Machine learning driven design of high-performance Al alloys
Abstract
Aluminum (Al) alloys with both high strength and thermal conductivity (TC) are promising structural materials for wide application across different industries. Yet, design of such alloys is challenging, since strength and TC often share a trade-off. In this paper, we build prediction models for TC and ultimate tensile strength (UTS) of Al alloys using eXtreme gradient boosting (XGBoost) and support vector machine (SVM) algorithms, respectively. The models take physical descriptors from the alloy composition into account. Lasso and Gini Impurity algorithms were adopted for feature engineering. Guided by the models, an Al-2.64Si-0.43Mg-0.10Zn-0.03Cu alloy with TC over 190 W·m-1·K-1 and UTS over 220 MPa was designed. The alloy was fabricated and tested by experiment, and its UTS and TC are close to the model prediction. Microstructure characterization suggests that the fragmented and spherical Si phase, along with a few non-spherical Si phases, may be a key reason for the improved properties.
Keywords
INTRODUCTION
Aluminum (Al) alloys are widely used in automobile[1], aerospace[2] and marine[3] industries for their high specific strength, thermal conductivity (TC) and recyclability. In most Al alloys, an increase in strength is often accompanied by a decrease in TC. This occurs because the lattice distortion and secondary phases introduced by alloying not only contribute to solution and precipitation strengthening but also significantly scatter electrons and phonons, thereby reducing TC.
Due to the differences in valence state[4], atomic radius[5], and solid solubility[6] between alloying elements and the Al matrix, the degree of lattice distortion caused by these elements varies, leading to different effects on TC. Alloying elements with greater difference in valence state have a more pronounced impact on TC. This difference in valence state alters the Brillouin zone of the matrix, either expanding or compressing it, which disrupts the periodic lattice structure, increases lattice distortion, and subsequently reduces TC[7]. The mismatch of Al and other atoms caused by difference in atom radii also disrupts the periodicity of the lattice, thus increasing the scattering of electrons[8]. The addition of Ce (atom radius = 0.183 nm), which has a larger atomic radius than Al (atom radius = 0.143 nm), can reduce the lattice distortion caused by the addition of Fe (atom radius = 0.127 nm) and Si (atom radius = 0.134 nm). This reduction in lattice distortion can create more paths for electron transition, thus improving the TC[9]. In addition, the content and morphology of secondary phases significantly affect the TC and strength of Al alloys. Highly continuous secondary phases impede electron transfer, thereby reducing the mean free path of electron migration and resulting in lower TC[10,11].
The lattice distortion and formation of secondary phases introduced by alloying elements can cause solid solution strengthening and precipitation strengthening in Al alloys. An additive model is commonly used to evaluate the contributions of individual elements to solid solution strengthening[12]: σ = ∑kiCi2/3, where ki is the scale coefficient of the ith alloying element and Ci represents its mass fraction. The scale coefficients of alloying elements are different[10,11], such as kZn = 3 MPa/wt.%-2/3, kMg = 29 MPa/wt.%-2/3, and kSi =
Machine learning (ML) has become popular in materials science research[15,16]; in particular, ML has been utilized for property prediction[17-19] and alloy design[20-22] of Al alloys. By using ML techniques, researchers can accelerate the process of predicting critical material properties, such as fracture toughness[23], corrosion resistance[24], and wear behaviour[25], with high accuracy and efficiency. Various ML algorithms such as support vector machine (SVM), tree ensembles and neural networks are used to establish separate models for predicting the performance of Al alloys with high precision[21]. Composition design and process parameter optimization based on the separate ML predicting models has proven to be an effective strategy for enhancing the performance of cast Al alloys across multiple dimensions, including hardness, strength, and modulus[26-29]. This approach enables the simultaneous optimization of multiple properties, thereby pushing the boundaries of alloy design toward higher application requirements. In addition, multi-objective optimization can also achieve a trade-off between conflicting performances. However, multi-objective optimization requires a large amount of data and accurate performance prediction models on a certain alloy series[18,21,30]. Insufficient data scale or quality can result in inaccurate outcomes during multi-objective optimization[31]. Regarding the input features, feature expansion and selection play crucial roles in incorporating physical parameters into prediction models and identifying the most significant features. Various atomic descriptors, such as atomic radius, electronegativity, modulus, and melting point, are commonly employed to enhance input features, thereby improving model interpretability[32]. Feature selection methods, including Lasso, Gini impurity, and correlation coefficients, are essential for identifying the most important features from an extensive set of expanded features. This process reduces model complexity and minimizes prediction errors[33].
This paper aims to use ML to design cast Al alloys with both high TC and ultimate tensile strength (UTS). Physical descriptors of the composition were added to the input feature list, followed by feature engineering to determine the optimal feature sets. ML models for predicting UTS and TC were developed separately. Guided by these models, a new Al alloy was designed, fabricated, and tested. The strength and TC closely align with the model predictions, and the underlying mechanisms are analyzed.
METHODS
We performed data collection, feature calculation, feature selection, prediction model development, new alloy design and validation [Figure 1]. Firstly, 277 as-cast Al alloys were collected from published papers one by one manually to ensure no mistakes occurred during data collection to establish an initial dataset [Supplementary Materials]. Data were only collected for cast Al alloys with no other processing techniques such as wrought or coating. The dataset included information about alloy composition, processing parameters of solution treatment and aging (i.e., time and temperature), TC, and UTS values. Table 1 shows a few sample data[34-36]. The alloy composition includes 22 elements (Si, Fe, Cu, B, Bi, Pb, Zn, Mn, Mg, Sn, Ti, V, Mo, Ni, Ce, Co, Cr, La, Sc, Sr, Zr, and Al) and their contents are given in wt.%. The processing parameters include the solution temperature [denoted as sol_T (oC)], solution time [denoted as sol_time (h)], quench temperature [denoted as quench_T (oC)], aging temperature [denoted as aging_T (oC)], and aging time [denoted as aging_time (h)]. Data format for the columns is all numeric and consistent. No outliers are identified from the dataset and no data were deleted. The missing values of processing parameters were replaced by -1. Figure 2 shows the distribution of UTS and TC of the dataset. The UTS values range from 50 to 350 MPa; the TC values range from 100 to 220 W·m-1·K-1.
Figure 2. The UTS and TC of the 277 data in the dataset. UTS: Ultimate tensile strength; TC: thermal conductivity.
Sample data in the dataset
Composition | sol_T (°C) | sol_time (h) | quench_T (°C) | aging_T (°C) | aging_time (h) | UTS (MPa) | TC (W·m-1·K-1) | Ref. |
Al-8Si-0.512Mg-0.55Fe-0.08Sr-0.01Cu | 535 | 0.5 | 50 | 170 | 3 | 304 | 171 | [34] |
Al-8Si-0.512Mg-0.55Fe-0.08Sr-0.01Cu | 535 | 4 | 50 | 170 | 3 | 294 | 163 | [34] |
Al-5Si-2Cu-2Mg | 500 | 6.5 | 85 | 250 | 4 | 249 | 169 | [35] |
Al-5Si-2Cu-2Mg-0.05Zr | 500 | 6.5 | 85 | 250 | 4 | 228 | 163 | [35] |
Al-5Si-2Cu-2Mg-0.1Zr | 500 | 6.5 | 85 | 250 | 4 | 226 | 167 | [35] |
Al-5Si-2Cu-2Mg-0.12Zr | 500 | 6.5 | 85 | 250 | 4 | 230 | 171 | [35] |
Al-5Si-2Cu-2Mg-0.19Zr | 500 | 6.5 | 85 | 250 | 4 | 210 | 165 | [35] |
Al-10.5Si-1.75Cu-0.76Zn-0.23Mg | 490 | 0.25 | 50 | 220 | 1 | 312 | 155 | [36] |
Al-10.5Si-2.43Cu-0.76Zn-0.24Mg | 490 | 0.25 | 50 | 220 | 0.5 | 349 | 152 | [36] |
To explore the composition information, physical descriptors must be added[37,38] to expand the feature list. A total of 42 physical parameters of elements were exported from the Materials Project[39], which are listed in Table 2. From the alloy composition, the average and variance of each parameter were then used as input features:
42 physical parameters of elements
Name | Definition |
a | Cell parameter (pm) |
b | Cell parameter (pm) |
c | Cell parameter (pm) |
AN | Atomic number |
AR | Atomic radius (pm) |
B | Bulk modulus (GPa) |
BIPT | Block in periodic table |
BP | Boiling point (K) |
C | Specific heat (J/K*mol) |
CR | Covalent radius (pm) |
DS | Density of solid (g/cm3) |
E | Young’s modulus (GPa) |
E0 | The change in enthalpy of a substance from 0 to 298 K (kJ/mol) |
EA | Electron affinity (kJ/mol) |
ENA | Enthalpy of atomization (kJ/mol) |
ENF | Enthalpy of fusion (kJ/mol) |
ENV | Enthalpy of vaporization (kJ/mol) |
FIE | First ionization energies (kJ/mol) |
G | Shear modulus (GPa) |
GIPT | Group in periodic table |
L | Angular quantum number |
M | Magnetic quantum number |
MP | Melting point (K) |
MV | Molar volume (cm3) |
N | Principal quantum number |
PE | Pauling electronegativity |
PIPT | Period in periodic table |
PR | Poisson ratio |
RAM | Relative atomic mass |
S0 | Standard entropy (J/K*mol) |
SE | Sanderson electronegativity |
SIE | Second ionization energies (kJ/mol) |
SOL | Solid solubility in Al (wt.%) |
TIE | Third ionization energies (kJ/mol) |
VE | Number of valence electrons |
VED | Number of valence electrons in the d orbital |
VEF | Number of valence electrons in the f orbital |
VEP | Number of valence electrons in the p orbital |
VES | Number of valence electrons in the s orbital |
VR | Van der Waals radius (pm) |
VS | Valence state |
WF | Work function (eV) |
where ci is the content of the element (wt.%) and the pi is the physical parameter of the element. The initial list contains 111 features, including 22 composition features, 84 physical descriptors and five processing features.
Subsequently, two feature selection methods based on Lasso and Gini impurity were employed to identify the optimal feature list for building ML models. Tree ensemble and SVM algorithms were used to predict TC and UTS, with a training-testing split ratio of 85% to 15%. The R2 and root mean square error (RMSE) metrics are used to evaluate the accuracy of the ML models:
where n is the number of data in the training/test set, yi is the actual value of the ith data,
RESULTS AND DISCUSSION
Feature engineering and ML
Choosing the appropriate features is necessary to establish reliable ML models, especially for a dataset with only 277 data. The removal of unimportant features can reduce the calculation complexity and enhance the prediction accuracy. The Lasso and Gini impurity selection are popular methods for feature selection. The Lasso algorithm[40] adds the L1 regularization term into the loss function of linear regression:
where yi is the actual value of the ith data,
Figure 3. The number of features with non-zero coefficient decreases with the increase of α in the (A) TC dataset and (B) UTS dataset. TC: Thermal conductivity; UTS: ultimate tensile strength.
Gini impurity[43], which reflects the uncertainty reduction after branching in tree models, is a common index to measure the feature importance in the prediction using tree algorithms. The feature will be more important when the prediction uncertainty reduces more after its inclusion. Three different tree algorithms including random forest (RF), eXtreme gradient boosting (XGBoost) and gradient boosting decision tree (GBDT) are used to calculate the Gini impurity of every feature. The average of feature importance calculated by these three algorithms is ranked in Figure 4, with the 26 most important features remaining as the input of performance prediction.
Figure 4. The feature importance ranking of (A) the TC features and (B) the UTS features. TC: Thermal conductivity; UTS: ultimate tensile strength.
Normalization was applied before the UTS prediction but not for the TC prediction:
where
To prove the necessity of feature expansion and selection, the model accuracy adopting different features is compared. The XGBoost algorithm is used to predict TC and the SVM algorithm is used to predict UTS, with four types of features as the input. As shown in Figures 5-7, when only composition information containing 22 elements is used as the model input, the prediction has the lowest accuracy. The accuracy of TC and UTS prediction improves substantially from 0.68 (composition only) to 0.82 (composition + processing). The prediction accuracy further increases after feature expansion. After feature selection by Lasso and Gini impurity algorithm, the R2 of TC and UTS prediction are both above 0.9.
Figure 5. The comparison of predicted and actual TC using XGBoost algorithm and input of (A) composition information, (B) composition and processing parameter information, (C) composition and processing parameter information with feature expansion (D) composition and processing parameter information with feature expansion and selection. TC: Thermal conductivity; XGBoost: eXtreme gradient boosting.
Figure 6. The comparison of predicted and actual UTS using SVM algorithm and input of (A) composition information, (B) composition and processing parameter information, (C) composition and processing parameter information with feature expansion, (D) composition and processing parameter information with feature expansion and selection. UTS: Ultimate tensile strength; SVM: support vector machine.
Figure 7. The R2 and RMSE of (A) TC and (B) UTS using different features as input. RMSE: Root mean squared error; TC: thermal conductivity; UTS: ultimate tensile strength.
Considering two performance prediction models have been established, the optimization of the as-cast Al alloy composition is necessary. Because the processing parameters have numerous missing values in the dataset, the following heat treatment parameters are used: solution treatment at 500 °C for 6 h and aging at 250 °C for 4 h, which is the most frequently used heat treatment condition in the dataset. Since Si, Mg, Zn, and Cu are the most common alloying elements, 800 virtual Al-Si-Mg-Zn-Cu alloy compositions are generated randomly, and TC and UTS values are predicted by the models. As shown in Figure 8, among the 800 virtual samples, a sample with the composition of Al-2.64Cu-0.43Mg-0.10Zn-0.03Si has high predicted values for both TC and UTS.
Experiment validation
According to the recommended composition, the alloy was fabricated by commercial-purity Al (99.95%), Mg (99.95%), Zn (99.95%), Mg-10 wt.% Si, and Mg-10 wt.% Cu master alloys. The raw materials were melted in an electric resistance furnace at 720 °C. The molten alloy was stirred manually and held for homogenization, and then cast into a cylinder ingot with Φ = 60 mm. The ingots were solution-treated at 500 °C for 6 h and aged at 250 °C for 4 h.
Three dog-bone-shaped tensile specimens with dimensions of 18 (L) mm × 3.4 (W) mm × 2 (T) mm were cut from the ingots. Tensile tests were conducted using a Zwick-100 kN instrument with a BT2-EXMACWD at a constant strain rate of 1.0 × 10-4·s-1. The TC λ was calculated by λ = ραCp[44] where ρ is the density of Al (2.7 g·cm-3), α is the thermal diffusivity, Cp is the specific heat capacity of Al (0.88 kJ·kg-1·K-1). The thermal diffusivity α was measured three times for each specimen (Ф 12.7 × H 2.0 mm) at room temperature (25 °C) using the laser transient TC meter (LFA467HT, Netzsch).
The predicted and actual values obtained by strength and TC tests are listed in Table 3 and the tensile stress-strain curve is shown in Figure 9. The relative errors for TC and UTS predictions are 5.9% and 5.1%, respectively. As shown in Figure 10[45-48], compared to other as-cast alloys reported in the literature, the Al-2.64Si-0.43Mg-0.10Zn-0.03Cu alloy has TC > 190 W·m-1·K-1 and UTS > 220 MPa, making it a good candidate material when both strength and TC are required.
The predicted and actual values of TC and UTS of the Al-2.64Si-0.43Mg-0.10Zn-0.03Cu alloy
Predicted value | Actual value | Relative error | |
TC | 179.7 W·m-1·K-1 | 191.0 W·m-1·K-1 | 5.9% |
UTS | 209.8 MPa | 221.0 MPa | 5.1% |
X-ray diffraction (XRD) analysis was conducted for the Al-2.64Si-0.43Mg-0.10Zn-0.03Cu alloy to determine the phase composition. As shown in Figure 11A, apart from Al peaks, only diffraction peaks of Si are present in the alloy. Figure 11B and C shows the optical micrograph (OM) and scanning electron microscopy (SEM) of the Al-2.64Si-0.43 Mg-0.10Zn-0.03Cu alloy. The microstructure of the alloy consists of primary α-Al cells, with spherical Si particles along with a few non-spherical Si particles. The energy-dispersive X-ray spectroscopy (EDS) results also confirm the XRD results. As shown in Figure 11D, Cu, Zn and Mg elements exist as solutes in Al, while the Si element is found in the secondary phase.
Figure 11. (A) XRD patterns (B) OM (C) SEM micrograph (D) EDS maps of the Al-2.64Si-0.43Mg-0.10Zn-0.03Cu alloy. XRD: X-ray diffraction; OM: optical micrograph; SEM: scanning electron microscopy; EDS: energy-dispersive X-ray spectroscopy.
The formation of Si secondary phase reduces the Si solute concentration in Al lattice. Because of the significant atomic radius difference between the Al atom (RAl = 118 pm) and the Si atom (RSi = 143 pm), Si solutes significantly reduce TC of Al alloys[49]. The precipitation of Si reduces the amount of Si solutes in the Al matrix, resulting in higher TC[50].
The morphology of the secondary phase also influences TC. Continuous secondary phases reduce the average free path of electrons[51], an effect that becomes more pronounced as the size of the secondary phase increases[52]. In the current alloy, the Si particles are predominantly spherical and exhibit discontinuous interfaces, allowing electrons to travel longer distances within the Al matrix[53]. As a result, TC is less adversely impacted[54].
The yield strength, σy, of Al-2.64Si-0.43Mg-0.10Zn-0.03Cu alloy can be expressed as:
where σ0 is the yield stress of pure Al (35 MPa[55]), σss is the solid-solution strengthening, σgs is the grain size strengthening, and σPPT is the precipitation strengthening.
Solid-solution strengthening, σss, can be calculated as the sum of the individual effects of each alloying element on strength enhancement, which is expressed by:
where ki is the contributing factor of alloying element (kSi = 9.3 MPa/at.%, kCu = 16.2 MPa/at.%, kMg =
The strength contribution from grain size strengthening σgs was calculated using the Hall–Petch equation[58]:
where k is a constant (0.06 MPa·m-1/2 for Al alloys[59]) and d is the grain size. The σgb value of Al-2.64Si-0.43Mg-0.10Zn-0.03Cu alloy is 6.7 MPa based on the average grain size (~80 μm).
Considering the yield stress of ~183 MPa in the Al-2.64Si-0.43Mg-0.10Zn-0.03Cu alloy, precipitation strengthening is likely to be important. The fragmented eutectic Si particles provide only a limited contribution to overall strengthening. It has been reported that eutectic Si particles with a radius of ~2 μm and a volume fraction of ~6% contribute around 4 MPa to the strength[60]. In contrast, Si nanoprecipitates and solute clusters formed during the aging process can significantly enhance the strength[60,61]. It has been shown that nanoscale Si particles and clusters, with radii ranging from 1 to 10 nm, provide strong Orowan strengthening, exceeding 100 MPa[62]. Additionally, nanoscale Si particles can act as dislocation pinning sites, leading to dislocation accumulation and further contributing to dislocation strengthening[63].
Key factors affecting TC and UTS
The three most important alloying elements affecting TC and UTS are listed in Table 4, in which Mg, Si, and Fe have the greatest impact on TC and Mn, and Cu and Zn have the greatest impact on UTS. Influence levels of alloying elements on TC of Al alloys depend on the physical parameters of these elements, such as valence electrons, atom radius difference, and so on[64]. Due to the high solid solubility of Mg in Al
The three most important alloying elements affecting TC and UTS
TC | UTS |
Mg | Mn |
Si | Cu |
Fe | Zn |
Mn usually exists as Al6Mn phases, which can improve the strength of the alloy by stabilizing the precipitation phases[67]. Cu acts as solution atom and forms Al2Cu phase, which can generate both solid solution strengthening and precipitation strengthening. In Al-Zn-Mg alloys, when the Cu content is below
CONCLUSIONS
In this work, we established ML models for predicting TC and UTS of as-cast Al alloys. The feature expansion and feature selection using Lasso and Gini impurity took more physical and chemical factors into consideration and significantly improve the prediction accuracy. The R2 for the prediction of TC and UTS are above 0.9. An alloy with the composition of Al-2.64Cu-0.43Mg-0.10Zn-0.03Si alloy is recommended by the models. The alloy was fabricated, and it exhibits TC > 190 W·m-1·K-1 and UTS > 220 MPa, which are consistent with the model prediction. The microstructure analysis indicates that the alloy contains fragmented and spherical precipitates which reduce the electron scattering and offer precipitates strengthening, thereby improving the TC and UTS.
DECLARATIONS
Authors’ contributions
Writing - original draft, methodology, software, validation, formal analysis: Lu Z
Writing - review and editing, supervision, conceptualization: Kapoor I
Investigation: Li Y
Methodology: Liu Y
Supervision, resources, project administration: Zeng X
Writing - review and editing, supervision, conceptualization: Wang L
Availability of data and materials
The original data is provided in the Supplementary Materials.
Financial support and sponsorship
This work was supported by SJTU-Warwick Joint Seed Fund 2023/24 (SJTU2308), Shenzhen Science and Technology Program (KJZD20231023092902005), and UK Engineering and Physical Sciences Research Council Impact Acceleration Account (G.ESWM.0730.EXP).
Conflicts of interest
All authors declared that there are no conflicts of interest.
Ethical approval and consent to participate
Not applicable.
Consent for publication
Not applicable.
Copyright
© The Author(s) 2024.
Supplementary Materials
REFERENCES
1. Hirsch J. Recent development in aluminium for automotive applications. T Nonferr Metal Soc 2014;24:1995-2002.
2. Williams JC, Starke EA. Progress in structural materials for aerospace systems. Acta Mater 2003;51:5775-99.
3. Ertuğ B, Kumruoğlu LC. 5083 type Al-Mg and 6082 type Al-Mg-Si alloys for ship building Am J Eng Res 2015. pp. 146-50. Available from: https://www.ajer.org/papers/v4(03)/T04301460150.pdf. [Last accessed on 2 Nov 2024]
5. Klemens PG, Williams RK. Thermal conductivity of metals and alloys. Int Metal Rev 1986;31:197-215.
6. Pan H, Pan F, Yang R, et al. Thermal and electrical conductivity of binary magnesium alloys. J Mater Sci 2014;49:3107-24.
7. Schindler AI, Salkovitz EI. Brillouin zone investigation of Mg alloys. I. Hall Effect and conductivity. Phys Rev 1953;91:1320-2.
8. Zhou Y, Zhang X, Zhong G, et al. Elucidating thermal conductivity mechanism of Al-9Si based alloys with trace transition elements (Mn, Cr, V). J Alloys Compd 2022;907:164446.
9. Luo G, Zhou X, Li C, Du J, Huang Z. Design and preparation of Al-Fe-Ce ternary aluminum alloys with high thermal conductivity. T Nonferr Metal Soc 2022;32:1781-94.
10. Choi S, Cho H, Kumai S. Effect of the precipitation of secondary phases on the thermal diffusivity and thermal conductivity of
11. Lee W, Lee J, Kyoung W, Lee H, Lee H, Kim D. Effect of inhomogeneous composition on the thermal conductivity of an Al alloy during the precipitation-hardening process. J Mater Res Technol 2020;9:10139-47.
12. Esmaeili S, Lloyd D, Poole W. A yield strength model for the Al-Mg-Si-Cu alloy AA6111. Acta Mater 2003;51:2243-57.
13. Lin B, Fan T, Li H, Zhao Y, Zhang W, Liu K. Microstructure and high temperature tensile properties of Al–Si–Cu–Mn–Fe alloys prepared by semi-solid thixoforming. T Nonferr Metal Soc 2021;31:2232-49.
14. Chen ZQ. Combining effect of Er and Sr on microstructure and mechanical properties of As-carted A356 alloy. Rare Metal Mat Eng 2020;49:3388-94.
15. Fang Z, Roy K, Xu J, Dai Y, Paul B, Lim JB. A novel machine learning method to investigate the web crippling behaviour of perforated roll-formed aluminium alloy unlipped channels under interior-two flange loading. J Build Eng 2022;51:104261.
16. Dai Y, Roy K, Fang Z, Chen B, Raftery GM, Lim JB. A novel machine learning model to predict the moment capacity of cold-formed steel channel beams with edge-stiffened and un-stiffened web holes. J Build Eng 2022;53:104592.
17. Juan Y, Niu G, Yang Y, Dai Y, Yang J, Zhang J. Machine learning-based identification method of new strengthening element and the study on Al-Zn-Mg-Cu-Zr-Hf alloy. Maters Today Commun 2024;38:108359.
18. Juan Y, Niu G, Yang Y, et al. Accelerated design of Al−Zn−Mg−Cu alloys via machine learning. T Nonferr Metal Soc 2024;34:709-23.
19. Jain S, Jain R, Dewangan S, Bhowmik A. A machine learning perspective on hardness prediction in multicomponent Al-Mg based lightweight alloys. Mater Lett 2024;365:136473.
20. Motamedi M, Nikzad MH, Nasri MR. Mixture design optimization and machine learning-based prediction of Al-Mg alloy composite reinforced by Zn nanoparticles: a molecular dynamics study. Mater Today Commun 2023;37:107473.
21. Shen Q, Yin Q, Zhao H, et al. Inversely optimized design of Al-Mg-Si alloys using machine learning methods. Comput Mater Sci 2024;242:113107.
22. Xue D, Wei W, Shi W, et al. Optimization of stabilized annealing of Al-Mg alloys utilizing machine learning algorithms. Mater Today Commun 2023;35:106177.
23. Fatriansyah JF, Satrio MRR, Federico A, Suhariadi I, Dhaneswara D, Gascoin N. Machine learning-based forward and inverse designs for prediction and optimization of fracture toughness of aluminum alloy. Results Eng 2024;23:102717.
24. Jiang L, Fu H, Zhang Z, et al. Synchronously enhancing the strength, toughness, and stress corrosion resistance of high-end aluminum alloys via interpretable machine learning. Acta Mater 2024;270:119873.
25. Santhosh N, Praveena B, Jain R, et al. Analysis of friction and wear of aluminium AA 5083/WC composites for building applications using advanced machine learning models. Ain Shams Eng J 2023;14:102090.
26. Jiang L, Wang C, Fu H, Shen J, Zhang Z, Xie J. Discovery of aluminum alloys with ultra-strength and high-toughness via a property-oriented design strategy. J Mater Sci Technol 2022;98:33-43.
27. Li H, Li X, Li Y, et al. Machine learning assisted design of aluminum-lithium alloy with high specific modulus and specific strength. Mater Design 2023;225:111483.
28. Mokhtari MA, Nikzad MH. Multi-objective optimization and comparison of machine learning algorithms for the prediction of tensile properties of aluminum-magnesium alloy. Mater Today Commun 2024;40:109476.
29. Chaudry U, Hamad K, Abuhmed T. Machine learning-aided design of aluminum alloys with high performance. Mater Today Commun 2021;26:101897.
30. Suh JS, Kim YM, Yim CD, Suh B, Bae JH, Lee HW. Interpretable machine learning-based analysis of mechanical properties of extruded Mg-Al-Zn-Mn-Ca-Y alloys. J Alloys Compd 2023;968:172007.
31. Zille H, Ishibuchi H, Mostaghim S, Nojima Y. Weighted optimization framework for large-scale multi-objective optimization. In: Proceedings of the 2016 on Genetic and Evolutionary Computation Conference Companion; Denver, USA; 2016. pp. 83-4.
32. Bai P, Shang C, Zhu D, et al. The interpretable descriptors for fatigue performance of wrought aluminum alloys. J Mater Res Technol 2024;32:3423-31.
33. Bak C, Roy AG, Son H. Quality prediction for aluminum diecasting process based on shallow neural network and data feature selection technique. CIRP J Manuf Sci Tec 2021;33:327-38.
34. Dong ZQ, Wang JG, Guan ZP, et al. Effect of short T6 heat treatment on the thermal conductivity and mechanical properties of different casting processes Al-Si-Mg-Cu alloys. Metals 2021;11:1450.
35. Bolibruchová D, Širanec L, Matejka M. Selected properties of a Zr-containing AlSi5Cu2Mg alloy intended for cylinder head castings. Materials 2022;15:4798.
36. Lumley RN, Deeva N, Larsen R, Gembarovic J, Freeman J. The role of alloy composition and T7 heat treatment in enhancing thermal conductivity of aluminum high pressure diecastings. Metall Mater Trans A 2013;44:1074-86.
37. Zhang H, Fu H, Zhu S, Yong W, Xie J. Machine learning assisted composition effective design for precipitation strengthened copper alloys. Acta Mater 2021;215:117118.
38. Carruthers C, Teitelbaum H. The linear mixture rule in chemical kinetics. II. Thermal dissociation of diatomic molecules. Chem Phys 1988;127:351-62.
39. Jain A, Ong SP, Hautier G, et al. Commentary: the materials project: a materials genome approach to accelerating materials innovation. APL Mater 2013;1:011002.
41. Li Z, Long Z, Lei S, Yang L, Zhang W, Zhang T. Explicit expressions of the saturation flux density and thermal stability in Fe-based metallic glasses based on Lasso regression. Intermetallics 2021;139:107361.
42. Raguraman S, Priyadarshini MS, Nguyen T, et al. Machine learning-guided accelerated discovery of structure-property correlations in lean magnesium alloys for biomedical applications. J Magnes Alloy 2024;12:2267-83.
43. Ho TK. Random decision forests. In Proceedings of 3rd International Conference on Document Analysis and Recognition; 1995 Aug 14-16; Montreal, Canada. IEEE; 2002. pp. 278-82.
44. Yao F, You G, Zeng S, Zhou K, Peng L, Ming Y. Fabrication, microstructure, and thermal conductivity of multilayered Cu mesh/AZ31 Mg foil composites. J Mater Res Technol 2021;14:1539-50.
45. Wang K, Li W, Xu W, Hou S, Hu S. Simultaneous improvement of thermal conductivity and strength for commercial A356 alloy using strontium modification process. Met Mater Int 2021;27:4742-56.
46. Vandersluis E, Bois-brochu A, Ravindran C, Chiesa F. Mechanical properties and conductivity of low-pressure die-cast 319 aluminum prepared with hot isostatic pressing, thermal treatment, or chemical treatment. J Mater Eng Perform 2020;29:2335-45.
47. Yang Z, He X, Li B, Atrens A, Yang X, Cheng H. Influence of Si, Cu, B, and trace alloying elements on the conductivity of the Al-Si-Cu alloy. Materials 2022;15:426.
48. Kim CW, Cho JI, Choi SW, Kim YC. The effect of alloying elements on thermal conductivity of aluminum alloys in high pressure die casting. Adv Mater Res 2013;813:175-8.
49. Luo G, Zhou X, Li C, Huang Z, Du J. A Quantitative study on the interaction between silicon content and heat treatment on thermal conductivity of Al-Si binary alloys. Int J Metalcast 2022;16:1585-94.
50. Gan J, Du J, Wen C, Zhang G, Shi M, Yuan Z. The effect of Fe content on the solidification pathway, microstructure and thermal conductivity of hypoeutectic Al–Si alloys. Int J Metalcast 2022;16:178-90.
51. Tian L, Anderson I, Riedemann T, Russell A. Modeling the electrical resistivity of deformation processed metal–metal composites. Acta Mater 2014;77:151-61.
52. Gan J, Huang Y, Du J, Wen C, Liu J. Synchronous improvement in thermal conductivity and mechanical properties of Al–7Si–0.6Fe–0.5Zn cast alloy by B/La/Sr composite modification. Mater Res Express 2020;7:086501.
53. Mulazimoglu MH, Drew RAL, Gruzleski JE. Solution treatment study of cast Al–Si alloys by electrical conductivity. Can Metall Quart 1989;28:251-8.
54. Li K, Zhang J, Chen X, et al. Microstructure evolution of eutectic Si in Al-7Si binary alloy by heat treatment and its effect on enhancing thermal conductivity. J Mater Res Technol 2020;9:8780-6.
55. Shanmugasundaram T, Heilmaier M, Murty B, Sarma VS. On the Hall–Petch relationship in a nanostructured Al–Cu alloy. Mat Sci Eng A 2010;527:7821-5.
56. Kammer C. Aluminum and aluminum alloys. In: Warlimont H, Martienssen W, Editors. Springer handbook of materials data. Springer International Publishing; 2018. pp. 161-97.
57. Zou Y, Wu X, Tang S, et al. Investigation on microstructure and mechanical properties of Al-Zn-Mg-Cu alloys with various Zn/Mg ratios. J Mater Sci Technol 2021;85:106-17.
58. Hansen N. The effect of grain size and strain on the tensile flow stress of aluminium at room temperature. Acta Metall 1977;25:863-9.
59. Thangaraju S, Heilmaier M, Murty BS, Vadlamani SS. On the estimation of true Hall–Petch constants and their role on the superposition law exponent in Al alloys. Adv Eng Mater 2012;14:892-7.
60. Lee S, Seo N, Kang M, Son SB, Lee S, Jung J. Natural aging-induced nanoprecipitation and its impact on tensile properties of Al–Si–Cu–Mg cast alloy. Mater Charact 2024;215:114204.
61. Gomes LF, Spinelli J, Bogno A, Gallerneault M, Henein H. Influence of annealing treatment on Si morphology and strength of rapid solidified Al-12 wt% Si powders. J Alloys Compd 2019;785:1077-85.
62. Zheng G, Li H, Lei C, Fu J, Bian T, Yang J. Natural aging behaviors and mechanisms of 7050 and 5A90 Al alloys: a comparative study. Mat Sci Eng A 2018;718:157-64.
63. Zhang X, Huang L, Zhang B, Chen Y, Liu F. Microstructural evolution and strengthening mechanism of an Al–Si–Mg alloy processed by high-pressure torsion with different heat treatments. Mat Sci Eng A 2020;794:139932.
64. Shin J, Ko S, Kim K. Development and characterization of low-silicon cast aluminum alloys for thermal dissipation. J Alloys Compd 2015;644:673-86.
65. Klemens PG. Deviations from Matthiessen’s rule and the electronic thermal conductivity of alloys. In: Mirkovich VV, Editor. Thermal conductivity 15. Springer US; 1978. pp. 203-7.
66. Chen JK, Hung HY, Wang CF, Tang NK. Thermal and electrical conductivity in Al–Si/Cu/Fe/Mg binary and ternary Al alloys. J Mater Sci 2015;50:5630-9.
67. Wang Y, Cao L, Wu X, Lin X, Yao T, Peng L. Multi-alloying effect of Ti, Mn, Cr, Zr, Er on the cast Al-Zn-Mg-Cu alloys. Mater Charact 2023;201:112984.
68. Li H, Cao F, Guo S, et al. Effects of Mg and Cu on microstructures and properties of spray-deposited Al-Zn-Mg-Cu alloys. J Alloys Compd 2017;719:89-96.
69. Ma K, Wen H, Hu T, et al. Mechanical behavior and strengthening mechanisms in ultrafine grain precipitation-strengthened aluminum alloy. Acta Mater 2014;62:141-55.
Cite This Article

How to Cite
Lu, Z.; Kapoor, I.; Li, Y.; Liu, Y.; Zeng, X.; Wang, L. Machine learning driven design of high-performance Al alloys. J. Mater. Inf. 2024, 4, 19. http://dx.doi.org/10.20517/jmi.2024.21
Download Citation
Export Citation File:
Type of Import
Tips on Downloading Citation
Citation Manager File Format
Type of Import
Direct Import: When the Direct Import option is selected (the default state), a dialogue box will give you the option to Save or Open the downloaded citation data. Choosing Open will either launch your citation manager or give you a choice of applications with which to use the metadata. The Save option saves the file locally for later use.
Indirect Import: When the Indirect Import option is selected, the metadata is displayed and may be copied and pasted as needed.
About This Article
Copyright
Data & Comments
Data
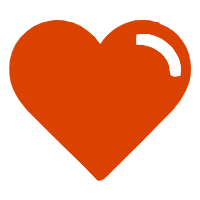
Comments
Comments must be written in English. Spam, offensive content, impersonation, and private information will not be permitted. If any comment is reported and identified as inappropriate content by OAE staff, the comment will be removed without notice. If you have any queries or need any help, please contact us at support@oaepublish.com.