Bayesian Monte Carlo-assisted life cycle assessment of lithium iron phosphate batteries production for electric vehicles under uncertainty
Abstract
The environmental performance of electric vehicles (EVs) largely depends on their batteries. However, the extraction and production of materials for these batteries present considerable environmental and social challenges. Traditional environmental assessments of EV batteries often lack comprehensive uncertainty analysis, resulting in evaluations that may not be sufficiently accurate or reliable. To address this issue and quantify uncertainties in the evaluation of EV battery production, based on the foreground data of the lithium-iron-phosphate battery pack manufacturing process, the ReCiPe midpoint methodology was adopted to quantify the lifecycle environmental impacts using eleven environmental indicators. Given the parametric uncertainties in the manufacturing process of lithium-iron-phosphate, a Bayesian Monte Carlo analytical method was developed to determine the probability distribution of global warming potential and acidification potential. Local sensitivity analysis was conducted to identify the influential factors of selected environmental indicators. Results indicated that battery cell production is the largest contributor to the entire emissions and resource utilization (comprising 63.38% of the production of each battery pack), in which cathode electrode paste and anode current collector manufacturing processes were the two predominant components with the highest environmental burdens. Sensitivity analysis showed that environmental indicators were quite sensitive to different substances in the battery pack. The application of Bayesian Monte Carlo uncertainty analysis effectively reduces the uncertainties in life cycle assessment. This study would contribute to uncertainty quantification towards solid life cycle assessment.
Keywords
INTRODUCTION
The advancement of electric vehicles (EVs) is critical in mitigating greenhouse gas emissions and is essential for achieving carbon neutrality objectives. By replacing internal combustion engines with battery-powered systems, EVs are claimed to have significant potential for emission reduction[1]. Over the past decade, the growth in EV development has been substantial, with the number of available models surpassing 500 by 2022, more than doubling since 2018[2]. The environmental impact of batteries has been a hotspot across academia, industry, and governmental organizations, particularly the European Union which enacted the new EU Batteries Regulation recently[3]. This regulation enforces comprehensive sustainability requirements to ensure lower lifecycle carbon emissions[4]. Thus, accurately estimating the lifecycle emissions of EV batteries is critically important. Life cycle assessment (LCA), widely acknowledged as an environmental management methodology, is the primary tool for such estimation[5].
The manufacturing stage has been identified as the most significant source of environmental impact for EV batteries[6]. This study aims to address the critical challenge of accurately evaluating the environmental impacts of EV battery production and mitigating inconsistencies in LCA results. The burgeoning efforts have been made to investigate the environmental impacts of the EV battery production phase[7]. Amidst these endeavors, many studies commonly adopt an approach grounded in the LCA framework, scrutinizing and listing the life cycle inventory (LCI) of EV batteries. For example, Temporelli et al.[6] assessed the ecological impacts of EV batteries throughout their life cycle, including material extraction, manufacturing, use, and end-of-life phases, highlighting the substantial environmental burdens during the production stage. However, the manufacturing phase of EV batteries is fraught with uncertainties. Although the general LCA framework exists[8], studies on batteries of the same type show diverse or even contradictory results. For instance, Hao et al.[9] and Shu et al.[10] reported 46.43 and 109.32 kg CO2 eq, respectively, when manufacturing a 1 kWh lithium-iron-phosphate (LFP) battery. However, Marques et al.[11] stated a much higher carbon emission during the production stage, with a value of 282.6 kg CO2 eq. Unsurprisingly, this large variability stems from various uncertainties, such as parameter-related uncertainty (e.g., variations in the energy sources used for manufacturing and the efficiency of material utilization[12,13]). Therefore, integration of uncertainty analysis into LCA is non-trivial for a reliable environmental assessment of EV batteries[14].
The absence of primary and reliable industry data necessitates several assumptions; for example, different energy structures for battery manufacturing can lead to variations in life cycle impact assessment (LCIA) results[15]. Current literature claims that uncertainty could influence the environmental burden significantly. Kim et al.[16] discussed the variability in the environmental impacts due to different data sources and assumptions, highlighting that cradle-to-gate emissions from lithium-ion battery (LiB) production could range from 56 to 494 kg CO2-eq per kWh depending on the manufacturing scenario. Similarly, Hao et al.[9] acknowledged the inherent uncertainties in the material sourcing and manufacturing processes of EV batteries, noting that greenhouse gas emissions from LiB production in China can vary by as much as 30% based on the regional energy mix and efficiency of manufacturing processes. Despite recognizing the influence of uncertainty, their study lacks a systematic approach to quantify these uncertainties across different stages of the battery lifecycle. This gap in comprehensive uncertainty analysis is a critical issue, as it undermines the reliability and comparability of LCA results, ultimately affecting policy and decision-making processes aimed at promoting sustainable practices in the EV industry. Thus, there is an urgent need for more rigorous and standardized approaches to uncertainty analysis in LCA studies of EV batteries to ensure more accurate and reliable environmental impact assessments.
Common uncertainty analysis techniques have been applied to the LCA of batteries, such as scenario analysis[17], sensitivity analysis[18], and probability modeling[19]. However, sensitivity analysis often oversimplifies by changing one variable at a time, failing to capture the interplay between multiple factors[20]. Scenario analysis, while useful for exploring different possibilities, does not provide probabilities for the occurrence of each scenario, limiting its predictive power[21]. Probabilistic modeling, although more sophisticated, can be computationally intensive and may still rely on uncertain input data, compounding the uncertainty rather than clarifying it[22]. As a tool for probabilistic modeling, the Monte Carlo (MC) simulation is commonly employed to conduct error propagation in model parameters[23]. Traditional MC simulation methods often face limitations in handling parameter uncertainties due to their reliance on frequentist probability distributions, which may not adequately represent real-world variability[24].
Collectively, research issues that motivated the present study were: (1) accurately evaluating the environmental impacts of EV battery production and (2) mitigating inconsistencies by quantifying uncertainties in the assessment of EV battery production. To address these problems, this study performed an LCA of LFP batteries under multiple uncertainties[25-27]. The system boundary was defined as "cradle-to-gate", specifically, the battery production process. The foreground data stem from multiple relevant literature on LFP production and the background data was rooted in Ecoinvent 3.0[28,29]. Then, the ReCiPe method was adopted and 11 environmental indicators were selected. Additionally, Bayesian inference was incorporated into the MC simulation, also referred to as the Bayesian Monte Carlo (BMC) method, for quantifying the LCI uncertainties of the LFP production process. This leverages prior information and updates our uncertainty estimates as more data becomes available, leading to more robust and accurate quantifications of uncertainties. Further, a local sensitivity analysis was conducted to determine environmentally sensitive parameters. The uncertainty analytics in this study not only reveals the variation of environmental impacts of LFP battery production but also enhances the robustness and reliability of assessment.
The rest of this article is organized as follows: Part 2 presents the methodology of LCA employed in this study. Part 3 describes the final numerical results. Part 4 provides critical discussions, and Part 5 concludes this study.
METHODS
LCA is a systematic and comprehensive methodology employed to evaluate the environmental impacts associated with a product, process, or system throughout its life cycle. In this study, the environmental assessment of one LFP battery pack is conducted using LCA methodology, following the guidelines of the International Organization for Standardization (ISO) 14040 (2006a) and ISO 1404 (2006b)[8,30].
Goal and scope definition
Goal of this study
The research object in this study is an LFP battery pack. According to Gaines et al.[31] and Ellingsen et al.[32], a single battery pack comprises several distinct components, including battery modules, a battery management system (BMS), a cooling system, and battery packaging. Within this framework, battery cells are consolidated into individual modules. Figure 1 illustrates the fundamental model of the battery pack, showcasing the pack level to modules and further down to individual cells. The goal of this study is to ascertain LCI data pertaining to an LFP battery, assess the environmental implications of LFP batteries within the manufacturing life cycle, and examine the influence of uncertainties in key parameters on environmental indicators for further environmental performance improvement. Meanwhile, these analysis results will furnish support for investigating the environmental impact of the subsequent use and recycling of LFP batteries in different scenarios.
Scope of this study
As a medium for energy storage and transfer, LFP battery plays a fundamental role in the power supply for EVs. Scrutinizing 241 EVs in China, Wang and Yu[33] found that the average weight of LiBs for these EVs approximates 298 kg. It is assumed that the average weight of the LFP battery pack in this study is 300 kg. Table 1 specifies the component ratios within a battery pack.
Battery pack configurations
Components | Mass (%) |
Battery cell | 60.1 |
Battery cooling system | 4.1 |
BMS | 3.7 |
Battery packaging | 32.1 |
The functional unit (FU) serves as the basis for the calculation of every intake of raw materials, energy consumption, waste gas produced, and effluent emitted during the battery production process. The FU in this study is defined as "producing one complete LFP battery pack, with a weight of 300 kg, designed for an electric vehicle". As shown in Figure 2, the system boundary is in the form of “cradle-to-gate”, primarily covering the production stages of LFP batteries and including material preparation, transportation, assembly, manufacturing, and package phases. The LFP battery can be classified into eight main components, namely the positive electrode, negative electrode, separator, cell container, electrolyte, BMS, battery cooling system, and battery package. Of these main components, the manufacturing processes of four critical ones are depicted in Figure 3. The energy consumption, heat generation, transportation, atmospheric emissions, and effluents occurring within the system boundary were primarily considered, while the usage and end-of-life disposal phases are ignored due to the issue of data availability in practical situations.
Data cutoff criteria and data collection
In this study, foreground data are the primary concerns on the production of LFP batteries, encompassing information gathered from diverse literature databases. The background data for raw material extraction, transportation, assembly, and manufacturing processes stem from the Ecoinvent 3.0 database. A 0% cutoff criterion was employed in the LCA practice, allowing for a comprehensive consideration of all relevant factors in the assessment. For the foreground data on specific materials missing in the LCA databases, data from similar materials were directly employed for imputation. Specific inventory information regarding the material substitution is detailed in the following chapter.
Life cycle inventory analysis
The LCI of the complete LFP battery pack was compiled in conjunction with data from various studies. Primary data on raw materials during the production of individual battery cells stemmed from the study of Notter et al.[34]. Energy consumption during assembly was obtained from Baars et al.[35], while inventories for battery packaging, cooling system, and the BMS were sourced from the research by Ellingsen et al.[32] and Crenna et al.[4]. The main components of these parts are detailed in Tables 1 and 2. Additionally, the Supplementary Materials provides a detailed summary of these tables, outlining the inventory for battery masses and the sources of the data. Our inventory employed Ecoinvent 3.0 as a background system.
Battery cell configurations
Components | Mass (%) |
Cathode electrode paste | 22.8 |
Cathode current collector | 2.9 |
Anode electrode paste | 9.9 |
Anode current collector | 13.3 |
Separator | 1.3 |
Electrolyte | 9.5 |
Battery cell container | 0.4 |
The cathode, a critical component of the LFP battery, consists of the positive electrode paste and the cathode current collector. The positive electrode paste is made from LFP as the primary material, with Polyvinylidene Fluoride (PVDF) as the binder, carbon black as the conductive agent, and N-methyl-2-pyrrolidinone (NMP) as the solvent to impart a slurry texture to the mixture. The cathode current collector is primarily composed of aluminum foil, supplemented with a pre-treatment process using sulfuric acid
Similar to the cathode, the anode comprises the negative electrode paste and anode current collector. The negative electrode paste uses carboxymethyl cellulose and styrene-butadiene rubber (SBR) as binders, graphite as the conductive carbon, and NMP as the solvent to provide a slurry texture to the mixture. Notably, non-metallic graphite serves as the primary component, exhibiting thermal and electrical conductivity akin to metals. The production of SBR, modeled using the inventory suggested by Peters et al.[38], includes materials such as butadiene, styrene, soap, deionized water, cyclohexane, and sodium persulfate. The separator inventory, sourced from the work by Notter et al.[34], includes polyethylene, phthalic anhydride, hexafluoroethane (C3F6), polyvinyl fluoride, and silica sand, with C2F6 replacing C3F6 in some cases. The electrolyte commonly comprises a lithium salt dissolved in an organic solvent and includes additives[39]. Building on the study by Baars et al.[35], the model employs lithium hexafluorophosphate (LiFP6) as the conductive salt, with ethylene carbonate (EC) and dimethyl carbonate (DMC) as solvents, and vinyl carbonate (VC) as an additive. The inventory data on these solvents and additives are available in the Ecoinvent 3 database.
The cell container's composition involves materials such as polyethylene terephthalate (PET), aluminum, polypropylene, and copper[4]. Existing LCA studies[40] on the BMS were performed in various degrees. In this study, the environmental inventory of BMS was grounded in the Ecoinvent 3 framework, encompassing the integrated battery interface system (IBIS), high-voltage system, low-voltage system, battery module boards (BMB), and electronic components. The BMB and fasteners in BMS were approximated by printed wiring boards and low-alloyed steel in the Ecoivent database, respectively. The cooling system of the battery pack is composed of a radiator, manifolds, clamps and fasteners, pipe fittings, a thermal pad, and coolant. Among these six sub-components, the radiator stands out as the most critical element, being constructed from aluminum metal. The environmental profile of the cooling system has been investigated by Ellingsen et al.[32].
Battery packaging is divided into three parts: module packaging, battery tray, and battery retention, with inventory data sourced from the work by Ellingsen et al.[32]. One battery pack comprises 12 modules, and one module consists of 30 cells. Each cell is encased in a protective cassette, featuring an outer and inner frame, with the outer frame made from nylon 66, and the inner frame is similarly constructed. The assembly needs steel fasteners (retention rods, bolts, nuts), nylon washers produced via injection molding, and busbars welded to cell tabs, all under a protective ABS plastic lid. The heat transfer plate is crafted from anodized aluminum. Bimetallic busbars, with 30% aluminum and 70% copper composition, and washers feature both aluminum and copper sides. Module components such as the busbar holder and end-busbar holder are made from ABS plastic through injection molding.
Within the framework of the model, the assembly phase encompasses the integration of anode material, cathode material, battery cells, battery modules, the battery cooling system, battery packaging, and the ultimate assembly of the battery pack itself. The energy consumption required for the assembly process is derived from the Ecoinvent 3 database and has been estimated based on data provided by Ellingsen et al.[32].
Analyzing the "cradle-to-gate" of EV batteries allows the identification of environmental hotspots within the production phase, which consistently constitutes the largest proportion of the overall environmental footprint. This approach facilitates early mitigation of resource usage, emissions, and environmental impacts during the product's life cycle[41,42]. Thus, transportation considerations are limited to the conveyance of raw materials to chemical plants and to factories where related components are manufactured, as well as the transportation of materials and prefabricated components to the battery production facilities. Given China's significant role in the production of raw materials for lithium batteries[43], it was assumed that the manufacturing phase occurs within the country. Consequently, the China Statistical Yearbook 2023 (Yearbook 2023) was referenced based on the assumptions made by the National Bureau of Statistics of China (NBSC)[44]. It was assumed that the raw materials and components are typically transported at an average distance of 180 km using diesel trucks with a capacity of over 32 metric tons.
The foreground data (data identified in the LFP battery manufacturing process) and background data (corresponding data in the LCA database) are integrated to prepare the LCIs in Table 3 based on the processes and the components of each part within the system boundary described above.
Main resource consumption and emissions in the life cycle of LFP battery manufacturing
Pollution type | Resources/emissions | unit | LFP battery manufacturing |
Resource consumption | Coal | kg | 177 |
Natural gas | m3 | 382 | |
Oil | kg | 178 | |
Water emission | Nitrate | kg | 2.26 |
Phosphate | kg | 9.78 | |
Atmospheric emission | CO2 | kg | 3154.67 |
CH4 | kg | 0.40 | |
N2O | kg | 0.12 |
Life cycle impact assessment
LCIA evaluates potential environmental impacts and resource consumption highlighted by an LCI. According to the ISO standards, impact category selection, classification, and characterization are three mandatory stages, while the normalization, grouping, and weighting steps are optional within the framework[29]. The ReCiPe midpoint (H) model[45] was employed to evaluate the environmental footprint of battery production. LCIA in this study covered a total of 11 impact categories, including global warming potential (GWP), ozone depletion potential (ODP), ozone formation potential (OFP), fine particulate matter formation (FPMF), acidification potential (AP), freshwater eutrophication (EP), marine eutrophication (EP), marine ecotoxicity (ME), urban land use (LU), fossil resource scarcity (FRS), and water consumption (WC). The excerpt of characterization factors related to these environmental impact categories is listed in Table 4.
Characterization factors of resources and emissions (excerpt)
Impact category | Resources/Emissions | Characterization factor | Unit |
Global Warming Potential | CO2 | 1 | kg CO2-eq/kg |
CH4 | 34 | kg CO2-eq/kg | |
CHCl3 | 20 | kg CO2-eq/kg | |
N2O | 298 | kg CO2-eq/kg | |
Ozone depletion potential | CFC-11 | 1 | kg CFC-11 -eq/kg |
Halon-1301 | 14.066 | kg CFC-11 -eq/kg | |
CCl4 | 0.895 | kg CFC-11 -eq/kg | |
Ozone formation potential | NOx | 1 | kg NOx-eq/kg |
NMVOC | 0.18 | kg NOx-eq/kg | |
Fine particulate matter formation | SO2 | 0.29 | kg PM2.5-eq/kg |
PM2.5 | 1 | kg PM2.5-eq/kg | |
Acidification potential | NOx | 0.36 | kg SO2-eq/kg |
NH3 | 1.96 | kg SO2-eq/kg | |
SO2 | 1.00 | kg SO2-eq/kg | |
Freshwater eutrophication | Phosphorus | 1 | kg P-eq/kg |
Phosphate | 0.33 | kg P-eq/kg | |
Marine ecotoxicity | 1,4-Dichlorobenzene | 1 | kg 1,4-DCB -eq/kg |
Nickel | 32 | kg 1,4-DCB -eq/kg | |
Water consumption | CF watershed median | 6.04E-13 | species·yr/m3 |
CF area-weighted country average | 1.74E-12 | species·yr/m3 | |
Fossil resource scarcity | Crude oil | 0.457 | kg USD2013/kg |
Hard coal | 0.034 | kg USD2013/kg | |
Natural gas | 0.301 | kg USD2013/kg |
BMC-based uncertainty analysis
This study employs the BMC method to conduct a robust uncertainty analysis for the LCA of LFP battery production[46]. The methodological framework [Figure 4] begins with defining a deterministic LCA model. To capture the inherent uncertainties in the LCA, a sensitivity analysis was performed to identify critical uncertain parameters.
Figure 4. BMC-based uncertainty analysis for LCA of LFP battery production. BMC: Bayesian Monte Carlo; LCA: Life cycle assessment; LFP: Lithium iron phosphate.
The prior probability distributions of these parameters were determined based on literature and expert judgment. An initial round of MC simulations is then conducted using these prior distributions, transforming the deterministic LCA model into a probabilistic one and confidence intervals. This could provide quantitative uncertainty of the environmental impact results. The subsequent phase involves Bayesian updating, wherein observed data is used to update the prior distributions. This process integrates observed data with prior distributions through Bayesian inference to produce posterior distributions, reflecting refined uncertainty estimates. For a practical implementation, the integral evaluated is:
where x is the material input, f(x) is the function representing the input parameter and p(x) is the probability density function (PDF) of x. BMC views the integral as a Bayesian inference problem, where
where K is the covariance matrix defined by a covariance function, such as:
with hyperparameters w0 and wd. The posterior distribution over the function values, given the observed data, is also Gaussian. The posterior mean and covariance are:
where k(X, x') is the covariance between new data points and observed data. The BMC estimate of the integral
where
COMPUTATIONAL RESULTS AND DISCUSSION
Results
The LCA results for the production of the four major components comprising the battery pack across eleven environmental impact categories are detailed in Table 5. In this study, environmental indicators such as GWP, ODP, and OFP were primarily considered to present the main results of the environmental impacts. As indicated, the resources and energy consumption during the cradle-to-gate LCA of the LFP battery are predominantly attributed to the production of battery cells, which consume the highest number of resources within a battery pack. Therefore, all the environmental impact categories associated with battery cell production significantly surpass those of other components’ production, accounting for more than 54% of the total value, respectively. Thus, Figure 5 illustrates the environmental impact of the more detailed breakdown of battery component production within a battery pack. The primary contributors to the total environmental impacts, as shown in Figure 5, are the cathode electrode paste production, which dominates GWP, ODP, OFP, EP, FRS, and WC (29.67%, 21.56%, 26.64%, 65.88%, 28.94%, and 36.53%, respectively). This is followed by the battery packaging, BMS, anode current collector, and anode electrode paste, which contribute in descending order to these impacts. For AP and EP, the anode current collector manufacturing has the highest impact (48.39% and 28.22%, respectively), followed by the cathode electrode paste, BMS, battery packaging, and other cell assembly processes.
LCA results for per LFP battery pack [ReCiPe Midpoint (H)/World Recipe H]
Impact category | Unit | Battery cell | Battery cooling system | BMS | Battery packaging | Total |
Global warming potential | kg CO2 eq | 1.86E+03 | 1.77E+02 | 7.89E+02 | 8.54E+02 | 3.68E+03 |
Ozone depletion potential | kg CFC-11 eq | 8.04E-04 | 4.37E-05 | 4.06E-04 | 3.40E-04 | 1.59E-03 |
Ozone formation potential | kg NOx eq | 6.35E+00 | 4.59E-01 | 2.72E+00 | 2.13E+00 | 1.17E+01 |
Fine particulate matter formation | kg PM2.5 eq | 8.81E+00 | 3.52E-01 | 2.30E+00 | 1.80E+00 | 1.33E + 01 |
Acidification potential | kg SO2 eq | 2.50E+01 | 7.87E-01 | 5.22E+00 | 4.23E+00 | 3.52E+ 01 |
Freshwater eutrophication | kg P eq | 2.58E+00 | 7.42E-02 | 1.20E+00 | 4.17E-01 | 4.27E+00 |
Marine eutrophication | kg N eq | 3.71E-01 | 4.26E-03 | 4.30E-02 | 5.56E-02 | 4.74E-01 |
Marine ecotoxicity | kg 1,4-DCB | 1.39E+03 | 1.81E+01 | 7.42E+02 | 1.45E+02 | 2.30E+03 |
Land use | m2a crop eq | 1.03E+02 | 2.49E+00 | 4.56E+01 | 1.72E+01 | 1.68E+02 |
Fossil resource scarcity | kg oil eq | 5.16E+02 | 3.86E+01 | 1.97E+02 | 2.11E+02 | 9.63E+02 |
Water consumption | m3 | 4.36E+01 | 2.38E+00 | 8.10E+00 | 8.81E+00 | 6.29E+01 |
Overall, the cathode electrode paste energy requirements are the biggest contributor to the impacts of GWP, ODP, marine eutrophication, Photochemical Ozone Creation Potential (POCP), water depletion, and fossil depletion, whereas the anode current collector is the biggest contributor to the impacts of AP, EP, Particulate matter formation, Human Toxicity Potential (HTP), freshwater ecotoxicity, ME, LU and metal depletion [Figure 5].
Since the environmental burdens of LFP battery production are largely driven by energy consumption[42], the energy consumption during the production procedure is further quantified. Figure 6 shows the cradle-to-gate energy consumption data expressed in mega joules (MJ) per production step, wherein nearly 40,800 MJ of energy is utilized in the manufacturing process of an LFP battery pack. The highest electricity consumption occurs during the production of cathode electrode paste, accounting for approximately 11,822 MJ, followed by the manufacturing of the BMS at approximately 8,387 MJ, and battery packaging at approximately 9,065 MJ. This characteristic of the LCA findings is consistent with observations made in other EV battery analyses[4]. Conversely, the battery container and separator production processes require the least amount of energy.
Sensitivity analysis and uncertainty analysis
To exemplify the uncertainty analysis conducted on the environmental impacts of LFP battery production, the MC simulation results for GWP were focused on. Figure 7 presents the distribution of GWP and closely resembles a Gaussian distribution. Statistical examination reveals that both the mean and median values stand at around 3,675 kg and 3,662 kg CO2 eq. per LFP battery pack, respectively, with negligible deviation from the deterministic LCA final value. The standard deviation is approximately 194 kg CO2 eq., with a coefficient of variability of 5.27%. The 95% confidence interval spans from 3,338 to 4,092 kg CO2 eq. per battery pack, as depicted by red bars in the visualization. Additionally, the box plot presents percentiles at 2.5%, 25%, 75%, and 97.5%. Further statistical details are provided in Table 6.
Figure 7. Output distribution of 10,000 MC runs for GWP; top diagram: box plot diagram; bottom diagram: histogram with red bars that indicate the 95% confidence interval. MC: Monte Carlo; GWP: Global warming potential.
Statistical evaluation of GWP impacts distribution
Values | Unit | |
Trials | 10000 | - |
Base case | 3682.03 | kg CO2 eq |
Mean | 3675.68 | kg CO2 eq |
Median | 3661.69 | kg CO2 eq |
Standard deviation | 193.86 | kg CO2 eq |
2.5% percentile | 3338.04 | kg CO2 eq |
97.5% percentile | 4091.80 | kg CO2 eq |
Coefficient of variability | 5.27 | % |
Minimum | 3016.40 | kg CO2 eq |
Maximum | 4705.72 | kg CO2 eq |
To further identify key substances affecting the GWP, we employed a stepwise tracing approach to identify key material parameters generating environmental impacts. In this study, the sensitivity analysis was carried out by changing ± 5% material input, and the results are shown in Figure 8.
Figure 8. Sensitivity analysis of GWP impacts of (A) Battery pack; (B) Cathode electrode paste; (C) LiFP4; (D) Cathode current collector; (E) Battery cooling system; (F) Battery cooling thermal pad; (G) Battery cooling manifolds with respective parameters. GWP: Global warming potential; LiFP4: Lithium iron phosphate.
Figure 8A highlights significant variations in GWP impacts during the battery pack manufacturing process, particularly in the fabrication of the cathode electrode paste, cathode current collector, and the battery cooling system. The respective GWP changes for these components range from -17.62% to -14.15%, -25.63% to 1.62%, and -14.41% to 8.87%. A focused analysis reveals that in the production of cathode electrode paste, the materials PVDF and LiFP4 exhibit notable GWP shifts of -15.70% to 15.7% and -13.16% to 13.16%, as detailed in Figure 8B). Further scrutiny of LiFP4 production material impacts reveals a notable influence from LiOH, with GWP variations of -14.90% to 14.9%, as detailed in Figure 8C. Similarly, the manufacturing of the cathode current collector shows significant GWP impacts from aluminum and NaOH with changes of -13.90% to 13.9% and -3.49% to -6.51%, as presented in Figure 8D. Further examination in Figure 8E indicates that during the battery cooling system manufacturing, the thermal pads and cooling manifolds lead to GWP shifts of -28.08% to 28.08% and -19.92% to 19.92%, respectively. The analysis extends to the specific materials used in the production of these components. For instance, silicon in the thermal pad manufacturing processes emerges as the most impactful material, with a GWP change range from -78.40% to 0.82%, as shown in Figure 8F. Moreover, aluminum used in the cooling manifold fabrications maintains a consistent GWP influence of -13.90% to 13.9%, as illustrated in Figure 8G. This analysis underscores the substantial GWP impacts of specific LFP EV battery manufacturing materials and processes. It becomes evident that key variables such as PVDF, LiOH, aluminum, and silicon play critical roles in shaping the environmental footprint of battery pack production.
In this study, the prior distributions used for estimating environmental impact in LCA were based on the Global EV Outlook provided by International Energy Agency (IEA)[2] and were subsequently updated using the likelihood distributions resulting from the results of the MC analysis. Table 7 shows the mean values and coefficients of variation (CV) for both the prior and refined (posterior) parameter distributions relevant to the inventory and impact assessment phases. The reduced CV values in the posterior distributions demonstrate that incorporating more precise likelihood information effectively decreased uncertainty. For example, the CV of the posterior probability distribution for LiOH was reduced to less than a quarter of its initial value (0.021), and similar reductions were observed for aluminum and silicon. The posterior distributions of these three key parameters were significantly shaped by the likelihood distributions due to their higher precision compared to the initial distributions.
Summary of prior, likelihood, and posterior distributions for impact categories and the changes in CV values
Parameters | Prior | Likelihood | Posterior | %change in CV | |||
Mean | CV | Mean | CV | Mean | CV | ||
LiOH | 30 | 0.096 | 24.34 | 0.0222 | 24.53 | 0.021 | -78.13% |
Aluminum | 8 | 0.125 | 1.1 | 0.019 | 1.105 | 0.019 | -84.8% |
Silicon | 0.2 | 2.236 | 0.22 | 0.18 | 0.22 | 0.18 | -91.95% |
Figure 9 illustrates the comparison of the prior and posterior probability density distribution (PDFs) for LiOH [Figure 9A], aluminum [Figure 9B], and silicon [Figure 9C]. It is shown that the posterior distribution is narrower than the prior distribution. The uncertainty associated with the parameters had been reduced due to the incorporation of more accurate observed data.
Figure 9. Comparison of prior and posterior distributions of the mass of (A) LiOH; (B) Aluminium; and (C) Silicon. LiOH: Lithium hydroxide.
Figure 10 presents the PDFs of the GWP in both prior and posterior distributions, represented by the blue and yellow lines, respectively. The mean, 2.5%, and 97.5% percentile values show minimal differences between the prior and posterior distributions. However, the posterior distribution is narrower, with a smaller standard deviation. Incorporating observed data into the prior probability distribution in this study effectively reduces uncertainty, enhancing the reliability of the GWP estimations.
Discussion
In this study, we analyzed the environmental impacts associated with LFP EV battery production, placing particular emphasis on data precision and inventory reliability. The findings reveal that the cathode electrode paste and anode current collector emerge as the primary contributors to emissions and resource utilization, primarily due to energy demands in battery cell manufacturing. This energy-intensive production phase accounts for 58.99% and 12.91% of total energy use, respectively, underscoring the CO2 reduction potential through optimizations in these components. Our result aligns with previous literature on LIBs, which similarly highlights energy consumption in cell manufacturing as a substantial factor in overall emissions[47].
For sensitivity analysis, key materials influencing the GWP were identified. Specifically, PVDF, LiOH, aluminum, and silicon demonstrated significant influence over GWP. PVDF and LiFePO4, integral to cathode paste, showed notable GWP variability, with LiOH prominently affecting GWP in LiFePO4 production. Aluminum’s impact spans cathode collectors and cooling manifold components, while silicon significantly contributes to the GWP variation within battery thermal pads. These findings corroborate previous environmental impact assessments and further elucidate the roles of specific materials in shaping the environmental footprint of battery production.
Moreover, the BMC method, applied to evaluate uncertainty, effectively reduced the CV values for GWP, validating its practicality for handling uncertainties in LCA studies. However, this analysis encountered limitations due to missing data, which constrained correlation assessments among input parameters. Future studies may benefit from physical process models that incorporate variable interactions, thereby allowing for enhanced assumptions in uncertainty analysis and improving the LCA's robustness and accuracy for LFP EV batterie[48,49].
CONCLUSIONS
In this study, a BMC-based uncertainty analysis method for LCA of LFP battery production was developed. The results show that the production of battery cells has the largest environmental impact. Consistent with the energy consumption analysis of the various components of LFP batteries, the production of the cathode electrode paste consumes the most electricity and exerts the greatest environmental impact. Sensitivity analysis reveals that the GWP is most sensitive to the production of the cathode electrode paste, the cathode current collector, and the battery cooling system. The key parameters influencing GWP are identified as LiOH, aluminum, and silicon. By incorporating observational data for these key parameters into the corresponding prior distributions, the CV for their posterior distributions was significantly reduced. As a result, the posterior distribution of GWP became notably narrower compared to the prior distribution, enhancing the overall reliability of the LCA results.
However, the limitation of this paper is that the potential for recycling and reuse of several raw materials used in LFP batteries was not considered. Follow-up studies can extend this “cradle-to-gate” case to “cradle-to-grave” assessment that incorporates the usage and end-of-life stages of an LFP battery pack. The results of this LCA could benefit LFP battery manufacturers by pinpointing the hotspots to mitigate environmental impacts across diverse manufacturing processes. Additionally, it is important to note that other types of uncertainty need to be considered, such as the scenario uncertainties that include market demand, fluctuations in raw material prices, changes in policies and regulations, and variations in recycling rates to obtain relatively accurate environmental assessment results. The EV batteries are still constantly developing and evolving. Their environmental impact and cost assessment will remain subjects of great concern to support sustainable production.
DECLARATIONS
Author’s contributions
Conceptualization, methodology and experiments, writing-original draft, data collection: Gao B
Methodology and experiments: Yang S
Editing and funding: Peng S
Availability of data and materials
The data and materials are available from the corresponding author upon reasonable request.
Financial support and sponsorship
This study is financially supported by the Project of Guangdong Science and Technology Innovation Strategy (Grant number STKJ202209065).
Conflicts of interest
Peng S is the Guest Editor of the Special Issue “Development of Green Manufacturing Technology for Batteries” of the journal of Green Manufacturing Open. The other authors declared that there are no conflicts of interest.
Ethical approval and consent to participate
Not applicable.
Consent for publication
Not applicable.
Copyright
© The Author(s) 2024.
Supplementary Materials
REFERENCES
1. Xia X, Li P. A review of the life cycle assessment of electric vehicles: Considering the influence of batteries. Sci Total Environ. 2022;814:152870.
2. IEA, 2023. World Energy Outlook 2023. IEA, Paris. Available from: https://www.iea.org/reports/world-energy-outlook-2023. [Last accessed on 11 Dec 2024].
3. Commission. Regulation (EU) 2023/1542 of the european parliament and of the council of 12 July 2023 concerning batteries and waste batteries, amending directive 2008/98/EC and regulation (EU) 2019/1020 and repealing directive 2006/66/EC (Text with EEA relevance). Off J Eur Union. ;2023:191:p.1-117. Available from: https://eur-lex.europa.eu/eli/reg/2023/1542/oj. [Last accessed on 11 Dec 2024]
4. Crenna E, Gauch M, Widmer R, Wäger P, Hischier R. Towards more flexibility and transparency in life cycle inventories for Lithium-ion batteries. Resour Conserv Recyc. 2021;170:105619.
5. Liu C, Cai W, Zhai M, Zhu G, Zhang C, Jiang Z. Decoupling of wastewater eco-environmental damage and China's economic development. Sci Total Environ. 2021;789:147980.
6. Temporelli A, Carvalho ML, Girardi P. Life cycle assessment of electric vehicle batteries: an overview of recent literature. Energies. 2020;13:2864.
7. Chen B, Xiong R, Li H, Sun Q, Yang J. Pathways for sustainable energy transition. J Clean Prod. 2019;228:1564-71.
8. ISO, 2006a. ISO 14040 international standard. In: environmental management-life cycle assessment-principles and framework. International organization for standardization, Geneva, Switzerland. Available from: https://www.iso.org/standard/37456.html. [Last accessed on 13 Dec 2024].
9. Hao H, Mu Z, Jiang S, Liu Z, Zhao F. GHG Emissions from the production of lithium-ion batteries for electric vehicles in China. Sustainability. 2017;9:504.
10. Shu X, Guo Y, Yang W, Wei K, Zhu G. Life-cycle assessment of the environmental impact of the batteries used in pure electric passenger cars. Energy Rep. 2021;7:2302-15.
11. Marques P, Garcia R, Kulay L, Freire F. Comparative life cycle assessment of lithium-ion batteries for electric vehicles addressing capacity fade. J Clean Prod. 2019;229:787-94.
12. Ellingsen LA, Singh B, Strømman AH. The size and range effect: lifecycle greenhouse gas emissions of electric vehicles. Environ Res Lett. 2016;11:054010.
13. Majeau-Bettez G, Hawkins TR, Strømman AH. Life cycle environmental assessment of lithium-ion and nickel metal hydride batteries for plug-in hybrid and battery electric vehicles. Environ Sci Technol. 2011;45:4548-54.
14. Liu Z, Islam F, Era IZ, Grandhi M. LCA-based environmental sustainability assessment of hybrid additive manufacturing of a turbine blade. Green Manuf Open. 2023;2:1.
15. Erakca M, Pinto Bautista S, Moghaddas S, et al. Closing gaps in LCA of lithium-ion batteries: LCA of lab-scale cell production with new primary data. J Clean Prod. 2023;384:135510.
16. Kim HC, Wallington TJ, Arsenault R, Bae C, Ahn S, Lee J. Cradle-to-gate emissions from a commercial electric vehicle li-ion battery: a comparative analysis. Environ Sci Technol. 2016;50:7715-22.
17. Fernández R. Stochastic analysis of future scenarios for battery electric vehicle deployment and the upgrade of the electricity generation system in Spain. J Clean Prod. 2021;316:128101.
18. Lai X, Meng Z, Wang S, et al. Global parametric sensitivity analysis of equivalent circuit model based on Sobol’ method for lithium-ion batteries in electric vehicles. J Clean Prod. 2021;294:126246.
19. Cox B, Mutel CL, Bauer C, Mendoza Beltran A, van Vuuren DP. Uncertain environmental footprint of current and future battery electric vehicles. Environ Sci Technol. 2018;52:4989-95.
20. Heijungs R, Kleijn R. Numerical approaches towards life cycle interpretation five examples. Int J LCA. ;6:141.
21. Huijbregts MA, Gilijamse W, Ragas AM, Reijnders L. Evaluating uncertainty in environmental life-cycle assessment. a case study comparing two insulation options for a Dutch one-family dwelling. Environ Sci Technol. 2003;37:2600-8.
23. Tong W, Koh WQ, Birgersson E, Mujumdar AS, Yap C. Correlating uncertainties of a lithium-ion battery - a monte carlo simulation: a monte carlo simulation of a lithium-ion battery model. Int J Energy Res. 2015;39:778-88.
24. Jones HE, Hickman M, Kasprzyk-Hordern B, Welton NJ, Baker DR, Ades AE. Illicit and pharmaceutical drug consumption estimated via wastewater analysis. part B: placing back-calculations in a formal statistical framework. Sci Total Environ. 2014;487:642-50.
26. Zhang W. Structure and performance of LiFePO4 cathode materials: a review. J Power Sources. 2011;196:2962-70.
27. International energy agency. Global EV Outlook 2023: Catching up with climate ambitions. (Global EV Outlook). Available from: https://www.oecd-ilibrary.org/energy/global-ev-outlook-2023_cbe724e8-en. [ Last accessed on 11 Dec 2024].
28. Wernet G, Bauer C, Steubing B, Reinhard J, Moreno-ruiz E, Weidema B. The ecoinvent database version 3 (part I): overview and methodology. Int J Life Cycle Assess. 2016;21:1218-30.
29. Steubing B, Wernet G, Reinhard J, Bauer C, Moreno-ruiz E. The ecoinvent database version 3 (part II): analyzing LCA results and comparison to version 2. Int J Life Cycle Assess. 2016;21:1269-81.
30. ISO, 2006b. ISO 14044 international standard. In: Environmental Management-life Cycle Assessment-requirements and Guidelines. International Organization for Standardization, Geneva, Switzerland. Available from: https://www.iso.org/standard/38498.html. [Last accessed on 13 Dec 2024].
31. Gaines L, Cuenca R. Costs of lithium-ion batteries for vehicles. In 2000 [cited 2024 Feb 28]. p. ANL/ESD-42, 761281. Available from: http://www.osti.gov/servlets/purl/761281-9hcCH0/webviewable/. [Last accessed on 11 Dec 2024].
32. Ellingsen LA, Majeau-bettez G, Singh B, Srivastava AK, Valøen LO, Strømman AH. Life cycle assessment of a lithium-ion battery vehicle pack. J Ind Ecol. 2014;18:113-24.
33. Wang S, Yu J. A comparative life cycle assessment on lithium-ion battery: Case study on electric vehicle battery in China considering battery evolution. Waste Manag Res. 2021;39:156-64.
34. Notter DA, Gauch M, Widmer R, et al. Contribution of li-ion batteries to the environmental impact of electric vehicles. Environ Sci Technol. 2010;44:6550-6.
35. Baars J, Cerdas F, Heidrich O. An integrated model to conduct multi-criteria technology assessments: the case of electric vehicle batteries. Environ Sci Technol. 2023;57:5056-67.
36. Peters JF, Weil M. Providing a common base for life cycle assessments of li-ion batteries. J Clean Prod. 2018;171:704-13.
37. Majeau-Bettez G, Strømman AH, Hertwich EG. Evaluation of process- and input-output-based life cycle inventory data with regard to truncation and aggregation issues. Environ Sci Technol. 2011;45:10170-7.
38. Peters J, Buchholz D, Passerini S, Weil M. Life cycle assessment of sodium-ion batteries. Energy Environ Sci. 2016;9:1744-51.
39. Armand M, Axmann P, Bresser D, et al. Lithium-ion batteries - current state of the art and anticipated developments. J Power Sources. 2020;479:228708.
40. Li S, Zhang C.
41. Quan J, Zhao S, Song D, Wang T, He W, Li G. Comparative life cycle assessment of LFP and NCM batteries including the secondary use and different recycling technologies. Sci Total Environ. 2022;819:153105.
42. Keshavarzmohammadian A, Cook SM, Milford JB. Cradle-to-gate environmental impacts of sulfur-based solid-state lithium batteries for electric vehicle applications. J Clean Prod. 2018;202:770-8.
43. Song J, Yan W, Cao H, et al. Material flow analysis on critical raw materials of lithium-ion batteries in China. J Clean Prod. 2019;215:570-81.
44. Compiled by national bureau of statistics of China. China statistical yearbook, 2023. Available from: https://www.stats.gov.cn/sj/ndsj/2023/indexch.htm. [Last accessed on 11 Dec 2024].
45. Huijbregts MAJ, Steinmann ZJN, Elshout PMF, et al. ReCiPe2016: a harmonised life cycle impact assessment method at midpoint and endpoint level. Int J Life Cycle Assess. 2017;22:138-47.
46. Rasmussen C E, Ghahramani Z.
47. Deng Y, Li J, Li T, Gao X, Yuan C. Life cycle assessment of lithium sulfur battery for electric vehicles. J Power Sources. 2017;343:284-95.
48. Igos E, Benetto E, Meyer R, Baustert P, Othoniel B. How to treat uncertainties in life cycle assessment studies? Int J Life Cycle Assess. 2019;24:794-807.
Cite This Article

How to Cite
Download Citation
Export Citation File:
Type of Import
Tips on Downloading Citation
Citation Manager File Format
Type of Import
Direct Import: When the Direct Import option is selected (the default state), a dialogue box will give you the option to Save or Open the downloaded citation data. Choosing Open will either launch your citation manager or give you a choice of applications with which to use the metadata. The Save option saves the file locally for later use.
Indirect Import: When the Indirect Import option is selected, the metadata is displayed and may be copied and pasted as needed.
About This Article
Special Issue
Copyright
Data & Comments
Data
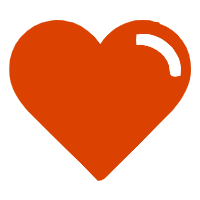
Comments
Comments must be written in English. Spam, offensive content, impersonation, and private information will not be permitted. If any comment is reported and identified as inappropriate content by OAE staff, the comment will be removed without notice. If you have any queries or need any help, please contact us at support@oaepublish.com.