Machine learning identifies efficient Ru-based electrocatalysts
Keywords
Water electrolysis for green hydrogen production enables the conversion of the renewable energy into clean fuel. Relative to the conventional alkaline water electrolyzers, proton exchange membrane (PEM) electrolyzers possess a more compact design and are capable of delivering a greater current density and higher voltage efficiency[1]. Regrettably, the sluggish kinetics of anodic oxygen evolution reaction (OER) and the degradation of catalysts in acidic and strongly oxidative environments largely hinder the upgrading of PEM[2]. Currently, Iridium oxides (IrO2), bearing an activity-stability compromise, are the primary catalysts for OER, yet the high cost and continuously growing demand urge us to find a feasible alternative[3]. In this context, Ru-based catalysts with the merits of approximately 100-fold greater abundance, 8-fold lower cost, and more appealing activity than IrO2 come into view[4]. Unfortunately, Ru-based catalysts, such as RuO2, tend to form soluble Ru>4+ species in the OER process, giving rise to a severe decay in catalytic performance. Notwithstanding the improvement in activity by some strategies, their lifespan remains limited to merely several hours at current densities higher than 10 mA·cm-2[5-7]. Challenges persist in the development of
Now, in their article published in Journal of the American Chemical Society, Abed et al. rapidly figured out the suitable composition of desirable Ru-based catalysts with the assistance of a ML computational pipeline [Figure 1A][9]. Initially, the trained pipeline identified the Ru-Cr-Ti-Ox as a good candidate with enhanced durability. And experimentally, the Ru0.6Cr0.2Ti0.2O2 was synthesized successfully, delivering a current density of 100 mA·cm-2 at the overpotential of 267 mV and tiny decay in a 200-hour stability test. Compared to
Figure 1. ML-aided framework identifies Ru-based acidic water oxidation catalysts. (A) Schematic of the design process of Ru-based catalysts from the ML-aided calculation model to the real catalyst; (B) The model named CGCNN-HD’s heatmaps of Pourbaix electrochemical stability (ΔGpbx) for the subset; (C) Mass activity at 1.48 V vs. RHE for Ru0.6Cr0.2Ti0.2Ox, Ru0.75Cr0.25Ox, and RuOx; (D) Stability test at a constant current density of 100 mA·cmgeo-2; (E) Accumulative total dissolved ions for RuOx and Ru0.6Cr0.2Ti0.2Ox; (F) O K-edge STXM spectroptychography XANES comparison between Ru0.75Cr0.25Ox and Ru0.6Cr0.2Ti0.2Ox; (G) DEMS signals of the reaction products using a H218O aqueous sulfuric acid electrolyte. This figure is quoted with permission from Abed et al.[9]. ML: Machine learning; CGCNN-HD: crystal graph convolutional neural network modified with a hyperbolic tangent activation function and dropout algorithm; RHE: reversible hydrogen electrode; STXM: scanning transmission X-ray microscopy; XANES: X-ray absorption near-edge structure; DEMS: differential electrochemical mass spectroscopy measurements.
To achieve this, firstly, a computational model employing a crystal graph convolutional neural network modified with a hyperbolic tangent activation function and dropout algorithm (CGCNN-HD) was trained, validated and tested using a data set of 36,465 metal oxide structures. The Pourbaix energy (ΔGpbx), referring to the energy of formation per atom, serves as a proxy to predict the electrochemical stability. All structures underwent a preprocessing and scaling procedure using the lattice scaling approach, with the predicted property shifted within a 95% confidence interval to minimize the errors caused by nonideal structures. With the trained model, a M-M2-O chemical space, which maintained the rutile oxide structure constant while substituting 46 elements, was explored to generate a dataset of 2,070 hypothetical compounds. A total of 195 rutile oxide structures were selected based on the energy above hull criteria [Figure 1B]. Focusing on the ΔGpbx of the Ru-containing structures, it was revealed that doping Ti, Sn, Ge and Cr improved the Pourbaix stability and resulted in the increase of the metal-oxygen covalency. Among these, Ti demonstrated the largest computational improvement and Cr exhibited enhancing activity in previous studies; thus, experimental validation was concentrated on the Ru-Cr-Ti-Ox family of catalysts.
A series of Ru-Cr-Ti-Ox materials preserving the rutile oxide structure were synthesized by a sol-gel method starting by tuning the amount of Cr in the Ru-Cr-O2 and then optimizing the Ti proportion. The
In summary, Abed et al. presented an inspiring approach for exploiting multi-component catalysts[9]. The guidance of ML enables the devising of efficient and durable catalysts to become more targeted, reducing the workload and time-consuming processes largely. Notably, the efficacy of ML models depends largely on the quantity and quality of training data. Despite the exponential growth in published experimental electrocatalytic data annually, a limited amount is currently accessible for ML training. Closer collaboration between experiments and ML simulations is needed. Besides, the selected descriptors require careful consideration, and the applicability of the ΔGpbx discussed in this work to other systems deserves to be verified. Although there remains considerable exploration ahead, the prospect for ML-assisted development of efficient catalysts is undoubtedly attractive.
DECLARATIONS
Authors’ contributions
Made substantial contributions to conception and writing of the highlight: Zhang M, Wan Y, Lin Y
Availability of data and materials
Not applicable.
Financial support and sponsorship
This work was supported by the Natural Science Foundation of Xiamen City, China (3502Z20227256), National Natural Science Foundation of China (22202205, 22209170), XMIREM Autonomously Deployment Project, China (2023CX14, 2023GG01), Major Science and Technology Program of Xiamen City, China (3502Z20231054), National Natural Science Foundation of Fujian Province, China (2022J01502), and STS Program of the Chinese Academy of Sciences, China (2023T3071).
Conflicts of interest
All authors declared that there are no conflicts of interest.
Ethical approval and consent to participate
Not applicable.
Consent for publication
Not applicable.
Copyright
© The Author(s) 2024.
REFERENCES
1. Li L, Wang P, Shao Q, Huang X. Recent progress in advanced electrocatalyst design for acidic oxygen evolution reaction. Adv Mater 2021;33:e2004243.
2. Xu X, Sun H, Jiang SP, Shao Z. Modulating metal-organic frameworks for catalyzing acidic oxygen evolution for proton exchange membrane water electrolysis. SusMat 2021;1:460-81.
3. Wu ZY, Chen FY, Li B, et al. Non-iridium-based electrocatalyst for durable acidic oxygen evolution reaction in proton exchange membrane water electrolysis. Nat Mater 2023;22:100-8.
4. Chen D, Yu R, Yu K, et al. Bicontinuous RuO2 nanoreactors for acidic water oxidation. Nat Commun 2024;15:3928.
5. Hao S, Liu M, Pan J, et al. Dopants fixation of Ruthenium for boosting acidic oxygen evolution stability and activity. Nat Commun 2020;11:5368.
6. Lin Y, Tian Z, Zhang L, et al. Chromium-ruthenium oxide solid solution electrocatalyst for highly efficient oxygen evolution reaction in acidic media. Nat Commun 2019;10:162.
7. Yao Y, Hu S, Chen W, et al. Engineering the electronic structure of single atom Ru sites via compressive strain boosts acidic water oxidation electrocatalysis. Nat Catal 2019;2:304-13.
8. Hu Z, Yan Q, Wang Y. Dynamic surface reconstruction of perovskite oxides in oxygen evolution reaction and its impacts on catalysis: a critical review. Mater Today Chem 2023;34:101800.
Cite This Article
How to Cite
Zhang, M.; Wan Y.; Lin Y. Machine learning identifies efficient Ru-based electrocatalysts. Chem. Synth. 2024, 4, 55. http://dx.doi.org/10.20517/cs.2024.74
Download Citation
Export Citation File:
Type of Import
Tips on Downloading Citation
Citation Manager File Format
Type of Import
Direct Import: When the Direct Import option is selected (the default state), a dialogue box will give you the option to Save or Open the downloaded citation data. Choosing Open will either launch your citation manager or give you a choice of applications with which to use the metadata. The Save option saves the file locally for later use.
Indirect Import: When the Indirect Import option is selected, the metadata is displayed and may be copied and pasted as needed.
About This Article
Copyright
Data & Comments
Data
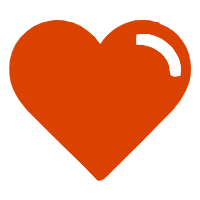
Comments
Comments must be written in English. Spam, offensive content, impersonation, and private information will not be permitted. If any comment is reported and identified as inappropriate content by OAE staff, the comment will be removed without notice. If you have any queries or need any help, please contact us at support@oaepublish.com.