characterized by atomically dispersed metal atoms on a substrate, have emerged as a promising class of catalysts due to their maximized metal atom utilization, tunable electronic properties, and exceptional catalytic performance[17-24]. SACs have shown great promise in various energy conversion reactions[25-27], and numerous high-performance SACs for NRR have been reported both experimentally and theoretically[28-35]. For instance, Feng et al. synthesized an efficient NRR electrocatalyst by immobilizing Ru atoms onto a graphdiyne/graphene sandwich structure, achieving a high NH3 yield rate of 56.8 μg h-1 mgcat-1 and a Faradaic efficiency (FE) of 37.6%[36]. Similarly, Geng et al. developed a
N-heterocyclic carbene nanomaterials (NCMs), which incorporate molecular N-heterocyclic carbenes (NHCs) into the lattices of low-dimensional carbon materials, represent a novel class of carbon-nitride materials with well-defined porous structures formed by NHC units[45-49]. These materials combine the advantages of NHCs and the rigid, periodic carbon frameworks, facilitating the formation of robust C-TM bonds with metal atoms. Simultaneously, the σ-donating and π-accepting properties of NHCs interact with the d-orbitals of the metal, modulating its electronic structure and thereby enhancing catalytic activity[47,49]. Thus, NCMs offer a distinctive NHC coordination environment, making them highly promising platforms for the design of high-performance SACs. In our previous work, graphene-based NCMs have been successfully employed to anchor various TMs (TM@graphene-NCM), demonstrating significant catalytic potential for NRR[45,47]. Among them, a Mn-embedded SAC exhibited remarkable performance, achieving a limiting potential (UL) as low as -0.51 V[47].
C2N-h2D (C2N), a two-dimensional porous carbon nitride material first synthesized in 2015, has garnered attention as an excellent substrate for anchoring metal atoms due to its wide band gap, high electron mobility, and excellent thermal stability[50,51]. Its unique structure, characterized by electron-rich nitrogen atoms exposed within a two-dimensional framework, offers an ideal platform for NHC functionalization, facilitating the design of novel NCM. Unlike graphene, C2N possesses distinct structural and electronic properties, providing a different local coordination environment that modulates the electronic properties of the anchored metal atoms, potentially enhancing their catalytic performance[24,52,53]. Inspired by these advantages, we systematically investigated C2N-based NCMs as platforms for SACs and explored their potential in NRR catalysis.
In this study, we designed 28 SACs by embedding 3d, 4d, and 5d TMs into a C2N-based NCM system, denoted as TM@C2N-NCM. Using a combination of first-principles calculations and machine learning (ML), we systematically evaluated the NRR catalytic performance of these SACs. Through a multiple-step screening process, eight candidates with high catalytic activity and selectivity for NRR were identified, all exhibiting lower UL than their TM@Graphene-NCM counterparts, demonstrating enhanced catalytic performance. Notably, Nb@C2N-NCM showed the best performance, with a UL of -0.29 V. ML models further revealed the intrinsic factors governing the varied NRR performance across different SACs. Compared to graphene-based NCMs, the introduction of C2N not only improved catalytic activity but also expanded the range of TMs that could be utilized as promising SACs for NRR. This study provides valuable insights into the design of highly efficient NRR electrocatalysts and offers strategies for developing more effective SACs in future research.
MATERIALS AND METHODS
All density functional theory (DFT) calculations were carried out using the Vienna Ab Initio Simulation Package (VASP)[54], with the electronic interactions between ions and electrons described through the projector augmented wave (PAW) method[55]. The exchange-correlation effects were treated using the generalized gradient approximation (GGA) combined with the Perdew-Burke-Ernzerhof (PBE) functionals[56]. For the plane-wave basis set, a kinetic energy cutoff of 500 eV was chosen to ensure computational accuracy. The energy convergence criterion was set at 10-5 eV, while the force convergence threshold was fixed at 0.01 eV/Å. The Brillouin zone was sampled using 2 × 2 × 1 and 8 × 8 × 1 Gamma-centered k-points for geometry optimizations and electronic property calculations[57]. Spin polarization was included in all calculations to account for potential magnetic effects. A vacuum layer of 18 Å along the
For the ML analysis, we utilized three non-linear regression algorithms: Random Forest Regression (RFR), Gradient Boosting Regression (GBR), and Extreme GBR (XGBR)[61-65]. A grid search technique was applied to optimize the hyperparameters for each model[66,67]. These algorithms were selected for their robustness against overfitting, their ability to process high-dimensional datasets, and their superior predictive accuracy in material property modeling. All ML models were implemented using the Scikit-learn library[68]. To prevent overfitting, data normalization was performed prior to training, and a 5-fold cross-validation was used to validate model performance. Model accuracy was evaluated using the coefficient of determination (R2) and mean absolute error (MAE), with ideal models approaching an R2 value of 1 and an MAE close to 0. For feature selection, we applied both Pearson correlation coefficient heatmap analysis and Recursive Feature Elimination (RFE)[69]. The heatmap identifies highly correlated features, enabling us to eliminate redundancy, while RFE iteratively removes the least important features, further refining the feature set. By combining these two methods, we derived the optimal feature subset for input into the ML models. To interpret the ML results and understand the influence of key descriptors on catalytic activity, the Shapley Additive Explanations (SHAP) method was employed. SHAP provides the magnitude and direction of the contribution of each feature to the model predictions[70]. In the SHAP summary plot, each point corresponds to a sample, with the horizontal axis showing the SHAP value, indicating the impact of the feature on the prediction. Positive SHAP values contribute to an increase in the predicted value, while negative values result in a decrease. The color of each point reflects the feature value, with red indicating higher values and blue representing lower values.
RESULTS AND DISCUSSION
Structure of TM@C2N-NCM
To construct the target catalysts, a C2N-based NCM was designed by introducing three classic five-membered NHC units into a 2 × 2 C2N supercell. TM atoms from the 3d, 4d, and 5d series were subsequently anchored into the pores of the C2N-based NCM framework, resulting in the TM@C2N-NCM structure (see Figure 1A). The lattice constant is a = b = 16.63 Å. The TMs studied include 3d (Sc, Ti, V, Cr, Mn, Fe, Co, Ni, Cu, Zn), 4d (Y, Zr, Nb, Mo, Ru, Rh, Pd, Ag, Cd), and 5d (Hf, Ta, W, Re, Os, Ir, Pt, Au, Hg) elements [Figure 1B]. To gain a more comprehensive understanding of the role of the substrate and various metals and identify broader trends, toxic Cd and Hg were also included in the study. Each TM atom is stably coordinated by three NHC units, forming robust TM-C bonds. Due to the presence of three five-membered rings, the non-planar TM@C2N-NCM structures result in the exposure of these metal centers on the surface, which is beneficial for N2 adsorption and catalytic activity.
Figure 1. (A) Top and side views of the optimized structure of TM@C2N-NCM. TM, C, and N atoms are represented in grey, yellow, and blue, respectively; (B) The 3d, 4d, and 5d transition metals selected for this study; (C) Calculated binding energies (Eb) of the TMs on the C2N-NCM substrate. TM@C2N-NCM:Transition metals into a two-dimensional C2N-based nanomaterial; TM: Transition metals.
To evaluate the stability of the metal atoms on the substrate, the binding energies (Eb) of the TMs with the C2N-NCM were calculated. As shown in Figure 1C, all TMs exhibited negative Eb, except for Au, which had a slightly positive value of 0.02 eV, indicating weak interaction between Au and the substrate. For the remaining 27 TMs, the Eb values range from -10.03 eV (Os) to -0.75 eV (Hg). These negative values confirm their stable adsorption on the C2N-NCM substrate, with more negative values indicating stronger metal-substrate interactions. Additionally, we found that the trend in Eb is strongly influenced by the d-electron configuration of the TMs, which is consistent with observations from TM@graphene-NCM. Metals located in the middle of each period (e.g., Fe, Ru, Os) exhibited more negative Eb values, which can be attributed to a balanced electron donation from the metal to the NHC ligands and back-donation from the NHCs to the d-orbitals of metal atoms. Early TMs (e.g., Ti, Zr, Ta) lack sufficient d-electrons for effective back-donation, while late TMs (e.g., Zn, Cd, Hg) have fewer vacant d-orbitals to accept electron donation from the NHCs. To further verify the thermodynamic stability of the catalysts, AIMD simulations were performed. Nb@C2N-NCM, which later demonstrated the best catalytic performance, was selected as a representative example to further verify its stability. The results showed that the energy fluctuations remained near equilibrium throughout the simulation, with the Nb atom firmly anchored in the NHC coordination environment, confirming the favorable thermodynamic stability of the structure (see Supplementary Figure 1). Based on these results, 27 SACs (excluding Au) were identified as stable candidates for further catalytic investigation.
Screening strategy
As shown in Figure 2, the NRR proceeds through several mechanistic pathways, including Distal, Alternating, Enzymatic, and Consecutive routes, each involving different proton and electron transfer sequences. Given this complexity, a systematic and efficient screening approach is essential for evaluating the catalytic performance of the designed catalysts. A three-step screening strategy was developed based on previous theoretical studies[38,71,72]. The first criterion is the Eads of N2, as effective N2 binding is crucial for initiating NRR. A threshold of Eads < -0.50 eV was established to ensure strong chemisorption. Although the pathways differ in intermediates, two protonation steps are critical across all mechanisms: the first protonation (*N2 + H+ + e- = *NNH), which breaks the N≡N triple bond, and the last protonation step (*NH2 + H+ + e- = *NH3), where nitrogen transitions from a half-filled sp3 hybrid orbital in *NH2 to a fully filled sp3 configuration in NH3. These two steps have been widely recognized in previous studies as the most likely potential-determining steps (PDS) in NRR, primarily due to the significant energy barriers associated with them[73-77]. Thus, the second and third criteria focus on the ΔG for these protonation steps, with
N2 adsorption and activation
The adsorption of N2 on TM@C2N-NCM occurs in two primary configurations, i.e., end-on and side-on. The corresponding Eads and N-N bond lengths for both configurations are summarized in Figure 3, with detailed values provided in Supplementary Table 1. Among the 27 investigated TMs, Zn, Ru, Cd, and Hg can only stabilize N2 in the end-on configuration, while the remaining metals can accommodate both adsorption modes. The Eads values for the end-on configuration range from -1.35 eV to -0.15 eV, while those for the side-on configuration range from -1.44 eV to -0.19 eV. More negative Eads values indicate stronger N2 binding, suggesting that the N2 molecule preferentially adopts the end-on configuration for most TMs. The N-N bond lengths after adsorption vary between 1.10 Å and 1.19 Å, indicative of varying degrees of N2 activation (except for the Zn system, where minimal activation is observed). The side-on mode typically results in greater N2 activation due to simultaneous interactions between both nitrogen atoms and the active metal center. To further elucidate the mechanism of N2 activation at the metal active sites, Fe@C2N-NCM and Nb@C2N-NCM, which have been identified as the most promising NRR catalysts in subsequent studies, were selected for detailed analysis using charge density difference, Bader charge, and projected density of states (PDOS) calculations. In both systems, the N2 molecules adopt the side-on configuration. As shown in Figure 4A and B, significant electron transfer occurs between the metal atoms and the adsorbed N2 molecules, resulting in charge accumulation at the TM-N interface and depletion along the N≡N bond. This electron redistribution is consistent with the classical “donation-acceptance” mechanism, where the empty d orbitals of metal atoms accept electron density from the lone pairs of N2, while simultaneously
Figure 3. Adsorption energies (Eads) and N-N bond lengths of N2 adsorbed on TM@C2N-NCM (TM = 3d, 4d, 5d transition metals) in end-on and side-on configurations. TM@C2N-NCM:Transition metals into a two-dimensional C2N-based nanomaterial.
Figure 4. (A) The Charge density difference for Fe@C2N-NCM and (B) Nb@C2N-NCM, with yellow and blue regions representing electron accumulation and depletion, respectively. The isosurface level is set at 0.0003 e/Å3; (C, D) PDOS for N2 adsorption on
Screening of the first and last protonation steps
After the initial screening based on N2 adsorption, the ΔG values for the first and last protonation steps were calculated for 37 N2 adsorption systems to refine the catalyst selection. As shown in Figure 5, eleven systems in the yellow-shaded region meet the second and third screening criteria (ΔG(*N2→*NNH) < 0.55 eV and
Figure 5. (A) Second and third step screening results of TM@C2N-NCM, based on the ΔG for *N2 → *NNH and *NH2 → *NH3. The yellow-shaded region in the lower-left contains the materials that passed both criteria; (B) Close-up view of the yellow-shaded region. TM@C2N-NCM: Transition metals into a two-dimensional C2N-based nanomaterial.
Full reaction pathways and selectivity
UL, which corresponds to the maximum free energy change (ΔGmax) in the PDS, is commonly used to evaluate the catalytic activity of NRR catalysts. To accurately determine UL, full-pathway calculations were performed for the eleven systems identified in the screening process. For catalysts with side-on adsorption (TM = Ti, V, Fe, Zr, Nb, Ta, W, Os, Ir), both enzymatic and consecutive pathways were analyzed. The distal and alternating pathways were evaluated for the Pt system due to the end-on adsorption, while Mn, capable of both adsorption modes, was assessed across all four pathways. The UL values and corresponding PDS for each system are illustrated in Figure 6A. The full reaction pathway for Nb@C2N-NCM, which demonstrated the best catalytic performance, is presented in Figure 6B, while the pathways for the remaining systems are provided in Supplementary Figure 2. Generally, the protonation steps across all pathways exhibit a characteristic increasing-decreasing energy trend. Seven systems exhibit the PDS in the first protonation step, with corresponding UL values of -0.46 V for V, -0.42 V for Mn, -0.30 V for Fe, -0.29 V for Nb, -0.47 V for Ta, -0.44 V for W, -0.53 V for Os, -0.51 V for Ir, and -0.44 V for Pt. Among these, Nb@C2N-NCM demonstrated the lowest UL, indicating the highest catalytic activity. In the cases of Zr@C2N-NCM and Ta@C2N-NCM, the PDS is located in the last step of the consecutive pathway, with UL values of -0.48 V and -0.47 V, respectively. For Ti@C2N-NCM, the PDS occurs during the third step of the enzymatic pathway (*NH*NH + H+ + e- → *NH2*NH), yielding a UL of -0.68 V. Except for Ti, all systems follow the
Figure 6. (A) Calculated theoretical UL and corresponding PDS for 11 TM@C2N-NCM catalysts. The color indicates the N2 adsorption configuration, and the grid pattern represents the protonation step in which the PDS occurs; (B) Calculated free energy diagrams for NRR via the enzymatic and consecutive mechanisms on Nb@C2N-NCM; (C) Comparison of adsorption energies (ΔE) for *N2 and *H. TM@C2N-NCM: Transition metals into a two-dimensional C2N-based nanomaterial; PDS: Potential-determining steps; NCM:
Although the computational hydrogen electrode (CHE) model can effectively describe the reaction mechanism and catalytic activity of NRR on Nb@C2N-NCM, it is overly simplistic for complex working conditions, considering the influence of electrode potential only through energy correction. Therefore, we further employed the Standard Hydrogen Electrode (SHE) model to investigate the effect of potential on the NRR activity of Nb@C2N-NCM. Supplementary Figure 3A shows the computed energies as a function of the applied electrode potential (vs. SHE) for Nb@C2N-NCM and the corresponding reaction intermediates. It demonstrates that the energy-potential points fit well into a quadratic function. As shown in Supplementary Figure 3B, we obtained the electrode potential-dependent free energy curves. The results indicate that Nb@C2N-NCM exhibits the best catalytic activity at an electrode potential of -3V, with the corresponding UL determined to be -0.14 V vs. SHE. Subsequently, we considered the effect of potential on the selectivity of the catalyst [Supplementary Figure 3C]. The results show that within the potential range considered, N2 always has lower Eads and more readily occupies the active sites of the catalyst compared to the H atom, indicating excellent selectivity for NRR over HER.
Machine learning analysis
To explore the intrinsic factors influencing the catalytic performance of NRR catalysts, ML was employed to uncover the relationships between fundamental physicochemical properties and catalytic activity. The workflow of the ML approach is shown in Figure 7. The target variable dataset was derived from the
To construct a reliable feature set, 20 features were selected (see Supplementary Table 2). Seventeen of these features represent the inherent properties of TM atoms, such as Pauling electronegativity (χP), the number of d-electrons (Nd), and electron affinity (EA), with values obtained from the National Institute of Standards and Technology (NIST) database[78].
Additionally, three binary features were introduced to distinguish between different protonation steps and adsorption modes: S1E (first-step protonation of side-on adsorption), S1S (first-step protonation of end-on adsorption), and S6 (last-step protonation). These features were encoded as one-hot vectors and incorporated into the feature set. We first analyzed the Pearson correlation among the 20 features to identify any potential redundancy. Subsequently, the RFE method was employed to select the optimal feature subset. The Pearson correlation heatmap is presented in Supplementary Figure 4, while the final dataset after feature selection is summarized in Supplementary Table 3. The results of optimal ML models using three algorithms (XGBR, GBR, RFR) are shown in Figure 8A-C. All models demonstrated strong linear correlations between the predicted values and the DFT-calculated results, with R2 values ranging from 0.88 to 0.91 and MAE values between 0.19 and 0.24, indicating excellent predictive performance across all models. Among these, XGBR exhibited the best performance, achieving the highest R2 and the lowest MAE. Additionally, the average R2 and MAE values from the 5-fold cross-validation are summarized in Figure 8D, further confirming XGBR as the best-performing model. Furthermore, we conducted additional model training using eight features for comparison. The results, as shown in Supplementary Figure 5, indicate that the average scores are very close, thereby supporting the reliability of the model.
Figure 8. (A-C) Comparison of DFT-calculated ΔG values with those predicted by three ML algorithms; (D) Average R2 and MAE scores from 5-fold cross-validation for three ML models. DFT: Density functional theory; ML: Machine learning; MAE: Mean absolute error.
Feature importance analysis revealed that the one-hot encoded features S6 contributed the most, accounting for 56.96% of the total importance. These features are crucial for distinguishing between the first and last protonation steps, and identifying the side-on and end-on adsorption modes in the first protonation step. The Mendeleev number (Nm) and Nd of the metal atom ranked as the second and third most important features, contributing 23.77% and 8.94%, respectively (see Figure 9A). This indicates that these two intrinsic properties of the metal center play a key role in determining the catalytic performance of the catalysts. To further understand the relationships between C-PDS and the key features, SHAP analysis was employed. As shown in Figure 9B, the most important feature S6 has a clear bipolar distribution, indicating that the last protonation step generally has a lower ΔG than the first step. Similarly, S1E, ranked fourth, reveals that
CONCLUSIONS
In this study, a combination of DFT calculations and ML methods was employed to comprehensively evaluate the NRR catalytic performance of 28 TM@C2N-NCM catalysts by embedding TMs into a
DECLARATIONS
Authors’ contributions
Made substantial contributions to the conception and design of this article, writing, and editing: Lu W, Xu J, Liu W
Assisted in processing the data of DFT calculations: Lu W, Zheng D
Provided technical support for machine learning and completed analysis and processing of data: Zheng D, Ye D
Performed data acquisition and interpretation: Lu W, Zheng D, Peng J, Gong X
Provided guidance and revised the manuscript: Xu J, Liu W
Availability of data and materials
The rata data supporting the findings of this study are available within this article and its Supplementary Materials. Further data are available from the corresponding authors upon request.
Financial support and sponsorship
This work is supported by the National Natural Science Foundation of China (No.12075211) and the Scientific Research Foundation of Zhejiang A&F University (No.2019FR005).
Conflicts of interest
All authors declared that there are no conflicts of interest.
Ethical approval and consent to participate
Not applicable.
Consent for publication
Not applicable.
Copyright
© The Author(s) 2024.
Supplementary Materials
REFERENCES
1. Canfield DE, Glazer AN, Falkowski PG. The evolution and future of Earth's nitrogen cycle. Science 2010;330:192-6.
2. Foster SL, Bakovic SIP, Duda RD, et al. Catalysts for nitrogen reduction to ammonia. Nat Catal 2018;1:490-500.
3. Rosca V, Duca M, de Groot MT, Koper MT. Nitrogen cycle electrocatalysis. Chem Rev 2009;109:2209-44.
4. Liu H. Ammonia synthesis catalyst 100 years: Practice, enlightenment and challenge. Chin J Catal 2014;35:1619-40.
5. Lehnert N, Musselman BW, Seefeldt LC. Grand challenges in the nitrogen cycle. Chem Soc Rev 2021;50:3640-6.
7. Kyriakou V, Garagounis I, Vourros A, Vasileiou E, Stoukides M. An electrochemical haber-bosch process. Joule 2020;4:142-58.
8. Chen JG, Crooks RM, Seefeldt LC, et al. Beyond fossil fuel-driven nitrogen transformations. Science 2018;360:eaar6611.
9. Yu L, Li F. Pt2 dimer anchored vertically in defective BN monolayer as an efficient catalyst for N2 reduction: a DFT Study. Catalysts 2022;12:1387.
10. Zhao X, Zhang X, Xue Z, Chen W, Zhou Z, Mu T. Fe nanodot-decorated MoS2 nanosheets on carbon cloth: an efficient and flexible electrode for ambient ammonia synthesis. J Mater Chem A 2019;7:27417-22.
11. Li S, Peng M, Song Y, et al. Screening transition metal and nonmetal atoms co-doped graphyne as efficient single-atom catalysts for nitrogen reduction. Chem Eng J 2024;495:153275.
12. Wang S, Shi L, Bai X, Li Q, Ling C, Wang J. Highly efficient photo-/electrocatalytic reduction of nitrogen into ammonia by dual-metal sites. ACS Cent Sci 2020;6:1762-71.
13. Yang X, Shang C, Zhou S, Zhao J. MBenes: emerging 2D materials as efficient electrocatalysts for the nitrogen reduction reaction. Nanoscale Horiz 2020;5:1106-15.
14. Han B, Li F. Regulating the electrocatalytic performance for nitrogen reduction reaction by tuning the N contents in Fe3@NxC20-x (x = 0~4): a DFT exploration. J Mater Inf 2023;3:24.
15. Cao J, Hu Y, Zheng Y, Zhang W, Yu B. Recent advances and challenges of nitrogen/nitrate electro catalytic reduction to ammonia synthesis. Front Energy 2024;18:128-40.
16. Ren Y, Yu C, Tan X, Huang H, Wei Q, Qiu J. Strategies to suppress hydrogen evolution for highly selective electrocatalytic nitrogen reduction: challenges and perspectives. Energy Environ Sci 2021;14:1176-93.
17. Cui Y, Ren C, Li Q, Ling C, Wang J. Hybridization state transition under working conditions: activity origin of single-atom catalysts. J Am Chem Soc 2024;146:15640-7.
18. Liu T, Jing Y, Li Y. First-principles insights into the selectivity of CO2 electroreduction over heterogeneous single-atom catalysts. J Phys Chem Lett 2024;15:6216-21.
19. Sun H, Liu J. Advancing CO2RR with O-coordinated single-atom nanozymes: a DFT and machine learning exploration. ACS Catal 2024;14:14021-30.
20. Liu S, Xing G, Liu J. Computational screening of single-atom catalysts for direct electrochemical NH3 synthesis from NO on defective boron phosphide monolayer. Appl Surf Sci 2023;611:155764.
21. Wang Q, Yu G, Yang E, Chen W. Through the self-optimization process to achieve high OER activity of SAC catalysts within the framework of TMO3@G and TMO4@G: a high-throughput theoretical study. J Colloid Interface Sci 2023;640:405-14.
22. Guo W, Wang S, Wang H, Cai Q, Zhao J. Cooperation between single atom catalyst and support to promote nitrogen electroreduction to ammonia: a theoretical insight. J Energy Chem 2024;96:336-44.
23. Yin Z, Li Y, Ye Y, et al. Sp/sp2 carbon ratio-driven high-throughput screening of electrocatalytic nitrogen reduction performance on transition metal single-atom catalysts. Rare Met 2024;43:5781-91.
24. Zhang Y, Wang X, Liu T, et al. Charge and spin communication between dual metal single-atom sites on C2N sheets: regulating electronic spin moments of Fe atoms for N2 activation and reduction. J Mater Chem A 2022;10:23704-11.
25. Liu J, Cai Y, Song R, et al. Recent progress on single-atom catalysts for CO2 electroreduction. Mater Today 2021;48:95-114.
26. Iqbal S, Safdar B, Hussain I, Zhang K, Chatzichristodoulou C. Trends and prospects of bulk and single-atom catalysts for the oxygen evolution reaction. Adv Energy Mater 2023;13:2203913.
27. Song W, Xiao C, Ding J, et al. Review of carbon support coordination environments for single metal atom electrocatalysts (SACS). Adv Mater 2024;36:e2301477.
28. Shen Y, Liang L, Zhang S, et al. Organelle-targeting surface-enhanced raman scattering (SERS) nanosensors for subcellular pH sensing. Nanoscale 2018;10:1622-30.
29. Liu S, Liu J. Rational design of highly efficient electrocatalytic single-atom catalysts for nitrogen reduction on nitrogen-doped graphene and g-C2N supports. J Power Sources 2022;535:231449.
30. Jiao D, Liu Y, Cai Q, Zhao J. Coordination tunes the activity and selectivity of the nitrogen reduction reaction on single-atom iron catalysts: a computational study. J Mater Chem A 2021;9:1240-51.
31. Long X, Huang F, Yao Z, et al. Advancements in electrocatalytic nitrogen reduction: a comprehensive review of single-atom catalysts for sustainable ammonia synthesis. Small 2024;20:e2400551.
32. Dai E, An W, Guo R, et al. Two-dimensional C2N-based single-atom catalyst with complex microenvironment for enhanced electrochemical nitrogen reduction: a descriptor-based design. J Energy Chem 2024;97:110-9.
33. Xu M, Ji Y, Qin Y, Dong H, Li Y. A universal descriptor for two-dimensional carbon nitride-based single-atom electrocatalysts towards the nitrogen reduction reaction. J Mater Chem A 2024;12:28046-55.
34. Liu Y, Zhao Z, Wei W, et al. Single-atom Fe-N4 on a carbon substrate for nitrogen reduction reaction. ACS Appl Nano Mater 2021;4:13001-9.
35. Shen P, Li X, Luo Y, Zhang N, Zhao X, Chu K. Ultra-efficient N2 electroreduction achieved over a rhodium single-atom catalyst (Rh1/MnO2) in water-in-salt electrolyte. Appl Catal B: Environ 2022;316:121651.
36. Feng X, Liu J, Chen L, et al. Hydrogen radical-induced electrocatalytic N2 reduction at a low potential. J Am Chem Soc 2023;145:10259-67.
37. Geng Z, Liu Y, Kong X, et al. Achieving a Record-High Yield Rate of 120.9 for N2 electrochemical reduction over Ru single-atom Catalysts. Adv Mater 2018;30:e1803498.
38. Ling C, Ouyang Y, Li Q, et al. A general two-step strategy-based high-throughput screening of single atom catalysts for nitrogen fixation. Small Methods 2019;3:1800376.
39. Choi CH, Kim M, Kwon HC, et al. Tuning selectivity of electrochemical reactions by atomically dispersed platinum catalyst. Nat Commun 2016;7:10922.
40. Liu C, Cui Y, Zhou Y. The recent progress of single-atom catalysts on amorphous substrates for electrocatalysis. Energy Mater 2024;4:400067.
41. Yang X, Wan J, Zhang H, Wang Y. In situ modification of the d-band in the core-shell structure for efficient hydrogen storage via electrocatalytic N2 fixation. Chem Sci 2022;13:11030-7.
42. Liu X, Qi L, Song E, Gao W. Effective descriptor for nitrogen reduction on atomic catalysts. Catal Lett 2023;153:300-10.
43. Cui X, Wang X, Fu C, et al. Tuning the local coordination environment of silver(I) coordination networks with counterions for enhanced electrocatalytic CO2 reduction. Sci China Chem 2024;67:1524-30.
44. Sun Y, Fan W, Li Y, et al. Tuning coordination structures of Zn sites through symmetry-breaking accelerates electrocatalysis. Adv Mater 2024;36:e2306687.
45. Liu W, Liu S, Guo K, et al. Low-dimensional N-heterocyclic carbenes nanomaterials: promising supports of single atom catalysts. Vacuum 2024;227:113390.
46. Liu W, Xie Y, Tong Z, Sun J, Chen L, Xu J. Heterogeneous N-heterocyclic carbenes: efficient and selective metal-free electrocatalysts for CO reduction to multi-carbon products. J CO2 Util 2023;75:102566.
47. Sun J, Zheng D, Deng F, et al. Heterogeneous N-heterocyclic carbenes supported single-atom catalysts for nitrogen fixation: a combined density functional theory and machine learning study. Appl Surf Sci 2024;644:158802.
48. Liu W, Xie Y, Peng J, Zheng D, Lu W, Xu J. Synergistic N-heterocyclic carbene and C2N integration for efficient and selective metal-free photocatalytic CO reduction to C2H5OH. Appl Surf Sci 2024;678:161122.
49. Liu W, Sun J, Xie Y, Chen L, Xu J. The effective regulation of heterogeneous N-heterocyclic carbenes: structures, electronic properties and transition metal adsorption. Phys Chem Chem Phys 2023;25:28382-92.
50. Miller TS, Jorge AB, Suter TM, Sella A, Corà F, McMillan PF. Carbon nitrides: synthesis and characterization of a new class of functional materials. Phys Chem Chem Phys 2017;19:15613-38.
51. Longuinhos R, Ribeiro-soares J. Stable holey two-dimensional C2N structures with tunable electronic structure. Phys Rev B 2018:97.
52. Wei Z, He J, Yang Y, Xia Z, Feng Y, Ma J. Fe, V-co-doped C2N for electrocatalytic N2-to-NH3 conversion. J Energy Chem 2021;53:303-8.
53. Li Y, Yang T, Wang S, Bian Z, Liu Z. A recyclable Cu@C2N nano-catalyst applied in the transformation of alkynes: pH switchable access to ketones and 1,3-diynes. Green Chem 2024;26:2540-5.
54. Kresse G, Furthmüller J. Efficiency of ab-initio total energy calculations for metals and semiconductors using a plane-wave basis set. Comput Mater Sci 1996;6:15-50.
56. Hammer B, Hansen LB, Nørskov JK. Improved adsorption energetics within density-functional theory using revised perdew-burke-ernzerhof functionals. Phys Rev B 1999;59:7413-21.
58. Bussi G, Donadio D, Parrinello M. Canonical sampling through velocity rescaling. J Chem Phys 2007;126:014101.
59. Grimme S, Antony J, Ehrlich S, Krieg H. A consistent and accurate ab initio parametrization of density functional dispersion correction (DFT-D) for the 94 elements H-Pu. J Chem Phys 2010;132:154104.
60. Bader RFW. A quantum theory of molecular structure and its applications. Chem Rev 1991;91:893-928.
61. Sahour H, Gholami V, Torkaman J, Vazifedan M, Saeedi S. Random forest and extreme gradient boosting algorithms for streamflow modeling using vessel features and tree-rings. Environ Earth Sci 2021;80:10054.
62. Umer M, Umer S, Zafari M, et al. Machine learning assisted high-throughput screening of transition metal single atom based superb hydrogen evolution electrocatalysts. J Mater Chem A 2022;10:6679-89.
63. Tamtaji M, Gao H, Hossain MD, et al. Machine learning for design principles for single atom catalysts towards electrochemical reactions. J Mater Chem A 2022;10:15309-31.
64. Ding R, Wang R, Ding Y, et al. Designing AI-aided analysis and prediction models for nonprecious metal electrocatalyst-based proton-exchange membrane fuel cells. Angew Chem Int Ed Engl 2020;59:19175-83.
65. Naceur H, Abdo HG, Igmoullan B, Namous M, Alshehri F, A Albanai J. Implementation of random forest, adaptive boosting, and gradient boosting decision trees algorithms for gully erosion susceptibility mapping using remote sensing and GIS. Environ Earth Sci 2024;83:11424.
66. Ali Y, Awwad E, Al-razgan M, Maarouf A. Hyperparameter search for machine learning algorithms for optimizing the computational complexity. Processes 2023;11:349.
67. Bischl B, Binder M, Lang M, et al. Hyperparameter optimization: Foundations, algorithms, best practices, and open challenges. WIREs Data Min Knowl 2023;13:e1484.
68. Pedregosa F, Varoquaux G, Gramfort A, et al. Scikit-learn: machine learning in python. J Mach Learn Res 2011;12:2825-30. Available from: https://dl.acm.org/doi/10.5555/1953048.2078195. [Last accessed on 28 Dec 2024].
69. Guyon I, Elisseeff A. An introduction to variable and feature selection. J Mach Learn Res 2003;3:1157-82.
70. Aas K, Jullum M, Løland A. Explaining individual predictions when features are dependent: more accurate approximations to shapley values. Artif Intell 2021;298:103502.
71. Lv X, Wei W, Huang B, Dai Y, Frauenheim T. High-throughput screening of synergistic transition metal dual-atom catalysts for efficient nitrogen fixation. Nano Lett 2021;21:1871-8.
72. Xiao Y, Shen C, Long T. Theoretical establishment and screening of an efficient catalyst for N2 electroreduction on two-dimensional transition-metal borides (MBenes). Chem Mater 2021;33:4023-34.
73. Seh ZW, Kibsgaard J, Dickens CF, Chorkendorff I, Nørskov JK, Jaramillo TF. Combining theory and experiment in electrocatalysis: Insights into materials design. Science 2017;355:eaad4998.
74. Lv SY, Huang CX, Li G, Yang LM. Electrocatalytic mechanism of N2 reduction reaction by single-atom catalyst rectangular TM-TCNQ monolayers. ACS Appl Mater Interfaces 2021;13:29641-53.
75. Liu L, Yang L, Zhang H, et al. Screening strategies for diatom metal-doped β-borophene nanosheet catalysts for electrochemical synthesis of ammonia using density functional theory. Energy Fuels 2023;37:9682-91.
76. Yue Y, Chen Y, Zhang X, Qin J, Zhang X, Liu R. High-throughput screening of highly active and selective single-atom catalysts for ammonia synthesis on WB2 (001) surface. Appl Surf Sci 2022;606:154935.
77. Pei W, Zhang W, Yu X, et al. Computational design of spatially confined triatomic catalysts for nitrogen reduction reaction. J Mater Inf 2023;3:26.
78. NIST Chemistry WebBook. NIST Standard Reference Database Number 69. Available from: https://doi.org/10.18434/T4D303. [Last accessed on 22 Dec 2024].
Cite This Article
How to Cite
Lu, W.; Zheng, D.; Ye, D.; Peng, J.; Gong, X.; Xu, J.; Liu, W. N-heterocyclic carbene coordinated single atom catalysts on C2N for enhanced nitrogen reduction. J. Mater. Inf. 2024, 4, 31. http://dx.doi.org/10.20517/jmi.2024.65
Download Citation
Export Citation File:
Type of Import
Tips on Downloading Citation
Citation Manager File Format
Type of Import
Direct Import: When the Direct Import option is selected (the default state), a dialogue box will give you the option to Save or Open the downloaded citation data. Choosing Open will either launch your citation manager or give you a choice of applications with which to use the metadata. The Save option saves the file locally for later use.
Indirect Import: When the Indirect Import option is selected, the metadata is displayed and may be copied and pasted as needed.
About This Article
Special Issue
Copyright
Data & Comments
Data
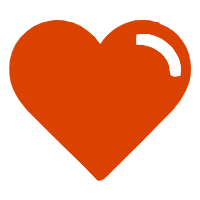
Comments
Comments must be written in English. Spam, offensive content, impersonation, and private information will not be permitted. If any comment is reported and identified as inappropriate content by OAE staff, the comment will be removed without notice. If you have any queries or need any help, please contact us at support@oaepublish.com.