Investigating the ability of CMIP6 HighResMIP models to simulate rainy season precipitation over the Lancang-Mekong River Basin
Abstract
The Coupled Model Intercomparison Project Phase 6 (CMIP6) has introduced the High-Resolution Model Intercomparison Project (HighResMIP) experiment, allowing unprecedented simulations and predictions of global climate models with high resolution (more than 50 km). This study evaluates the capability of six Global Climate Models (GCMs) of HighResMIP in reproducing rainy season precipitation in the Lancang-Mekong River Basin. (1) We selected mean precipitation, hourly precipitation frequency and hourly precipitation intensity during the multi-year rainy season as Precipitation Characteristic Indices to evaluate the ability of GCMs. We found that two GCMs, BCC-CSM2-HR and HadGEM3-GC31-HM, demonstrate a strong ability to reproduce the spatial distribution of precipitation indices during the rainy season, outperforming other GCMs and MME-M; (2) Most GCMs and multi-model ensemble mean (MME-M) can reproduce the latitudinal distribution of mean precipitation, hourly precipitation frequency and hourly precipitation intensity, except HadGEM3-GC31-HM (mean precipitation), HiRAM-SIT-LR and NICAM16-8S (hourly precipitation frequency and hourly precipitation intensity); (3) We found that most GCMs overestimate the frequency of light precipitation, which can reach 60%-80%, while the frequency in the ERA5 (the latest fifth generation reanalysis data product launched by the European Centre for Medium-Range Weather Forecasts) is 53.9%. However, almost all GCMs underestimate the frequency of moderate precipitation and heavy precipitation. For torrential precipitation, severe torrential precipitation, and extremely torrential precipitation events, most GCMs overestimate their frequency; and (4) Most GCMs can not simulate hourly precipitation well according to the Taylor diagram, because the correlation coefficients of four GCMs are about 0.1 and their normalized standard deviations are greater than 1. However, EC-Earth3P-HR and MRI-AGCM3-2-S demonstrate relatively better performance, with correlation coefficients of 0.42 and 0.36, and the normalized standard deviations are close to the reference. These findings will improve the understanding of GCM precipitation simulation with high spatial resolution and higher temporal resolution.
Keywords
INTRODUCTION
The Lancang-Mekong River Basin (LMRB) is a transnational river that connects six countries: China, Myanmar, Laos, Thailand, Cambodia and Vietnam. With its rich resources and advantageous geographical location, it is an important platform for regional consultation and joint construction under the Belt and Road Initiative[1]. The LMRB serves as a cornerstone for the socio-economic development of each country in the basin[2]. The spatial and temporal distribution of surface water resources and rainfall in the LMRB are extremely heterogeneous under the influence of monsoonal fluctuations in South and East Asia[3]. The LMRB is highly susceptible to extreme precipitation events, frequently resulting in disasters such as floods and droughts[4,5]. The frequency of floods in countries along the Mekong River Basin has shown an increasing trend since 1960 according to the statistics[6]. Although the timing and locations of flood occurrences vary among countries, floods constitute approximately 70% to 80% of the total number of natural disasters in the region[6]. Simultaneously, The Sixth Assessment Report of the Intergovernmental Panel on Climate Change (IPCC AR6) reports that the frequency and intensity of extreme precipitation events are increasing (high confidence) in the context of global climate change[7]. Moreover, Lutz et al. (2014)[8] suggest that increasing glacier melt and precipitation exacerbate the sensitivity to climate change in the upper LMRB, which originates on the Tibetan Plateau, affecting the composition of runoff and total runoff in the basin. Their study suggests that runoff in the LMRB will continue to increase until at least 2050, necessitating a shift in focus to addressing extreme events and intra-annual shifts in water availability. In conclusion, accurate estimation of the spatial and temporal characteristics of precipitation in the LMRB is of great significance for water resource management, flood early warning, agricultural production, ecological environmental protection, and climate change research.
Global Climate Models (GCMs) offer valuable insights into the simulated distribution of global precipitation. Conventional GCMs face limitations in capturing smaller-scale features, orographic effects, and interactions between scales. As a result, they are primarily applied for studying large-scale regions, such as the globe and hemispheres[9]. Furthermore, studies have demonstrated that GCMs excel in simulating temperature changes[10]. However, significant deviations persist in the simulation of regional precipitation, particularly in the context of small- and medium-scale precipitation changes[10,11]. The Coupled Model Intercomparison Project Phase (CMIP) initiated by the World Climate Research Program (WCRP) in 1995 has evolved to its sixth phase. The rapid development of high-performance computing resources is driving research into climate models with higher resolutions. These models can have a profound impact on the meteorological process of small- and medium-scale weather systems, resulting in a certain increase in simulation accuracy[12]. Some studies evaluate the historical precipitation simulation of CMIP5 and CMIP6 by comparing them with observed precipitation data[13-15]. Xin et al. (2020)[16] compared eight CMIP6 GCMs and their corresponding CMIP5 GCMs in simulating summer precipitation and the East Asian Summer Monsoon in China. They found that most CMIP6 GCMs performed better than the corresponding previous CMIP5 models with a larger correlation coefficient and smaller standard deviation, and the CMIP6 MME is more skillful than the CMIP5 MME. The reason is that all the CMIP6 models improve their ability to simulate the climatological pattern of the East Asian Summer Monsoon compared with the previous CMIP5 models. In summary, CMIP6 performs better in modeling precipitation compared to CMIP5. Previous studies have shown that improving the resolution of CMIP6 GCMs can enhance the authenticity of small- and medium-scale precipitation simulations[17,18]. Consequently, the CMIP6 has introduced the High-Resolution Model Intercomparison Project (HighResMIP) experiment, and there is a growing focus on investigating the capability of CMIP6 HighResMIP GCMs in capturing characteristics and changes in precipitation at smaller regional scales.
Recently, numerous studies have evaluated the performance of the CMIP6 GCMs in simulating precipitation at different resolutions, elucidating the reasons for bias and the resulting impacts. For instance, Muetzelfeldt et al. (2021) evaluate the performance of the Unified Model (UM) from the UK Meteorological Office (UKMO) in its global climate configuration, HadGEM3-GC3.1[9]. The evaluation focused on the simulation of the daily cycle of precipitation, along with frequency and intensity over Asia. They found that the high-resolution GCMs with convective parameterization improve simulations of the precipitation daily cycle at all spatial scales. These models also demonstrate a fairly accurate reproduction of the spatial pattern of precipitation in China compared to other regions in Asia. However, the interaction between airflow and topography exacerbates the existing bias in summer mean precipitation in high-resolution simulations. Yang et al. (2021) evaluated the precipitation simulation performance of 20 CMIP6 GCMs in China using the observed data from CN05.1[19]. They found that the CMIP6 models can better reproduce spatial distributions of precipitation and their interannual variability. Furthermore, the optimal model ensemble (BMME) outperforms the multimodel ensemble in simulating annual and winter precipitation, especially in regions with complex topography. However, there is no significant improvement in the simulation of summer precipitation. Xiao et al. (2022)[20] evaluated the performance of eight CMIP6 HighResMIP GCMs in simulating summer hourly precipitation and extreme precipitation over the Tibetan Plateau, comparing the results to TRMM 3B42 V7. The finding indicated an overestimation of summer precipitation over the Tibetan Plateau, primarily attributed to the overestimation of precipitation frequency. Huang et al. (2021) compare the high- and low-resolution simulation results of 12 CMIP6 GCMs based on the daily precipitation data from stations and satellite observations and the ERA5 precipitation data[21]. They mainly evaluate the performance of current climate models in simulating summer precipitation in the Southwest China region, especially the effect of model horizontal resolution on the simulation of extreme precipitation. They found that increasing the horizontal resolution can improve the simulation of summer precipitation intensity in steep terrain, but not in flat terrain, and the effect of the model parameterization scheme on the simulation of precipitation intensity needs to be further considered. Few studies have evaluated historical precipitation simulations of the CMIP6 HighResMIP GCMs on the LMRB. So far, we have found only one paper that compared the CMIP6 GCMs with their corresponding CMIP5 GCMs on the LMRB at a spatial resolution of 250 km and a monthly temporal resolution[22]. Therefore, it is necessary to study the performance of CMIP6 high-resolution GCM precipitation simulation on the LMRB.
Our study aims to evaluate the performance of precipitation simulation during the rainy season based on CMIP6 HighResMIP GCMs with high spatial resolution (50 km) and higher temporal resolution
MATERIALS AND METHODS
Study area
Figure 1 shows the geographical location of the LMRB. The Lancang-Mekong River originates from the Tanggula Mountains on the Tibetan Plateau, often referred to as the "Third Pole of the Earth", and flows through China, Myanmar, Laos, Thailand, Cambodia, and Vietnam before finally reaching the South China Sea. The entire length of the LMRB is approximately 4,880 km, with a total basin area of about 744,000 km2. The Upper reaches of the river in China are called the Lancang River, and The reaches flowing out of Yunnan is called the Mekong River. The LMRB belongs to the Pacific Ocean water system and is an important north-south transboundary water system in Asia, with a rich variety of climatic types, flowing through a variety of climatic zones ranging from frigid to tropical, dry-cold, dry-hot and humid-hot[25].
Figure 1. The Lancang-Mekong River Basin. The national boundaries in this figure are obtained from the Standard Map Service website of the Ministry of Natural Resources of the People's Republic of China with no modifications. The Map Content Approval Number is GS (2016) 1665.
The LMRB is shaped similarly to a strip, with the terrain gradually decreasing from northwest to southeast [Figure 1]. The elevation difference in the basin exceeds 6,000 m, which makes it rich in hydropower. However, the huge elevation difference also increases the difficulty of construction of national meteorological stations, making the region one of the more data-poor basins. Influenced by the westerlies and the Indian monsoon, the rainy season in the LMRB usually occurs between May and October, while the dry season is from November to April, and the runoff is mainly recharged by precipitation[26]. The upper reaches of the LMRB are cold with little rain, the middle reaches have distinct rainy and dry seasons, and the lower reaches are hot and humid. The distribution of annual precipitation is extremely uneven, with more than 85% of the precipitation concentrated in the rainy season due to the typical monsoon climate. The annual mean precipitation in the northern part of the LMRB is 400 mm, gradually increasing southward. The annual mean precipitation is more than 3,000 mm in the southern regions of Laos, Cambodia, and the mountainous areas on the edge of Vietnam[27].
Observation and reanalysis
The Global Grid Reanalysis Data (ERA5) was selected as the reference data to evaluate the performance of CMIP6 HighResMIP Models. ERA5 is the latest fifth generation reanalysis data product launched by the European Centre for Medium-Range Weather Forecasts (ECMWF), which has greatly improved in terms of temporal and spatial resolution and assimilation methods. Compared to the fourth generation reanalysis data product (ERA-Interim), the temporal and spatial resolution of ERA5 has been improved, with a horizontal resolution of 0.25° and a vertical resolution of 0.01 hPa (approximately 80 km). The temporal resolution has been increased from every 6 h to every 1 h, and the data time range has been extended to include real-time data from 1950 to the last five days[28]. In this paper, the ERA5 data spanning a time range of 1950-2014, totaling 65 years, is selected, with a temporal resolution of 1 h and a spatial resolution of
CMIP6 HighResMIP model data
HighResMIP is one of the sub-program experiments conducted by the CMIP6, which aimed to evaluate the impact of different model level resolutions on the fidelity of climate simulations[29]. The HighResMIP climate model is divided into three tiers for simulation experiments. The first tier (Tier-1) experiments are the historically forced Atmospheric Model Intercomparison Program (AMIP) simulation experiments from 1950-2014, and the second tier (Tier-2) experiments are the 100-year coupled (ocean-atmosphere coupled) simulation experiments from 1950-2050[30]. The third tier (Tier-3) experiments are an extension of the first tier for the years 2015-2050 (which can be further extended to 2100)[31]. Each tier experiment contains two sets of experiments: standard resolution and high resolution.
In this paper, the historical output of the CMIP6 HighResMIP Tier-1 high-resolution experiment is selected for analysis [Table 1]. The external forcing used in Tier-1 consists of aerosol loads of greenhouse gases including O3 and the 1950s ( 10-year mean) climate state, which is equivalent to the pre-industrial control of HighResMIP[29]. The boundary conditions are set as follows: the land use/land cover is kept unchanged (roughly the same as that of around 2000), and the surface's leaf area index (LAI) is its climatic state value characterized by seasonal variations[29]. The target high resolution of the Tier-1 experiment is 25-50 km, which is higher than the basic resolution of CMIP6 by 100 km and significantly higher than the basic resolution of CMIP5 model by 150 km. More detailed information on the experiment design can be found in Haarsma et al. (2016)[29].
Description of the six GCMs from CMIP6 HighResMIP used in the study area
Model | Resolution (latitude × longitude) | Modeling group | Reference |
BCC-CSM2-HR | 0.45° × 0.45° | Beijing Climate Center, China | Wu et al. (2021)[32] |
EC-Earth3P-HR | 0.35° × 0.35° | EC-Earth Consortium, Europe | Haarsma et al. (2020)[33] |
HadGEM3-GC31-HM | 0.23° × 0.35° | Met Office Hadley Centre, UK | Roberts et al. (2019)[34] |
HiRAM-SIT-LR | 0.50° × 0.50° | Research Center for Environmental Changes, Academia Sinica, Taiwan | Chen et al. (2023)[35] |
MRI-AGCM3-2-S | 0.19° × 0.19° | Meteorology Research Institute, Japan | Mizuta et al. (2012)[36] |
NICAM16-8S | 0.28° × 0.28° | JAMSTEC-AORI-R-CCS, Japan | Kodama et al. (2019)[37] |
Method
In order to facilitate the comparison between the GCMs from CMIP6 HighResMIP data and the observation data, this study used the bilinear interpolation method to interpolate all the data to the latitude and longitude grid of 0.5° × 0.5°. The multi-model ensemble averaging technique uses equal-weighted ensemble averaging, i.e., taking the arithmetic average of the simulation results of six models
In this paper, we selected mean precipitation, hourly precipitation frequency, and hourly precipitation intensity during the multi-year rainy season as Precipitation Characteristic Index to evaluate the ability of GCMs to simulate precipitation [Table 2]. The mean precipitation during the rainy season (units: mm d-1) is calculated as the total effective precipitation divided by the total number of days during the rainy season[20]. The performance of precipitation simulations can be evaluated through the examination of both hourly precipitation frequency and intensity. This dual analysis provides insights into the characteristics of precipitation bias (Xiao et al., 2022; Li et al., 2015)[20,40]. The hourly precipitation frequency (units: %) is defined as the percentage of the number of effective precipitation hours to the total number of hours throughout the study period[20]. The hourly precipitation intensity [units: mm (3h)-1] is the cumulative amount of effective precipitation divided by the number of effective precipitation hours[20]. In order to further understand how precipitation characteristics change with the altitude or elevation of GCMs and ERA5, we used the latitudinal distribution in mean precipitation, hourly precipitation frequency, and hourly precipitation intensity to show these changes. The latitudinal distribution is calculated by averaging the Precipitation Characteristic Index at the same latitude.
The selected Climatological Precipitation Index during the rainy season
Label | Description | Index definition | Units |
Pa | Mean precipitation during the rainy season | The total effective precipitation is divided by the total number of days during the rainy season | mm d-1 |
Pf | Hourly precipitation frequency | The percentage of effective precipitation hours to the total number of hours during the rainy season | % |
Pi | Hourly precipitation intensity | The cumulative amount of effective precipitation divided by the number of effective precipitation hours | mm (3h)-1 |
Fi | precipitation grade events-frequency | The percentage of precipitation hours in precipitation grade i to all effective precipitation hours | % |
Additionally, the relationship between precipitation frequency and intensity is an important indicator for evaluating the ability of GCMs to simulate precipitation (Li et al., 2015)[40]. Based on the grade of 12-h precipitation amounts specified in the People’s Republic of China Grade of precipitation National Standard (GB/T 28592-2012), we classified our 3-h precipitation intensity as follows: light precipitation
where Fi is the frequency for precipitation in grade i; N is the total number of grids; Tj is the total number of time steps at grid point j during the rainy season, and Iij is the indicator function for whether precipitation intensity falls into grade i at grid point j.
The indicator function Iij is 1 if the precipitation intensity falls into the precipitation grade i at grid point j during the rainy season, and otherwise the indicator function is 0.
Moreover, we use the Taylor diagram and Taylor Skill Score (TSS) to quantitatively evaluate the performance of GCMs and MME-M precipitation simulation during the rainy season over the LMRB (Taylor, 2001)[41]. Taylor diagram provides a concise statistical of correlation coefficients (R), root-mean-square difference (RMSD), and the ratio of standard deviation (RSD) between simulation and observation[42,43]. TSS is calculated by:
Where R is the correlation coefficient between the simulation and observation; R0 is the maximum possible R (typically set to 0.999); and σm and σo are the standard deviations of the simulations and observation, respectively. A closer TSS to 1 indicates that the simulation aligns well with the observation, whereas a TSS near 0 indicates that the simulation is opposite to the observation, and the simulation performance is poor.
RESULTS
The spatial distribution of climatological precipitation index during the rainy season
Figure 2 shows the observed and simulated spatial distribution of mean precipitation in the rainy season from 1950 to 2014 over the LMRB. The mean precipitation of ERA5 [Figure 2H] in rainy season showed significant spatial variability, which decreases gradually from south to north. Most areas in the lower LMRB experience mean precipitation higher than 6 mm d-1, with some regions reaching up to 12 mm d-1, while the mean precipitation is lower than 4 mm d-1 in the upper LMRB. The GCMs simulated the mean precipitation spatial pattern similar to ERA5 in rainy season with high mean precipitation in the lower LMRB and low mean precipitation in the upper LMRB, e.g., BCC-CSM2-HR [Figure 2A], EC-Earth3P-HR [Figure 2B] and HadGEM3-GC31-HM
Figure 2. Spatial distribution of mean precipitation in rainy season (unit: mm d-1) from 1950 to 2014 over the LMRB. (A-F) is six CMIP6 GCMs, (G) is the CMIP6 MME-M, and (H) is the ERA5 dataset.
Figure 3 shows the observed and simulated spatial distribution of hourly precipitation frequency in rainy season from 1950 to 2014 over the LMRB. The hourly precipitation frequency of ERA5 [Figure 3H] in rainy season also demonstrates the higher frequency in the lower LMRB. It ranges from 30% to 60%. The spatial distribution of hourly precipitation frequency of BCC-CSM2-HR [Figure 3A] and HadGEM3-GC31-HM
Figure 3. Spatial distribution of hourly precipitation frequency in rainy season (unit: %) from 1950 to 2014 over the LMRB. (A-F) is six CMIP6 GCMs, (G) is the CMIP6 MME-M, and (H) is the ERA-5 dataset.
Figure 4 shows the observed and simulated spatial distribution of hourly precipitation intensity in rainy season from 1950 to 2014 over the LMRB. The hourly precipitation intensity of ERA5 [Figure 4H] is higher than 1.5 mm (3h)-1 in most areas of the lower LMRB, and lower than 1.2 mm (3h)-1 in the upper LMRB. However, only a few GCMs are capable of accurately simulating the spatial distribution of hourly precipitation intensity during the rainy season. The simulation performance of BCC-CSM2-HR [Figure 4A] and HadGEM3-GC31-HM
Figure 4. Spatial distributions of hourly precipitation intensity [unit: mm (3h)-1] in rainy season from 1950 to 2014 over the LMRB. (A-F) is six CMIP6 GCMs, (G) is the CMIP6 MME-M, and (H) is the ERA-5 dataset.
The reasonableness of the simulated precipitation during rainy season depends on the accurate combination of precipitation frequency and precipitation intensity[20,40]. Therefore, we used hourly precipitation frequency and hourly precipitation intensity to reveal the bias characteristics of the CMIP6 HighResMIP GCMs. The HiRAM-SIT-LR and NICAM16-8S overestimated the precipitation intensity and underestimated the precipitation frequency in most areas of the LMRB (accounting for over 90% of the area). On the contrary, EC-Earth3P-HR and MRI-AGCM3-2-S underestimate the hourly precipitation intensity and overestimate the hourly precipitation frequency.
The latitudinal distribution of three precipitation indices during the rainy season
Figure 5 shows the latitudinal distribution of (a) mean precipitation, (b) hourly precipitation frequency, and (c) hourly precipitation intensity between the observed and simulated in rainy season from 1950 to 2014 over the LMRB. The maximum value of mean precipitation (3.3 mm d-1) in the rainy season of ERA5 (black line in Figure 5A) is located at 14.75° N with an altitude below 500 m. Additionally, the ERA5 mean precipitation during the rainy season decreases sharply above 20° N with increasing altitude. The simulating maximum value of mean precipitation (3.0-5.7 mm d-1) in the rainy season of GCMs and MME-M has similar characteristics to ERA5, which is also located at low latitudes of 14.75° N and lower altitude below 500 m. HadGEM3-GC31-HM (green line in Figure 5A) simulated the maximum value of mean precipitation is much higher than the ERA5 and other GCMs, which is 5.67 mm d-1. Similarly, the mean precipitation of GCMs during the rainy season also decreases sharply above 20° N with increasing altitude. The trend of ERA5 and GCMs for hourly precipitation frequency is similar to that for mean precipitation [Figure 5B]. The maximum hourly precipitation frequency of ERA5 and GCMs also mainly occurs at
Precipitation frequency distribution under different grades of precipitation
As shown in Figure 6, the precipitation frequency distribution under different grades of precipitation simulated by the CMIP6 HighResMIP GCMs is different from the ERA5. To more effectively demonstrate this distribution difference, in Figure 6A, we limited the grades of precipitation to torrential precipitation. We found that most GCMs overestimate the frequency of light precipitation [1.25 mm (3h)-1], which can reach 60%-80%, while the frequency in the ERA5 is 53.9%. However, almost all GCMs underestimate the frequency of moderate precipitation [3.75 mm (3h)-1] and heavy precipitation [7.5 mm (3h)-1]. The logarithmic coordinate [Figure 6B] can be used to enlarge the tail end of the precipitation grade events-frequency distribution. Most GCMs overestimate the precipitation frequency in simulating torrential precipitation [17.5 mm (3h)-1], severely torrential precipitation [35 mm (3h)-1], and extremely torrential precipitation events [more than 35 mm (3h)-1].
The performance of simulated precipitation during the rainy season
Figure 7 shows the Taylor diagram [Figure 7A] and TSS [Figure 7B] for the simulated precipitation in rainy season from 1950 to 2014 over the LMRB. EC-Earth3P-HR and MRI-AGCM3-2-S demonstrate relatively better performance for hourly precipitation, with correlation coefficients of 0.42 and 0.36, and the normalized standard deviations are close to the reference (REF). However, most GCMs can not simulate hourly precipitation well, because the correlation coefficients of foue GCMs are about 0.1 and their normalized standard deviations are greater than 1. The correlation coefficient of MME-M is about 0.3, while the Normalized standard deviation is far from the REF. TSS results are further consistent with the results of Taylor diagram; EC-Earth3P-HR and MRI-AGCM3-2-S perform better than other GCMs and MME-M.
DISCUSSION
This research discussion was divided into two parts. First, we discussed the similarities and differences between our simulated climatological precipitation results and other results. Additionally, the limitations of this study and future research directions are summarized.
The similarities and differences between simulated precipitation
In comparison with other studies, our findings exhibit both similarities and differences. The spatial pattern of CMIP6 HighResMIP GCM mean precipitation is high in the lower LMRB and low in the upper LMRB, which is similar to that of ERA5 during rainy season. The wet bias of precipitation was not found in the upper LMRB [Figure 2], which is confirmed by previous studies that CMIP6 HighResMIP GCMs simulations significantly reduce the wet bias of precipitation in regions, such as the east of the Tibetan Plateau and the Hengduan Mountains[43,44]. However, three CMIP6 HighResMIP GCMs, HiRAM-SIT-LR, MRI-AGCM3-2-S and NICAM16-8S, exhibit lower mean precipitation than that of ERA5 in the lower LMRB. The bias characteristics of the CMIP6 HighResMIP GCMs are further revealed by hourly precipitation frequency and hourly precipitation intensity. EC-Earth3P-HR and MRI-AGCM3-2-S suffer from the issue of "low intensity with high frequency", which is a common problem among CMIP6 HighResMIP GCMs in numerical simulations[20]. However, HiRAM-SIT-LR and NICAM16-8S overestimated the precipitation intensity and underestimated the precipitation frequency, resulting in an abnormal "high intensity with low frequency" problem.
Our analysis shows that the maximum value and its location of mean precipitation and hourly precipitation frequency in MME-M over the LMRB align with those in ERA5 from the latitudinal distribution. This result contrasts with the findings of Xiao et al. (2022)[20], who reported that hourly precipitation frequency in MME-M over the southern margin and the eastern part of the Tibet Plateau is generally higher than that of Tropical Rainfall Measuring Mission (TRMM). We also found that hourly precipitation intensity in
We further categorize precipitation intensity into light precipitation, moderate precipitation, heavy precipitation, torrential precipitation, severe torrential precipitation, and extreme torrential precipitation, based on the grade of 12-h precipitation amounts specified in the the Grade of Precipitation National Standard. However, Xiao et al. (2022) only used 1 mm/h intervals to classify precipitation intensity and did not study the frequency distribution across different grades of precipitation[20]. Most GCMs overestimate the frequency of light precipitation and underestimate the frequency of moderate precipitation and heavy precipitation than ERA5 does in our study, which is consistent with the findings of Xiao et al. (2022) over the eastern part of the Tibet Plateau[20]. Moreover, we found that most GCMs overestimate the frequency of torrential, severe torrential, and extreme torrential precipitation events, which contrasts with the conclusions of Xiao et al. (2022)[20].
Previous results show the good performance of most GCMs in simulating daily or monthly precipitation[22,43], but in our study most GCMs struggle to effectively simulate hourly precipitation. Therefore, most GCMs in the HighResMIP protocol should continue optimizing convective parameters at the hourly scale and improving the governing equations for atmospheric moisture transport and condensation in their next generation products to enhance the accuracy of precipitation simulations with higher temporal and spatial resolution[45].
Limitations
The limitations in selecting the number of HighResMIP GCMs
The six HighResMIP GCMs we chosen are from the experiments in Tier 1, which require the modeling groups to provide precipitation simulations at a 3-h temporal resolution. Nine GCMs can provide 3-h precipitation simulations in CMIP6 HighResMIP. We ultimately selected BCC-CSM2-HR, HadGEM3-GC31-HM, EC-Earth3P-HR, HiRAM-SIT-LR, MRI-AGCM3-2-S, and NICAM16-8S because two GCM datasets are unavailable for download. This results in a significant discrepancy between the number of GCMs and the selected GCMs compared with other studies, making it difficult to compare the results.
The limitation of choosing ERA5 as the observation precipitation
The six GCMs exhibit varying degrees of underestimation in the precipitation frequency at 6 mm (3h)-1 precipitation intensity than ERA5 in our study. Many researchers have found that ERA5 precipitation has a slight wet bias[46-48], and overestimation of precipitation amounts at specific locations and excessive snow depth in mountainous regions above 1,500 m[28]. Therefore, ERA5 precipitation chosen as the reference precipitation in this study may cause some bias compared to the research that chose the observation station precipitation data and remote sensing retrieval precipitation data as the reference precipitation[20,43,49].
The limitation in selecting precipitation indicators
We selected three indices, mean precipitation, hourly precipitation frequency, and hourly precipitation intensity, as Precipitation Characteristic Index to evaluate the ability of GCMs. Liu et al. (2023) selected six indices from the Expert Group on Climate Change Detection and Indicators (ETCCDI) to study the characteristics of extreme precipitation indices in the LMRB from 1980 to 2020, Annual total wet day precipitation, Number of heavy precipitation days, Number of very heavy precipitation days, Very wet days, MAX 5-day precipitation amount, and Simple daily intensity index[50]. The difference of precipitation indices will lead to diverse model evaluation performance. ETCCD provided 27 climate indices which have been widely used in the analysis and study of extreme climate events[51]. In the future, more precipitation indicators can be selected for analysis and evaluation.
CONCLUSION
In this paper, we evaluated the performance of precipitation simulation during the rainy season based on CMIP6 HighResMIP GCMs with high spatial resolution (50 km) and higher temporal resolution
(1) The spatial distribution of precipitation indices during the rainy season. For mean precipitation, the spatial pattern of the GCMs is similar to that of ERA5 with high mean precipitation in the lower LMRB and low mean precipitation in the upper LMRB, e.g., BCC-CSM2-HR, EC-Earth3P-HR, HadGEM3-GC31-HM, and MME-M. For hourly precipitation frequency, the spatial distribution of BCC-CSM2-HR and HadGEM3-GC31-HM are close to that of ERA5. The remaining four GCMs significantly deviate from the ERA5; EC-Earth3P-HR and MRI-AGCM3-2-S overestimate the hourly precipitation frequency over the entire LRMB; HiRAM-SIT-LR and NICAM16-8S underestimate the hourly precipitation frequency over the whole LMRB. The spatial pattern of MME-M is uniform and rarely captures the maximum hourly precipitation frequency. For hourly precipitation intensity, only two GCMs are capable of accurately simulating the spatial distribution: BCC-CSM2-HR and HadGEM3-GC31-HM. However, EC-Earth3P-HR and MRI-AGCM3-2-S have certain underestimation, and HiRAM-SIT-LR and NICAM16-8S have significant overestimation. The MME-M exhibits a slight overestimation of precipitation intensity in the lower LMRB. We found that two GCMs, BCC-CSM2-HR and HadGEM3-GC31-HM, demonstrate a strong ability to reproduce the spatial distribution of precipitation indices during the rainy season, performing better than other GCMs and MME-M.
(2) The latitudinal distribution of three climatological precipitation indices in the rainy season. The simulating maximum value of mean precipitation of GCMs and MME-M has similar characteristics to ERA5, which is also located at low latitudes of 14.75° N and lower altitudes below 500 m. However, HadGEM3-GC31-HM is much higher than the ERA5 and other GCMs, which is 5.67 mm d-1. The mean precipitation of GCMs decreases sharply above 20° N with increasing altitude. The trend of ERA5 and GCMs for hourly precipitation frequency is similar to that for mean precipitation. The hourly precipitation frequency of GCMs and MME-M is generally higher than that of ERA5, except for HiRAM-SIT-LR and NICAM16-8S. Some GCMs and MME-M can accurately simulate precipitation intensity, but the precipitation intensity of NICAM16-8S and HadGEM3-GC31-HM significantly deviates from that of the ERA5. However, MME-M slightly overestimates the intensity at lower altitudes below 20° N. We can conclude that most GCMs and MME-M are capable of accurately reproducing changes in the latitudinal distribution of mean precipitation, hourly precipitation frequency and hourly precipitation intensity, except HadGEM3-GC31-HM (mean precipitation), HiRAM-SIT-LR and NICAM16-8S (hourly precipitation frequency and hourly precipitation intensity).
(3) Precipitation frequency distribution under different grades of precipitation. We found that most GCMs overestimate the frequency of light precipitation, which can reach 60%-80%, while the frequency in the ERA5 is 53.9%. However, almost all GCMs underestimate the frequency of moderate precipitation and heavy precipitation. For torrential precipitation, severe torrential precipitation, and extremely torrential precipitation events, most GCMs overestimate their frequency.
(4) The performance of simulated precipitation during the rainy season. Most GCMs can not simulate hourly precipitation well, because the correlation coefficients of four GCMs are about 0.1 and their normalized standard deviations are greater than 1. However, EC-Earth3P-HR and MRI-AGCM3-2-S demonstrate relatively better performance, with correlation coefficients of 0.42 and 0.36, and the normalized standard deviations are close to REF.
We believe that with the improvement of numerical simulation at the high temporal and spatial resolution, the results can provide an important basis for water resource management in the LMRB.
DECLARATIONS
Acknowledgments
We would like to express our sincere gratitude for the two meteorological data (CMIP6 and ERA5) that were essential to this study. Our heartfelt thanks also go to the three reviewers for their valuable suggestions and to the editors for their meticulous work in refining the English language.
Authors’ contributions
Investigation: Yao D, Zhang M, Lang Y
Methodology, supervision: Yao D, Peng Y, Lang Y
Writing - original draft: Yao D, Zhang M
Writing - review and editing: Peng Y, Lang Y
Availability of data and materials
The ERA5 datasets used in this study are publicly available meteorological reanalysis data, which can be downloaded from the official website: https://cds.climate.copernicus.eu/ [Last accessed on 19 April 2023]. The CMIP6 HighResMIP Model datasets used in this study are publicly available for global climate modeling and forecasting and can be downloaded from the official websites: https://esgf-node.llnl.gov/search/cmip6/ [Last accessed on 27 September 2023]. Elevation data of STRM are available for download at https://www.gscloud.cn/ [Last accessed on 13 October 2023].
Financial support and sponsorship
This research was funded by the National Natural Science Foundation of China (grant number 41907147); Basic Research Project of Yunnan Province (grant number 202401AT070419); the Innovation and Entrepreneurship Training Fund for Undergraduate Students of Yunnan University (grant number 202205055); Scientific Research Project of Yunnan Meteorological Bureau (YZ202411).
Conflicts of interest
All authors declared that there are no conflicts of interest.
Ethical approval and consent to participate
Not applicable.
Consent for publication
Not applicable.
Copyright
© The Author(s) 2024.
REFERENCES
1. Gong X. The belt & road initiative and China’s influence in Southeast Asia. Pac Rev 2019;32:635-65.
2. Wu SN, Lei Y, Zhang WH, Cheng DS. Reviews of the national cooperation of flood disaster risk reduction in the Mekong River Basin. Sci Technol Rev 2020;38:80-7. (in Chinese).
3. Tan XS, Wang J, Tang XP, et al. Variation trends of climate change and hydrological responses of individual regions in Lancang-Mekong River Basin from 1960-2012. J Water Resour Water Eng 2020;31:1-8.
4. Wang S, Zhang L, She D, Wang G, Zhang Q. Future projections of flooding characteristics in the Lancang-Mekong River Basin under climate change. J Hydrol 2021;602:126778.
5. Kang H, Sridhar V, Ali SA. Climate change impacts on conventional and flash droughts in the Mekong River Basin. Sci Total Environ 2022;838:155845.
6. Chen XR, Wang XY, Baiyinbaoligao. Analysis of spatial and temporal distribution of flood losses in Mekong River Basin since 1962. J Catastrophol 2019;34:113-6. (in Chinese).
7. Zhou BT, Qian J. Changes of weather and climate extremes in the IPCC AR6. Clim Chang Res 2021;17:713-8. (in Chinese). Available from: https://www.researchgate.net/publication/372656309_Changes_of_weather_and_climate_extremes_in_the_IPCC_AR6 [Last accessed on 19 Nov 2024]
8. Lutz AF, Immerzeel WW, Shrestha AB, Bierkens MFP. Consistent increase in high Asia’s runoff due to increasing glacier melt and precipitation. Nat Clim Chang 2014;4:587-92.
9. Muetzelfeldt MR, Schiemann R, Turner AG, Klingaman NP, Vidale PL, Roberts MJ. Evaluation of Asian summer precipitation in different configurations of a high-resolution general circulation model in a range of decision-relevant spatial scales. Hydrol Earth Syst Sci 2021;25:6381-405.
10. Alexander LV, Arblaster JM. Historical and projected trends in temperature and precipitation extremes in Australia in observations and CMIP5. Weather Clim Extrem 2017;15:34-56.
11. Chen XC, Xu Y, Xu CH, Yao Y. Assessment of precipitation simulations in China by CMIP5 multi-models. Clim Chang Res 2014;10:217-25. (in Chinese).
12. Hu Y, Xu Y, Li J, Han Z. Evaluation on the performance of CMIP6 global climate models with difference horizontal resolution in simulating the precipitation over China. Clim Chang Res 2021;17:730-43. (in Chinese). Available from: https://www.researchgate.net/publication/373519285_CMIP6butongfenbianluquanqiuqihoumoshiduizhongguojiangshuimoninenglipinggu [Last accessed on 19 Nov 2024]
13. Ayugi B, Zhihong J, Zhu H, et al. Comparison of CMIP6 and CMIP5 models in simulating mean and extreme precipitation over East Africa. Intl J Climatol 2021;41:6474-96.
14. Gusain A, Ghosh S, Karmakar S. Added value of CMIP6 over CMIP5 models in simulating Indian summer monsoon rainfall. Atmos Res 2020;232:104680.
15. Bağçaci SÇ, Yucel I, Duzenli E, Yilmaz MT. Intercomparison of the expected change in the temperature and the precipitation retrieved from CMIP6 and CMIP5 climate projections: a mediterranean hot spot case, Turkey. Atmos Res 2021;256:105576.
16. Xin X, Wu T, Zhang J, Yao J, Fang Y. Comparison of CMIP6 and CMIP5 simulations of precipitation in China and the East Asian summer monsoon. Intl J Climatol 2020;40:6423-40.
17. Hariadi MH, van der Schrier G, Steeneveld G, et al. Evaluation of onset, cessation and seasonal precipitation of the Southeast Asia rainy season in CMIP5 regional climate models and HighResMIP global climate models. Intl J Climatol 2022;42:3007-24.
18. Liang J, Meng C, Wang J, Pan X, Pan Z. Projections of mean and extreme precipitation over China and their resolution dependence in the HighResMIP experiments. Atmos Res 2023;293:106932.
19. Yang X, Zhou B, Xu Y, Han Z. CMIP6 evaluation and projection of temperature and precipitation over China. Adv Atmos Sci 2021;38:817-30.
20. Xiao YJ, Li J, Li NN. Evaluation of CMIP6 HighResMIP models in simulating precipitation over Tibetan Plateau. Torrential Rain Dis 2022; 41(2):215-223. (in Chinese).
21. Huang Z, Wu X, Mao J. An evaluation for impacts of the horizontal resolution of CMIP6 models on simulating extreme summer rainfall over Southwest China. Plateau Meteorol 2021;40:1470-83. (in Chinese).
22. Try S, Tanaka S, Tanaka K, Sayama T, Khujanazarov T, Oeurng C. Comparison of CMIP5 and CMIP6 GCM performance for flood projections in the Mekong River Basin. J Hydrol 2022;40:101035.
23. Xin X, Wu T, Jie W, Zhang J. Impact of higher resolution on precipitation over China in CMIP6 HighResMIP models. Atmosphere 2021;12:762.
24. Dong W, Krasting JP, Guo H. Analysis of precipitation diurnal cycle and variance in multiple observations, CMIP6 models, and a series of GFDL-AM4.0 simulations. J Clim 2023;36:8637-55.
25. He DM. Analysis of hydrological characteristics in Lancang-Mekong river. Yunnan Geogr Environ Res 1995;1:58-74. (in Chinese). Available from: https://kns.cnki.net/kcms2/article/abstract?v=Oa1N_PzK0nRsDYvwhjSFvTJ_LR6lRBDAoC5UVSpy3WC0U7feWbjEjs6FThG4r6IIkbY0J53sNxdrZhhMZDahzt26wvZvkFp8QyX1DS3qklJyEKyj0qNzocrjM_lH4PyRh7aCURfjhZmn46nvtCZioLnkxlvZz-w9N-sTxFwtuHwJSPT4exCJTnIPpTW5oFbj4Au8IoI5kT8=&uniplatform=NZKPT [Last accessed on 27 Nov 2024]
26. Long D, Han ZY, Wang YM. Projection of future droughts across the Lancang-Mekong river under a changing environment. Adv Water Sci 2022;33:766-79. (in Chinese).
27. Li R, Huang H, Yu G, Yu H. Contributions of climatic variation and human activities to streamflow changes in the Lancang-Mekong River Basin. Resour Sci 2021;43:2428-41. (in Chinese).
28. Hersbach H, Bell B, Berrisford P, et al. The ERA5 global reanalysis. Quart J Royal Meteoro Soc 2020;146:1999-2049.
29. Haarsma RJ, Roberts MJ, Vidale PL, et al. High resolution model intercomparison project (HighResMIP v1.0) for CMIP6. Geosci Model Dev 2016;9:4185-208.
30. Wang L, Bao Q, He B. Short commentary on CMIP6 high resolution model intercompanson project (HighResMIP). Clim Chang Res 2019;15:498-502. (in Chinese). Available from: https://www.cnki.com.cn/Article/CJFDTotal-QHBH201905009.htm [Last accessed on 27 Nov 2024]
31. Yang KK, Guo DL, Hua W, Ma D, Xin YT. Evaluation and projection of CMIP6 HighResMIP in simulating surface air temperature and precipitation over Tibetan Plateau. Trans Atmos Sci 2023;46:193-204.
32. Wu T, Yu R, Lu Y, et al. BCC-CSM2-HR: a high-resolution version of the Beijing climate center climate system model. Geosci Model Dev 2021;14:2977-3006.
33. Haarsma R, Acosta M, Bakhshi R, et al. HighResMIP versions of EC-Earth: EC-Earth3P and EC-Earth3P-HR - description, model computational performance and basic validation. Geosci Model Dev 2020;13:3507-27.
34. Roberts MJ, Baker A, Blockley EW, et al. Description of the resolution hierarchy of the global coupled HadGEM3-GC3.1 model as used in CMIP6 HighResMIP experiments. Geosci Model Dev 2019;12:4999-5028.
35. Chen T, Zhang Y, Li N. Evaluation of CMIP6 HighResMIP models and ERA5 reanalysis in simulating summer precipitation over the Tibetan Plateau. Atmosphere 2023;14:1015.
36. Mizuta R, Yoshimura H, Murakami H, et al. Climate simulations using MRI-AGCM3.2 with 20-km grid. J Meteorol Soc Japan 2012;90A:233-58.
37. Kodama C, Ohno T, Seiki T, et al. The nonhydrostatic ICosahedral atmospheric model for CMIP6 HighResMIP simulations (NICAM16-S): experimental design, model description, and impacts of model updates. Geosci Model Dev 2021;14:795-820.
38. Abramowitz G, Herger N, Gutmann E, et al. ESD reviews: model dependence in multi-model climate ensembles: weighting, sub-selection and out-of-sample testing. Earth Syst Dynam 2019;10:91-105.
39. Merrifield AL, Brunner L, Lorenz R, Medhaug I, Knutti R. An investigation of weighting schemes suitable for incorporating large ensembles into multi-model ensembles. Earth Syst Dynam 2020;11:807-34.
40. Li J, Yu R, Yuan W, Chen H, Sun W, Zhang Y. Precipitation over East Asia simulated by NCAR CAM5 at different horizontal resolutions. J Adv Model Earth Syst 2015;7:774-90.
41. Taylor KE. Summarizing multiple aspects of model performance in a single diagram. J Geophys Res 2001;106:7183-92.
42. Sun Q, Miao C, Duan Q. Projected changes in temperature and precipitation in ten river basins over China in 21st century. Intl J Climatol 2015;35:1125-41.
43. Jin Z, Ge F, Chen Q, Lin Z. To what extent horizontal resolution improves the simulation of precipitation in CMIP6 HighResMIP models over Southwest China? Front Earth Sci 2023;10:1003748.
44. Chen Q, Ge F, Jin Z, Lin Z. How well do the CMIP6 HighResMIP models simulate precipitation over the Tibetan Plateau? Atmos Res 2022;279:106393.
45. Norris J, Hall A, Neelin JD, Thackeray CW, Chen D. Evaluation of the tail of the probability distribution of daily and Subdaily precipitation in CMIP6 models. J Clim 2021;34:2701-21.
46. Ou T, Chen D, Tang J, et al. Wet bias of summer precipitation in the northwestern Tibetan Plateau in ERA5 is linked to overestimated lower-level southerly wind over the plateau. Clim Dyn 2023;61:2139-53.
47. Lavers DA, Simmons A, Vamborg F, Rodwell MJ. An evaluation of ERA5 precipitation for climate monitoring. Quart J Royal Meteoro Soc 2022;148:3152-65.
48. Jiao D, Xu N, Yang F, Xu K. Evaluation of spatial-temporal variation performance of ERA5 precipitation data in China. Sci Rep 2021;11:17956.
49. Liang-Liang L, Jian L, Ru-Cong Y. Evaluation of CMIP6 HighResMIP models in simulating precipitation over central Asia. Adv Clim Chang Res 2022;13:1-13.
50. Liu J, Liu Y, Chen X, et al. Extreme precipitation events variation and projection in the Lancang-Mekong River Basin based on CMIP6 simulations. Atmosphere 2023;14:1350.
Cite This Article
How to Cite
Yao, D.; Zhang, M.; Peng, Y.; Lang, Y. Investigating the ability of CMIP6 HighResMIP models to simulate rainy season precipitation over the Lancang-Mekong River Basin. Dis. Prev. Res. 2024, 3, 10. http://dx.doi.org/10.20517/dpr.2024.02
Download Citation
Export Citation File:
Type of Import
Tips on Downloading Citation
Citation Manager File Format
Type of Import
Direct Import: When the Direct Import option is selected (the default state), a dialogue box will give you the option to Save or Open the downloaded citation data. Choosing Open will either launch your citation manager or give you a choice of applications with which to use the metadata. The Save option saves the file locally for later use.
Indirect Import: When the Indirect Import option is selected, the metadata is displayed and may be copied and pasted as needed.
About This Article
Copyright
Data & Comments
Data
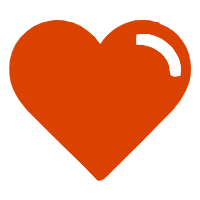
Comments
Comments must be written in English. Spam, offensive content, impersonation, and private information will not be permitted. If any comment is reported and identified as inappropriate content by OAE staff, the comment will be removed without notice. If you have any queries or need any help, please contact us at support@oaepublish.com.